class: center, middle, inverse, title-slide # SOC 301 Slides ## Pacific University ### Chester Ismay ### Spring 2017 --- layout: true .footer[[Week 14 - Tuesday](https://ismayc.github.io/soc301_s2017/2017/05/02/week-14---tuesday/)] --- name: week14t class: center, middle # Week 14 - Tuesday # May 2, 2017 --- class: center, middle <img src="figure/toldyouso.png" width="100%" /> --- ## Warmup ### Provide the definitions of - <u>confidence interval</u> and - <u>standard error</u> ### as given in class and in the textbook. --- class: center, middle # Work through R problem from PS13 -- - Discussion [here](https://ismayc.github.io/soc301_s2017/problem-sets/ps-key/index.html#ps13-key) --- ## Relating hypothesis testing and confidence intervals - Worksheet [here](http://ismayc.github.io/soc301_s2017/ci-ht.pdf) --- layout: true .footer[[Week 13 - Thursday](https://ismayc.github.io/soc301_s2017/2017/04/27/week-13---thursday/)] --- name: week13th class: center, middle # Week 13 - Thursday # April 27, 2017 --- # Chatting about *p*-values and *p*-hacking - Discuss the big ideas from the 538 articles you have read with ONE other person you haven't paired with before in this class - [You Can’t Trust What You Read About Nutrition](http://fivethirtyeight.com/features/you-cant-trust-what-you-read-about-nutrition/) - [Statisticians Found One Thing They Can Agree On: It’s Time To Stop Misusing P-Values](http://fivethirtyeight.com/features/statisticians-found-one-thing-they-can-agree-on-its-time-to-stop-misusing-p-values/) - [Science Isn’t Broken](https://fivethirtyeight.com/features/science-isnt-broken/) - How do these articles relate to the content covered in class/ModernDive? --- class: center, middle # Turn in your paragraphs from the nutrition article for PS12 --- name: haven ## Global Social Data ### Includes data from including the CIA World Factbook, the World Bank, the Association of Religion Data Archives, the United Nations Office on Drugs and Crime, the International Centre for Prison Studies, and the Stockholm International Peace Research Institute. - Let's imagine this data represents our population - We want to identify the mean percentage of women holding national office. ```r install.packages("haven") library(haven); library(dplyr) url <- "https://ismayc.github.io/global13sdss_0.sav" global13_full <- read_sav(url) global13 <- global13_full %>% select(country, FEMALEOFFICE) ``` --- ## Plotting the population data ```r library(ggplot2) ggplot(data = global13, mapping = aes(x = FEMALEOFFICE)) + geom_histogram(color = "white", bins = 15) ``` 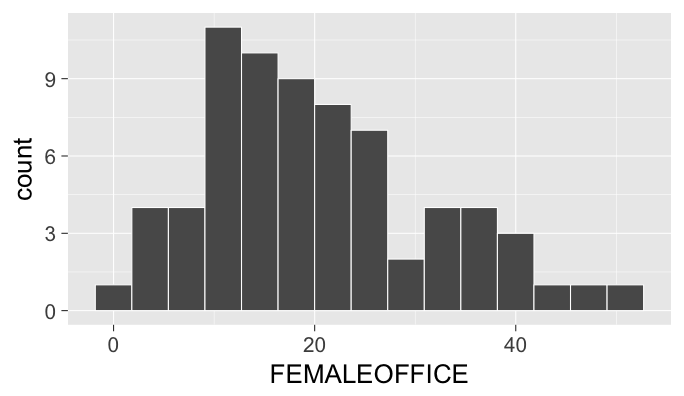<!-- --> --- ## Sampling - Let's use **sampling** to produce an estimate of the population mean - Let's choose a sample of size 25 ```r library(mosaic); set.seed(2017) sample1 <- global13 %>% resample(size = 25, replace = FALSE) (mean_s1_f <- sample1 %>% summarize(mean_f = mean(FEMALEOFFICE))) ``` ``` # A tibble: 1 × 1 mean_f <dbl> 1 19.576 ``` -- - But this is just one sample? We could produce many samples with different means. Which one is correct? --- ## Sampling distribution ```r sample_means <- do(5000) * global13 %>% resample(size = 25, replace = FALSE) %>% summarize(mean_f = mean(FEMALEOFFICE)) sample_means ``` ``` mean_f 1 21.028 2 20.388 3 21.160 4 14.924 5 20.812 6 17.752 7 21.860 8 19.352 9 21.124 10 19.284 11 18.720 12 20.940 13 18.800 14 19.516 15 18.100 16 18.896 17 22.044 18 21.140 19 21.804 20 20.376 21 20.204 22 22.836 23 23.432 24 24.860 25 19.340 26 23.988 27 19.628 28 23.044 29 21.900 30 22.956 31 22.432 32 21.692 33 22.532 34 21.280 35 21.864 36 19.820 37 21.240 38 19.580 39 22.536 40 17.652 41 20.852 42 20.544 43 17.772 44 20.036 45 20.036 46 19.768 47 21.592 48 19.340 49 20.748 50 20.044 51 21.212 52 20.588 53 18.388 54 21.808 55 21.480 56 20.004 57 19.464 58 22.236 59 19.556 60 18.216 61 20.200 62 22.212 63 20.516 64 20.096 65 21.504 66 19.656 67 21.032 68 22.692 69 20.836 70 21.896 71 21.292 72 21.500 73 21.688 74 21.872 75 23.608 76 24.100 77 24.252 78 22.424 79 22.088 80 21.740 81 19.828 82 20.968 83 18.724 84 18.260 85 16.644 86 18.748 87 21.876 88 20.220 89 22.044 90 19.300 91 21.008 92 19.388 93 20.796 94 21.556 95 20.120 96 20.004 97 23.572 98 18.688 99 18.596 100 18.300 101 22.688 102 24.868 103 22.248 104 21.688 105 20.260 106 18.340 107 18.460 108 20.988 109 20.524 110 19.656 111 22.992 112 20.404 113 16.520 114 18.744 115 24.052 116 21.928 117 20.352 118 19.528 119 19.984 120 19.280 121 22.048 122 19.104 123 20.400 124 18.332 125 22.444 126 16.420 127 17.868 128 19.112 129 23.244 130 22.892 131 17.076 132 23.672 133 21.300 134 19.228 135 25.100 136 22.584 137 17.460 138 20.116 139 20.872 140 17.676 141 22.488 142 19.116 143 19.028 144 20.912 145 19.404 146 17.032 147 22.060 148 23.028 149 22.564 150 17.052 151 22.328 152 21.976 153 20.556 154 16.044 155 18.460 156 18.624 157 19.120 158 18.988 159 21.172 160 20.912 161 20.280 162 20.292 163 19.672 164 17.836 165 20.668 166 20.424 167 23.704 168 22.844 169 21.660 170 21.780 171 21.292 172 23.104 173 20.924 174 16.312 175 23.036 176 21.408 177 16.716 178 21.920 179 23.408 180 22.928 181 19.040 182 18.488 183 20.000 184 21.104 185 17.408 186 20.212 187 20.308 188 21.160 189 20.928 190 22.176 191 22.232 192 20.792 193 24.136 194 20.504 195 22.744 196 24.068 197 19.220 198 21.868 199 16.912 200 20.532 201 22.112 202 19.356 203 18.244 204 19.960 205 19.140 206 18.224 207 21.376 208 19.372 209 17.680 210 20.484 211 18.588 212 19.252 213 20.816 214 18.516 215 18.996 216 18.376 217 19.696 218 20.084 219 17.416 220 21.052 221 19.888 222 19.496 223 18.956 224 21.552 225 16.384 226 21.048 227 22.096 228 19.660 229 22.972 230 22.056 231 19.592 232 22.840 233 17.856 234 20.236 235 21.776 236 20.700 237 20.168 238 22.580 239 18.896 240 18.932 241 20.084 242 20.528 243 18.132 244 18.288 245 22.508 246 21.516 247 22.296 248 19.056 249 22.880 250 19.036 251 21.700 252 21.304 253 19.020 254 19.332 255 22.172 256 21.932 257 22.016 258 21.324 259 22.636 260 20.544 261 22.080 262 20.280 263 21.864 264 21.116 265 19.792 266 20.988 267 20.336 268 21.120 269 22.912 270 17.672 271 20.196 272 19.588 273 21.476 274 18.908 275 20.228 276 18.784 277 18.276 278 18.808 279 21.788 280 21.144 281 21.864 282 16.936 283 22.344 284 24.976 285 19.536 286 23.040 287 24.088 288 18.804 289 21.016 290 20.184 291 22.552 292 22.368 293 20.976 294 21.388 295 19.244 296 21.360 297 22.852 298 18.076 299 19.616 300 22.496 301 22.348 302 20.420 303 22.320 304 17.344 305 18.324 306 19.008 307 21.756 308 24.532 309 19.244 310 21.484 311 19.920 312 19.644 313 19.704 314 18.368 315 24.200 316 21.300 317 19.180 318 19.248 319 20.908 320 20.688 321 16.576 322 20.740 323 21.516 324 20.140 325 19.208 326 20.432 327 23.512 328 21.772 329 21.588 330 20.024 331 22.236 332 20.892 333 21.584 334 22.460 335 20.300 336 20.056 337 18.828 338 19.788 339 21.724 340 22.208 341 23.112 342 16.620 343 22.384 344 19.028 345 21.968 346 22.352 347 19.496 348 19.976 349 21.652 350 17.420 351 17.844 352 21.944 353 19.032 354 20.876 355 20.944 356 21.068 357 21.748 358 23.036 359 20.752 360 20.748 361 16.736 362 19.620 363 18.184 364 22.608 365 20.560 366 22.064 367 20.384 368 18.084 369 20.204 370 22.160 371 22.140 372 23.764 373 19.188 374 18.084 375 22.108 376 18.148 377 18.160 378 20.044 379 19.592 380 23.672 381 20.516 382 22.108 383 22.976 384 19.052 385 22.432 386 20.440 387 20.460 388 22.376 389 20.404 390 18.064 391 17.220 392 20.928 393 21.568 394 21.400 395 21.444 396 19.820 397 20.780 398 21.548 399 18.152 400 16.200 401 21.908 402 20.824 403 21.124 404 16.904 405 19.740 406 21.820 407 19.880 408 21.868 409 18.528 410 19.316 411 22.412 412 22.600 413 21.020 414 20.548 415 20.164 416 21.816 417 18.380 418 21.356 419 20.752 420 18.244 421 21.456 422 21.148 423 17.028 424 19.444 425 17.164 426 21.304 427 22.964 428 18.780 429 21.340 430 20.064 431 21.004 432 23.876 433 21.832 434 16.992 435 20.428 436 17.864 437 21.612 438 17.732 439 18.444 440 18.828 441 20.356 442 20.100 443 20.148 444 21.376 445 20.208 446 19.296 447 18.308 448 22.528 449 22.900 450 20.944 451 19.176 452 18.728 453 19.296 454 20.456 455 21.088 456 21.720 457 16.616 458 17.672 459 20.872 460 18.204 461 20.320 462 22.992 463 21.452 464 21.024 465 18.376 466 17.700 467 23.056 468 19.912 469 18.700 470 19.884 471 21.124 472 21.636 473 23.624 474 17.900 475 20.832 476 22.708 477 20.484 478 18.616 479 19.088 480 21.484 481 21.908 482 20.408 483 19.876 484 19.348 485 21.036 486 22.608 487 20.316 488 19.036 489 23.016 490 21.344 491 19.380 492 18.676 493 20.824 494 17.732 495 19.948 496 22.900 497 22.360 498 24.544 499 21.048 500 16.220 501 19.924 502 19.188 503 20.520 504 21.624 505 19.300 506 23.644 507 21.772 508 18.108 509 23.228 510 19.500 511 22.176 512 20.456 513 20.132 514 21.620 515 21.656 516 21.296 517 19.104 518 19.576 519 21.928 520 21.592 521 22.120 522 21.748 523 19.440 524 17.228 525 19.916 526 22.632 527 19.236 528 20.320 529 20.568 530 20.352 531 19.460 532 20.092 533 20.808 534 22.952 535 22.924 536 21.020 537 17.240 538 20.632 539 20.516 540 23.680 541 19.144 542 20.160 543 19.988 544 18.248 545 18.604 546 19.500 547 18.440 548 23.144 549 23.564 550 20.944 551 18.696 552 19.456 553 20.292 554 21.044 555 20.852 556 21.228 557 15.416 558 18.164 559 24.676 560 18.200 561 21.212 562 19.484 563 19.176 564 18.732 565 19.524 566 20.464 567 19.072 568 20.468 569 22.016 570 19.088 571 20.544 572 19.136 573 20.228 574 20.868 575 23.564 576 21.492 577 20.948 578 22.816 579 17.936 580 21.180 581 21.612 582 17.932 583 18.544 584 19.264 585 24.608 586 20.596 587 15.456 588 17.916 589 18.716 590 21.356 591 22.004 592 22.508 593 21.256 594 18.316 595 19.420 596 19.664 597 20.656 598 21.968 599 18.936 600 20.820 601 21.668 602 18.704 603 18.796 604 22.624 605 18.256 606 23.044 607 19.084 608 23.192 609 23.104 610 19.060 611 23.744 612 19.056 613 20.512 614 19.872 615 20.288 616 18.688 617 22.152 618 19.756 619 21.960 620 20.476 621 18.592 622 19.056 623 19.304 624 23.024 625 20.620 626 22.004 627 20.972 628 22.504 629 18.636 630 18.988 631 21.100 632 19.300 633 20.844 634 23.212 635 20.672 636 19.480 637 20.044 638 22.644 639 20.976 640 20.132 641 16.036 642 24.072 643 20.004 644 22.264 645 21.176 646 21.824 647 17.116 648 22.968 649 20.544 650 22.768 651 21.088 652 20.160 653 21.936 654 19.256 655 16.952 656 20.656 657 18.924 658 18.640 659 22.716 660 23.204 661 21.000 662 21.112 663 19.884 664 16.584 665 21.336 666 20.096 667 20.816 668 20.128 669 24.392 670 21.056 671 16.848 672 17.708 673 17.908 674 20.548 675 20.384 676 22.336 677 19.876 678 22.356 679 20.540 680 21.388 681 18.048 682 23.112 683 19.276 684 19.220 685 20.876 686 21.376 687 24.608 688 21.268 689 22.288 690 18.884 691 18.972 692 22.628 693 20.424 694 18.704 695 22.136 696 22.840 697 18.500 698 16.696 699 21.604 700 21.972 701 20.092 702 21.432 703 20.664 704 23.800 705 23.212 706 20.160 707 21.988 708 22.288 709 19.672 710 19.948 711 21.764 712 21.012 713 22.952 714 23.108 715 19.868 716 17.188 717 19.924 718 20.432 719 21.632 720 19.268 721 20.092 722 17.928 723 20.416 724 18.312 725 21.560 726 20.204 727 22.268 728 23.328 729 21.652 730 20.624 731 21.144 732 20.672 733 21.556 734 19.272 735 22.540 736 18.076 737 17.980 738 19.856 739 22.468 740 18.060 741 19.216 742 16.748 743 17.540 744 19.512 745 19.584 746 19.624 747 22.164 748 18.468 749 16.280 750 20.040 751 19.804 752 21.060 753 21.748 754 21.828 755 20.692 756 19.412 757 19.408 758 20.968 759 20.936 760 20.492 761 20.016 762 20.516 763 19.596 764 19.964 765 21.472 766 17.968 767 22.916 768 20.676 769 21.092 770 21.836 771 22.712 772 19.416 773 24.052 774 18.204 775 23.120 776 18.636 777 19.136 778 22.944 779 18.556 780 20.556 781 21.320 782 16.076 783 17.904 784 17.608 785 20.872 786 20.552 787 20.508 788 20.064 789 18.252 790 20.536 791 18.684 792 18.216 793 17.632 794 19.956 795 18.032 796 19.656 797 19.448 798 20.536 799 18.776 800 17.516 801 21.704 802 17.732 803 21.572 804 19.500 805 22.432 806 18.708 807 22.272 808 18.600 809 20.480 810 18.408 811 23.160 812 22.548 813 21.444 814 18.324 815 19.292 816 22.624 817 19.556 818 20.944 819 18.504 820 19.168 821 19.288 822 18.068 823 19.212 824 19.584 825 21.304 826 19.652 827 20.172 828 25.196 829 16.976 830 21.380 831 21.300 832 16.224 833 19.024 834 20.868 835 18.612 836 19.920 837 19.352 838 19.984 839 20.632 840 23.184 841 18.952 842 24.208 843 21.292 844 17.220 845 19.388 846 24.272 847 21.080 848 20.520 849 22.800 850 20.088 851 21.636 852 22.180 853 19.580 854 18.008 855 24.592 856 22.280 857 19.164 858 24.616 859 21.236 860 19.468 861 20.920 862 18.076 863 18.292 864 21.692 865 19.704 866 21.024 867 19.644 868 19.568 869 21.168 870 18.416 871 22.720 872 19.512 873 19.812 874 17.544 875 19.132 876 20.188 877 16.708 878 18.396 879 19.036 880 19.104 881 21.212 882 22.888 883 21.192 884 20.912 885 18.444 886 18.120 887 20.332 888 16.588 889 18.260 890 17.836 891 21.392 892 21.624 893 19.532 894 22.684 895 19.984 896 17.712 897 19.464 898 24.564 899 19.672 900 22.680 901 18.948 902 21.564 903 17.464 904 18.408 905 19.492 906 18.464 907 22.256 908 21.156 909 24.496 910 21.492 911 22.968 912 22.752 913 16.944 914 20.200 915 19.128 916 25.024 917 20.992 918 20.396 919 18.172 920 22.840 921 20.812 922 24.024 923 17.864 924 19.440 925 18.448 926 20.340 927 23.168 928 24.584 929 20.308 930 22.316 931 18.628 932 21.468 933 22.832 934 17.020 935 18.620 936 22.880 937 16.416 938 22.048 939 19.288 940 22.968 941 21.940 942 21.560 943 21.844 944 21.408 945 22.088 946 17.988 947 19.928 948 21.640 949 24.400 950 19.132 951 20.160 952 20.112 953 19.784 954 19.836 955 17.288 956 18.688 957 22.036 958 20.488 959 20.280 960 16.772 961 17.820 962 20.596 963 20.316 964 20.588 965 19.788 966 21.332 967 20.092 968 19.900 969 19.856 970 22.044 971 20.088 972 19.976 973 20.056 974 18.620 975 22.056 976 18.956 977 18.488 978 23.140 979 22.108 980 25.664 981 20.228 982 20.116 983 19.548 984 22.196 985 18.580 986 20.380 987 19.756 988 22.180 989 21.104 990 19.300 991 19.336 992 22.216 993 20.004 994 20.556 995 21.944 996 17.548 997 25.456 998 19.800 999 20.812 1000 21.648 1001 15.952 1002 26.032 1003 21.740 1004 22.208 1005 17.968 1006 19.496 1007 19.240 1008 19.156 1009 18.024 1010 21.588 1011 19.292 1012 23.252 1013 21.596 1014 23.008 1015 18.828 1016 18.792 1017 20.492 1018 24.136 1019 22.692 1020 21.640 1021 22.892 1022 20.208 1023 17.184 1024 21.312 1025 19.432 1026 19.088 1027 21.236 1028 21.948 1029 22.072 1030 19.104 1031 20.268 1032 20.500 1033 22.412 1034 18.692 1035 20.008 1036 21.688 1037 20.832 1038 20.008 1039 18.568 1040 20.680 1041 17.480 1042 17.908 1043 20.128 1044 22.356 1045 19.224 1046 20.908 1047 17.620 1048 18.916 1049 22.436 1050 16.436 1051 23.496 1052 21.268 1053 21.932 1054 18.636 1055 20.260 1056 19.920 1057 21.496 1058 17.760 1059 19.660 1060 19.528 1061 21.180 1062 17.268 1063 20.072 1064 22.236 1065 17.920 1066 23.624 1067 21.996 1068 22.284 1069 21.124 1070 20.636 1071 19.228 1072 23.616 1073 17.316 1074 20.488 1075 20.432 1076 17.160 1077 19.532 1078 20.168 1079 19.828 1080 25.236 1081 18.604 1082 21.292 1083 24.924 1084 21.716 1085 25.768 1086 22.664 1087 20.000 1088 19.140 1089 21.468 1090 19.012 1091 21.540 1092 22.680 1093 17.968 1094 19.196 1095 19.332 1096 17.500 1097 19.900 1098 19.692 1099 19.380 1100 19.276 1101 19.256 1102 20.660 1103 19.860 1104 17.756 1105 17.912 1106 20.900 1107 16.424 1108 21.244 1109 19.960 1110 21.668 1111 21.440 1112 18.672 1113 20.764 1114 20.076 1115 18.308 1116 22.884 1117 22.684 1118 21.940 1119 19.772 1120 23.720 1121 20.896 1122 20.156 1123 22.036 1124 17.704 1125 22.008 1126 16.128 1127 21.484 1128 18.956 1129 21.444 1130 21.136 1131 22.780 1132 19.024 1133 17.560 1134 23.408 1135 21.684 1136 20.496 1137 21.284 1138 20.632 1139 19.268 1140 19.004 1141 18.720 1142 19.660 1143 21.604 1144 19.724 1145 20.956 1146 16.964 1147 21.668 1148 22.988 1149 21.232 1150 21.944 1151 20.992 1152 19.644 1153 19.228 1154 17.712 1155 20.812 1156 18.476 1157 17.160 1158 20.000 1159 17.968 1160 21.324 1161 20.316 1162 18.700 1163 21.076 1164 21.180 1165 18.016 1166 22.600 1167 23.984 1168 21.992 1169 23.584 1170 20.832 1171 23.144 1172 22.576 1173 18.696 1174 19.808 1175 16.816 1176 17.764 1177 19.784 1178 19.200 1179 20.756 1180 18.748 1181 15.392 1182 20.116 1183 18.656 1184 19.380 1185 20.932 1186 18.972 1187 19.788 1188 18.944 1189 20.968 1190 21.700 1191 20.936 1192 18.324 1193 21.196 1194 19.668 1195 22.648 1196 21.160 1197 17.132 1198 20.700 1199 18.624 1200 21.852 1201 18.456 1202 18.548 1203 19.744 1204 18.492 1205 22.316 1206 19.324 1207 22.384 1208 21.160 1209 19.240 1210 22.944 1211 20.340 1212 22.976 1213 18.848 1214 19.100 1215 19.332 1216 20.840 1217 18.260 1218 20.980 1219 19.288 1220 18.792 1221 21.404 1222 22.404 1223 20.444 1224 26.224 1225 19.216 1226 21.036 1227 20.072 1228 18.632 1229 17.112 1230 17.656 1231 20.904 1232 19.148 1233 21.040 1234 19.552 1235 21.476 1236 18.052 1237 20.792 1238 21.004 1239 20.820 1240 19.232 1241 18.564 1242 18.604 1243 21.840 1244 20.788 1245 20.636 1246 19.016 1247 21.028 1248 21.848 1249 19.728 1250 22.592 1251 22.008 1252 21.340 1253 18.920 1254 19.056 1255 23.748 1256 18.280 1257 20.104 1258 22.784 1259 18.620 1260 21.776 1261 25.252 1262 21.044 1263 22.928 1264 18.800 1265 20.484 1266 19.896 1267 20.212 1268 19.500 1269 22.112 1270 21.476 1271 21.524 1272 19.216 1273 17.624 1274 20.136 1275 18.184 1276 20.160 1277 18.296 1278 21.920 1279 18.708 1280 18.820 1281 20.036 1282 20.680 1283 19.612 1284 19.820 1285 19.924 1286 22.568 1287 18.688 1288 21.460 1289 21.708 1290 19.696 1291 22.204 1292 20.652 1293 20.928 1294 17.580 1295 21.752 1296 17.160 1297 19.312 1298 20.092 1299 20.128 1300 19.592 1301 17.604 1302 22.568 1303 22.132 1304 20.540 1305 17.988 1306 15.536 1307 21.252 1308 20.208 1309 21.464 1310 21.428 1311 21.260 1312 20.960 1313 17.412 1314 21.104 1315 19.148 1316 19.280 1317 23.328 1318 20.412 1319 18.804 1320 21.236 1321 17.728 1322 21.888 1323 21.028 1324 22.456 1325 22.692 1326 19.360 1327 23.092 1328 20.996 1329 21.088 1330 22.012 1331 20.680 1332 21.896 1333 20.656 1334 18.212 1335 20.928 1336 22.508 1337 22.492 1338 21.020 1339 21.552 1340 20.548 1341 20.612 1342 17.136 1343 17.732 1344 23.808 1345 21.100 1346 17.020 1347 18.940 1348 18.792 1349 20.716 1350 22.260 1351 17.736 1352 18.880 1353 23.792 1354 21.264 1355 19.580 1356 20.868 1357 19.948 1358 23.320 1359 19.444 1360 24.336 1361 20.100 1362 20.096 1363 20.632 1364 25.792 1365 19.948 1366 20.300 1367 20.176 1368 20.796 1369 22.428 1370 18.332 1371 21.128 1372 19.784 1373 18.680 1374 17.784 1375 20.488 1376 15.280 1377 22.544 1378 18.468 1379 20.980 1380 21.500 1381 21.508 1382 19.344 1383 23.028 1384 19.984 1385 21.260 1386 21.008 1387 20.780 1388 22.908 1389 21.312 1390 21.564 1391 20.188 1392 20.932 1393 20.576 1394 21.412 1395 22.260 1396 19.780 1397 19.392 1398 22.576 1399 22.316 1400 18.248 1401 19.952 1402 21.508 1403 19.132 1404 18.428 1405 21.560 1406 17.656 1407 20.896 1408 21.944 1409 16.180 1410 21.404 1411 19.816 1412 17.284 1413 19.148 1414 19.744 1415 18.608 1416 17.420 1417 22.284 1418 21.116 1419 23.072 1420 19.416 1421 18.092 1422 18.616 1423 22.456 1424 22.432 1425 24.452 1426 21.440 1427 20.124 1428 18.192 1429 21.080 1430 24.072 1431 18.140 1432 20.192 1433 22.992 1434 16.664 1435 20.244 1436 21.908 1437 18.364 1438 22.288 1439 20.880 1440 19.048 1441 20.340 1442 24.860 1443 17.384 1444 16.844 1445 24.208 1446 20.448 1447 22.452 1448 17.716 1449 20.948 1450 18.156 1451 22.428 1452 19.516 1453 21.776 1454 21.264 1455 17.752 1456 20.740 1457 19.320 1458 19.928 1459 22.212 1460 22.900 1461 17.764 1462 18.988 1463 17.692 1464 18.372 1465 19.696 1466 19.536 1467 23.064 1468 20.408 1469 22.204 1470 18.500 1471 18.824 1472 21.500 1473 20.468 1474 20.580 1475 19.144 1476 19.576 1477 21.208 1478 17.800 1479 17.844 1480 20.152 1481 19.324 1482 21.368 1483 23.136 1484 18.368 1485 20.060 1486 22.564 1487 17.716 1488 20.092 1489 25.756 1490 19.988 1491 21.788 1492 21.432 1493 19.372 1494 19.184 1495 19.832 1496 23.564 1497 23.256 1498 21.496 1499 21.332 1500 20.388 1501 19.744 1502 21.096 1503 19.988 1504 20.140 1505 19.920 1506 19.828 1507 20.680 1508 24.252 1509 16.488 1510 20.108 1511 14.976 1512 22.988 1513 21.196 1514 21.980 1515 19.284 1516 22.020 1517 21.968 1518 22.056 1519 20.220 1520 23.904 1521 18.716 1522 21.468 1523 19.608 1524 19.372 1525 17.408 1526 20.108 1527 19.448 1528 23.712 1529 22.568 1530 20.288 1531 20.252 1532 22.956 1533 18.912 1534 19.176 1535 22.820 1536 22.176 1537 20.212 1538 19.748 1539 20.392 1540 21.676 1541 19.416 1542 19.932 1543 20.372 1544 19.196 1545 17.940 1546 23.560 1547 21.288 1548 20.428 1549 18.300 1550 23.612 1551 21.288 1552 21.624 1553 17.952 1554 17.864 1555 19.628 1556 21.380 1557 22.464 1558 17.944 1559 20.368 1560 21.632 1561 20.408 1562 21.556 1563 18.244 1564 18.096 1565 19.636 1566 23.176 1567 20.748 1568 24.064 1569 19.872 1570 18.176 1571 16.748 1572 24.000 1573 20.400 1574 22.284 1575 23.104 1576 19.416 1577 21.424 1578 22.120 1579 22.384 1580 17.488 1581 22.248 1582 23.240 1583 19.772 1584 20.064 1585 17.148 1586 19.376 1587 19.508 1588 20.824 1589 17.176 1590 18.288 1591 23.784 1592 22.936 1593 16.904 1594 18.656 1595 20.476 1596 22.928 1597 18.676 1598 20.904 1599 19.812 1600 19.208 1601 20.576 1602 20.800 1603 15.636 1604 19.680 1605 22.052 1606 20.800 1607 22.460 1608 24.192 1609 19.356 1610 19.768 1611 19.020 1612 21.688 1613 24.488 1614 21.252 1615 20.360 1616 19.908 1617 20.692 1618 20.296 1619 20.520 1620 21.292 1621 20.132 1622 21.944 1623 19.500 1624 18.436 1625 21.396 1626 18.428 1627 20.972 1628 21.500 1629 20.216 1630 21.236 1631 17.972 1632 22.680 1633 19.160 1634 19.500 1635 20.320 1636 20.364 1637 19.060 1638 14.956 1639 21.168 1640 22.248 1641 19.784 1642 17.236 1643 23.688 1644 22.384 1645 20.816 1646 22.088 1647 19.580 1648 21.556 1649 22.524 1650 21.212 1651 19.328 1652 19.668 1653 24.568 1654 19.784 1655 19.980 1656 23.868 1657 20.384 1658 23.212 1659 24.368 1660 22.704 1661 21.292 1662 21.996 1663 24.584 1664 18.920 1665 18.184 1666 20.604 1667 20.536 1668 22.448 1669 24.296 1670 20.172 1671 19.540 1672 19.160 1673 17.648 1674 24.228 1675 20.436 1676 20.264 1677 21.196 1678 19.008 1679 17.448 1680 17.388 1681 18.472 1682 20.656 1683 18.844 1684 19.104 1685 23.300 1686 19.888 1687 23.116 1688 19.252 1689 23.736 1690 17.832 1691 21.892 1692 18.696 1693 22.040 1694 18.248 1695 20.740 1696 19.588 1697 19.348 1698 23.020 1699 20.344 1700 17.644 1701 19.224 1702 19.576 1703 21.312 1704 19.708 1705 18.936 1706 22.664 1707 22.964 1708 19.840 1709 24.720 1710 18.820 1711 21.456 1712 19.240 1713 24.144 1714 21.868 1715 20.948 1716 20.420 1717 17.576 1718 17.832 1719 19.972 1720 19.388 1721 18.720 1722 18.348 1723 18.616 1724 20.236 1725 20.448 1726 21.912 1727 23.548 1728 22.524 1729 20.824 1730 18.772 1731 18.076 1732 22.360 1733 19.520 1734 18.864 1735 18.112 1736 20.756 1737 21.016 1738 22.636 1739 22.732 1740 19.344 1741 18.892 1742 20.196 1743 21.848 1744 22.624 1745 18.488 1746 21.312 1747 20.512 1748 22.228 1749 19.856 1750 20.836 1751 18.408 1752 21.560 1753 23.496 1754 22.008 1755 22.052 1756 18.908 1757 18.708 1758 19.940 1759 21.424 1760 21.028 1761 21.292 1762 21.304 1763 17.292 1764 24.040 1765 20.272 1766 23.704 1767 21.736 1768 22.040 1769 21.416 1770 21.728 1771 18.620 1772 23.712 1773 20.028 1774 21.856 1775 21.596 1776 20.156 1777 22.772 1778 21.148 1779 18.684 1780 21.864 1781 24.756 1782 19.840 1783 19.792 1784 20.700 1785 18.768 1786 20.940 1787 20.536 1788 20.408 1789 17.288 1790 22.760 1791 18.584 1792 20.104 1793 19.200 1794 23.040 1795 23.104 1796 19.236 1797 22.300 1798 23.076 1799 24.724 1800 23.028 1801 20.148 1802 20.116 1803 19.900 1804 20.488 1805 22.496 1806 17.596 1807 21.176 1808 17.672 1809 22.468 1810 23.140 1811 18.936 1812 22.796 1813 20.916 1814 24.400 1815 19.572 1816 20.420 1817 19.804 1818 18.696 1819 16.488 1820 18.552 1821 18.500 1822 21.224 1823 20.616 1824 18.688 1825 17.232 1826 26.800 1827 18.692 1828 21.220 1829 20.440 1830 22.368 1831 19.064 1832 19.292 1833 20.656 1834 21.116 1835 21.268 1836 21.276 1837 18.972 1838 24.768 1839 23.216 1840 17.116 1841 20.168 1842 21.756 1843 20.524 1844 20.796 1845 17.804 1846 20.372 1847 19.888 1848 21.268 1849 20.416 1850 20.304 1851 20.128 1852 19.740 1853 20.488 1854 20.276 1855 20.508 1856 20.000 1857 19.948 1858 21.156 1859 20.900 1860 18.728 1861 21.028 1862 19.508 1863 19.028 1864 18.104 1865 18.672 1866 20.028 1867 20.796 1868 22.176 1869 16.688 1870 20.796 1871 22.484 1872 18.804 1873 19.196 1874 20.296 1875 19.284 1876 24.428 1877 18.644 1878 20.928 1879 19.872 1880 20.228 1881 24.032 1882 21.612 1883 19.220 1884 19.136 1885 19.896 1886 21.284 1887 20.656 1888 20.596 1889 21.488 1890 21.564 1891 18.380 1892 21.864 1893 19.712 1894 16.748 1895 21.308 1896 18.992 1897 21.948 1898 22.888 1899 20.492 1900 21.252 1901 20.284 1902 23.304 1903 19.076 1904 20.972 1905 22.384 1906 19.704 1907 16.324 1908 21.412 1909 19.732 1910 18.420 1911 20.076 1912 19.784 1913 17.068 1914 20.908 1915 19.232 1916 19.888 1917 19.660 1918 19.296 1919 21.284 1920 19.688 1921 19.932 1922 21.204 1923 20.760 1924 20.224 1925 16.016 1926 19.220 1927 19.816 1928 22.264 1929 21.428 1930 21.948 1931 21.256 1932 20.928 1933 19.004 1934 21.624 1935 18.664 1936 22.304 1937 19.844 1938 21.668 1939 20.780 1940 22.828 1941 20.596 1942 19.212 1943 21.088 1944 20.184 1945 21.684 1946 17.904 1947 20.508 1948 20.540 1949 22.580 1950 18.528 1951 19.540 1952 22.032 1953 19.372 1954 20.940 1955 18.060 1956 18.584 1957 20.752 1958 18.308 1959 21.028 1960 19.368 1961 18.648 1962 20.156 1963 21.136 1964 16.992 1965 20.172 1966 20.612 1967 20.784 1968 20.768 1969 18.596 1970 19.092 1971 18.124 1972 20.596 1973 21.316 1974 21.344 1975 22.768 1976 21.372 1977 23.312 1978 18.552 1979 21.656 1980 23.220 1981 20.084 1982 22.256 1983 20.664 1984 17.412 1985 21.420 1986 22.180 1987 20.064 1988 20.508 1989 23.884 1990 20.488 1991 18.300 1992 18.776 1993 23.868 1994 22.320 1995 18.640 1996 20.064 1997 18.440 1998 23.216 1999 19.232 2000 16.448 2001 22.524 2002 20.324 2003 23.288 2004 21.268 2005 19.252 2006 20.924 2007 21.304 2008 22.748 2009 20.464 2010 21.828 2011 15.608 2012 22.788 2013 19.896 2014 21.744 2015 21.088 2016 18.884 2017 19.516 2018 23.620 2019 20.940 2020 19.180 2021 18.692 2022 20.832 2023 19.024 2024 18.124 2025 18.020 2026 20.416 2027 21.700 2028 17.760 2029 20.944 2030 22.600 2031 19.192 2032 20.704 2033 24.984 2034 22.424 2035 17.244 2036 22.740 2037 20.940 2038 19.220 2039 22.508 2040 22.008 2041 19.984 2042 21.940 2043 17.564 2044 18.100 2045 18.968 2046 22.864 2047 18.152 2048 21.652 2049 21.160 2050 21.128 2051 19.572 2052 23.184 2053 19.416 2054 18.028 2055 21.868 2056 20.572 2057 20.948 2058 21.996 2059 19.776 2060 17.996 2061 19.876 2062 21.468 2063 19.248 2064 20.456 2065 21.232 2066 20.416 2067 19.184 2068 18.364 2069 16.520 2070 21.040 2071 18.936 2072 19.320 2073 20.708 2074 20.464 2075 20.232 2076 19.256 2077 20.552 2078 21.560 2079 20.236 2080 19.300 2081 21.032 2082 21.824 2083 20.436 2084 19.992 2085 19.248 2086 23.992 2087 24.308 2088 23.524 2089 20.136 2090 15.668 2091 21.128 2092 19.332 2093 24.104 2094 21.312 2095 23.244 2096 18.004 2097 17.144 2098 19.904 2099 17.844 2100 21.536 2101 22.908 2102 22.152 2103 20.188 2104 19.876 2105 19.672 2106 22.164 2107 18.584 2108 21.328 2109 16.736 2110 19.596 2111 20.920 2112 18.480 2113 18.484 2114 22.352 2115 20.612 2116 23.860 2117 23.928 2118 17.516 2119 22.252 2120 19.868 2121 23.308 2122 21.284 2123 20.088 2124 20.308 2125 19.204 2126 24.252 2127 21.768 2128 16.320 2129 20.012 2130 19.504 2131 20.236 2132 18.296 2133 18.520 2134 22.008 2135 20.384 2136 21.812 2137 18.696 2138 20.392 2139 23.720 2140 21.152 2141 20.104 2142 20.132 2143 21.352 2144 21.180 2145 17.700 2146 20.044 2147 17.868 2148 18.060 2149 18.632 2150 16.740 2151 19.316 2152 18.436 2153 17.384 2154 19.380 2155 18.992 2156 17.440 2157 19.584 2158 18.232 2159 24.196 2160 20.452 2161 21.284 2162 20.032 2163 23.220 2164 21.332 2165 19.992 2166 24.044 2167 18.948 2168 18.944 2169 22.516 2170 21.744 2171 19.216 2172 20.224 2173 23.480 2174 21.136 2175 16.684 2176 19.960 2177 22.292 2178 20.636 2179 20.488 2180 19.188 2181 20.464 2182 22.572 2183 18.716 2184 18.272 2185 22.812 2186 17.312 2187 22.472 2188 20.160 2189 22.572 2190 21.776 2191 21.184 2192 22.304 2193 21.744 2194 18.092 2195 18.952 2196 19.704 2197 21.928 2198 19.060 2199 21.288 2200 23.792 2201 20.900 2202 19.932 2203 19.000 2204 19.644 2205 21.700 2206 21.192 2207 20.772 2208 20.548 2209 19.324 2210 21.984 2211 18.476 2212 19.436 2213 19.380 2214 19.036 2215 19.616 2216 19.052 2217 18.076 2218 21.064 2219 24.256 2220 18.680 2221 19.128 2222 20.648 2223 19.548 2224 21.460 2225 21.320 2226 17.116 2227 19.068 2228 19.316 2229 20.648 2230 19.040 2231 21.980 2232 21.540 2233 23.728 2234 20.464 2235 21.708 2236 20.792 2237 18.904 2238 20.664 2239 19.064 2240 21.080 2241 21.016 2242 19.176 2243 21.828 2244 22.572 2245 24.852 2246 17.620 2247 17.868 2248 19.872 2249 23.572 2250 23.524 2251 17.700 2252 17.504 2253 17.656 2254 21.144 2255 19.328 2256 20.656 2257 18.576 2258 20.276 2259 22.140 2260 20.500 2261 18.976 2262 17.788 2263 19.432 2264 19.528 2265 21.376 2266 19.796 2267 19.088 2268 16.424 2269 20.900 2270 23.500 2271 17.812 2272 22.832 2273 19.184 2274 17.764 2275 23.664 2276 19.204 2277 24.248 2278 17.652 2279 20.344 2280 17.976 2281 23.808 2282 18.848 2283 22.256 2284 20.636 2285 21.560 2286 19.824 2287 18.920 2288 19.224 2289 19.764 2290 17.516 2291 24.584 2292 18.816 2293 18.824 2294 20.204 2295 21.628 2296 19.248 2297 19.732 2298 23.528 2299 19.912 2300 21.036 2301 23.224 2302 19.904 2303 18.616 2304 18.080 2305 20.488 2306 15.180 2307 25.024 2308 21.012 2309 18.532 2310 22.024 2311 21.856 2312 20.404 2313 18.244 2314 18.000 2315 20.204 2316 21.704 2317 21.248 2318 19.344 2319 20.728 2320 20.096 2321 22.580 2322 19.856 2323 19.660 2324 19.336 2325 20.276 2326 22.748 2327 21.164 2328 20.844 2329 24.076 2330 18.576 2331 19.848 2332 17.544 2333 21.580 2334 20.080 2335 20.324 2336 18.692 2337 20.028 2338 21.560 2339 18.080 2340 20.808 2341 22.084 2342 19.460 2343 20.244 2344 20.624 2345 22.404 2346 16.972 2347 18.268 2348 18.892 2349 21.624 2350 20.652 2351 22.984 2352 20.328 2353 21.956 2354 21.868 2355 20.508 2356 17.652 2357 20.544 2358 23.700 2359 23.852 2360 20.056 2361 19.632 2362 17.776 2363 18.468 2364 20.964 2365 21.576 2366 24.556 2367 23.776 2368 23.716 2369 18.184 2370 21.644 2371 19.100 2372 19.704 2373 19.144 2374 20.684 2375 21.988 2376 24.876 2377 19.264 2378 19.256 2379 19.808 2380 21.268 2381 17.372 2382 16.836 2383 18.740 2384 17.584 2385 20.452 2386 16.636 2387 19.620 2388 22.692 2389 20.744 2390 19.508 2391 22.000 2392 20.200 2393 21.824 2394 22.336 2395 18.328 2396 20.880 2397 17.200 2398 16.648 2399 20.324 2400 21.864 2401 21.440 2402 19.004 2403 20.028 2404 22.148 2405 20.668 2406 24.628 2407 21.684 2408 23.028 2409 21.908 2410 24.272 2411 18.688 2412 24.460 2413 21.024 2414 20.940 2415 16.636 2416 20.548 2417 17.984 2418 23.648 2419 15.972 2420 19.552 2421 18.196 2422 19.320 2423 22.184 2424 18.832 2425 22.592 2426 20.116 2427 21.484 2428 16.480 2429 20.732 2430 21.476 2431 22.144 2432 18.368 2433 21.668 2434 21.680 2435 19.164 2436 21.420 2437 21.400 2438 19.056 2439 18.232 2440 18.980 2441 22.308 2442 17.716 2443 20.344 2444 25.208 2445 21.824 2446 20.080 2447 21.624 2448 24.300 2449 22.784 2450 18.668 2451 19.292 2452 20.884 2453 22.252 2454 19.744 2455 18.272 2456 19.544 2457 21.252 2458 21.072 2459 20.016 2460 18.764 2461 20.296 2462 20.948 2463 23.296 2464 21.492 2465 19.420 2466 17.784 2467 20.496 2468 20.632 2469 19.436 2470 18.232 2471 20.140 2472 19.468 2473 21.172 2474 20.120 2475 19.216 2476 21.680 2477 19.504 2478 21.284 2479 22.428 2480 19.012 2481 20.180 2482 21.216 2483 21.016 2484 22.628 2485 21.740 2486 21.528 2487 22.920 2488 22.008 2489 20.812 2490 21.948 2491 22.528 2492 22.448 2493 20.140 2494 20.340 2495 20.940 2496 23.736 2497 21.684 2498 22.196 2499 18.908 2500 21.860 2501 17.888 2502 22.532 2503 20.096 2504 18.260 2505 18.348 2506 22.280 2507 20.364 2508 24.452 2509 20.928 2510 19.220 2511 19.384 2512 24.708 2513 19.904 2514 19.736 2515 20.988 2516 21.412 2517 20.476 2518 19.056 2519 19.400 2520 17.192 2521 21.708 2522 19.260 2523 18.228 2524 18.624 2525 19.008 2526 19.404 2527 19.408 2528 19.920 2529 20.032 2530 17.360 2531 18.684 2532 20.928 2533 20.604 2534 19.824 2535 20.296 2536 19.696 2537 22.544 2538 19.604 2539 16.364 2540 21.716 2541 19.408 2542 20.344 2543 19.632 2544 20.956 2545 21.320 2546 17.808 2547 20.872 2548 22.516 2549 22.052 2550 16.308 2551 15.436 2552 22.812 2553 19.780 2554 22.956 2555 22.112 2556 19.008 2557 17.964 2558 21.704 2559 19.252 2560 19.132 2561 19.472 2562 18.700 2563 18.768 2564 20.768 2565 22.384 2566 20.808 2567 21.336 2568 20.352 2569 18.840 2570 22.624 2571 16.884 2572 17.152 2573 21.348 2574 19.808 2575 20.224 2576 19.076 2577 20.328 2578 19.292 2579 15.852 2580 22.016 2581 25.368 2582 19.160 2583 17.864 2584 21.024 2585 18.452 2586 20.976 2587 20.268 2588 23.004 2589 22.004 2590 22.192 2591 20.244 2592 18.396 2593 25.044 2594 23.172 2595 21.968 2596 20.996 2597 19.480 2598 19.708 2599 21.484 2600 18.028 2601 23.240 2602 16.152 2603 18.436 2604 18.280 2605 19.684 2606 20.216 2607 23.176 2608 16.556 2609 18.776 2610 23.244 2611 21.204 2612 21.648 2613 21.372 2614 19.768 2615 19.140 2616 23.396 2617 21.780 2618 21.600 2619 18.036 2620 22.108 2621 22.820 2622 23.528 2623 21.604 2624 19.852 2625 21.068 2626 20.208 2627 21.660 2628 17.960 2629 20.080 2630 18.404 2631 19.164 2632 18.980 2633 22.504 2634 17.840 2635 16.608 2636 19.784 2637 19.080 2638 19.168 2639 19.612 2640 19.708 2641 20.720 2642 18.064 2643 23.524 2644 18.648 2645 21.084 2646 16.580 2647 19.572 2648 19.516 2649 18.140 2650 21.496 2651 18.924 2652 18.184 2653 18.228 2654 19.960 2655 23.672 2656 22.340 2657 16.308 2658 21.956 2659 23.028 2660 21.620 2661 20.328 2662 19.820 2663 20.940 2664 19.032 2665 20.168 2666 16.288 2667 22.920 2668 20.564 2669 19.232 2670 23.080 2671 20.892 2672 17.500 2673 20.600 2674 20.064 2675 20.144 2676 20.184 2677 20.940 2678 20.064 2679 18.816 2680 21.716 2681 16.492 2682 21.136 2683 21.752 2684 20.444 2685 19.436 2686 21.164 2687 20.972 2688 22.164 2689 21.204 2690 20.608 2691 15.416 2692 20.964 2693 19.204 2694 23.060 2695 21.120 2696 18.416 2697 20.272 2698 18.248 2699 19.076 2700 22.404 2701 18.716 2702 17.360 2703 18.760 2704 18.648 2705 22.000 2706 21.376 2707 17.724 2708 22.080 2709 22.060 2710 20.856 2711 18.956 2712 19.160 2713 23.184 2714 22.412 2715 18.960 2716 22.388 2717 20.764 2718 19.520 2719 20.256 2720 21.352 2721 17.772 2722 17.832 2723 21.400 2724 20.840 2725 19.640 2726 20.000 2727 17.620 2728 23.708 2729 21.704 2730 24.176 2731 23.368 2732 20.184 2733 22.328 2734 20.948 2735 23.708 2736 20.396 2737 23.596 2738 21.356 2739 21.004 2740 19.644 2741 23.364 2742 19.576 2743 16.652 2744 22.044 2745 20.556 2746 21.552 2747 22.060 2748 21.700 2749 20.560 2750 16.528 2751 23.056 2752 21.780 2753 23.044 2754 17.356 2755 16.880 2756 23.576 2757 20.296 2758 20.708 2759 18.100 2760 17.868 2761 19.624 2762 24.068 2763 19.280 2764 23.164 2765 21.804 2766 21.856 2767 18.272 2768 21.176 2769 20.768 2770 23.676 2771 18.600 2772 18.004 2773 20.756 2774 21.484 2775 20.720 2776 18.712 2777 22.020 2778 19.472 2779 21.784 2780 21.584 2781 19.808 2782 19.716 2783 21.928 2784 20.348 2785 18.448 2786 21.780 2787 17.520 2788 19.156 2789 22.224 2790 18.984 2791 23.164 2792 20.692 2793 21.512 2794 23.452 2795 19.248 2796 20.664 2797 21.760 2798 22.064 2799 20.072 2800 21.524 2801 17.368 2802 21.484 2803 20.264 2804 19.440 2805 17.248 2806 22.840 2807 19.332 2808 17.960 2809 22.324 2810 21.848 2811 16.604 2812 21.572 2813 21.088 2814 20.644 2815 18.416 2816 21.684 2817 20.012 2818 19.492 2819 18.860 2820 21.132 2821 20.132 2822 17.944 2823 19.176 2824 17.880 2825 20.060 2826 18.900 2827 20.520 2828 21.940 2829 19.700 2830 22.236 2831 18.976 2832 20.048 2833 18.940 2834 19.084 2835 17.652 2836 19.752 2837 17.048 2838 24.884 2839 19.500 2840 18.140 2841 19.820 2842 17.872 2843 20.840 2844 21.988 2845 20.488 2846 19.708 2847 20.600 2848 18.916 2849 20.276 2850 20.908 2851 20.100 2852 21.572 2853 20.008 2854 23.552 2855 22.832 2856 19.472 2857 22.128 2858 22.692 2859 22.656 2860 18.864 2861 19.592 2862 20.908 2863 19.992 2864 24.032 2865 20.032 2866 19.088 2867 22.848 2868 20.056 2869 19.804 2870 17.844 2871 17.316 2872 19.776 2873 20.028 2874 19.344 2875 17.972 2876 20.020 2877 22.256 2878 19.456 2879 19.116 2880 22.064 2881 17.780 2882 22.136 2883 19.932 2884 16.592 2885 21.032 2886 17.272 2887 20.480 2888 22.240 2889 19.228 2890 19.836 2891 17.184 2892 21.404 2893 20.696 2894 21.896 2895 21.244 2896 21.444 2897 20.696 2898 20.728 2899 19.660 2900 20.820 2901 18.208 2902 19.688 2903 19.092 2904 19.608 2905 22.872 2906 17.664 2907 17.256 2908 22.428 2909 19.872 2910 19.204 2911 21.536 2912 19.540 2913 23.440 2914 21.812 2915 17.376 2916 24.744 2917 19.084 2918 17.656 2919 17.928 2920 18.932 2921 18.896 2922 20.144 2923 24.376 2924 21.356 2925 18.876 2926 18.900 2927 23.516 2928 20.992 2929 22.000 2930 20.056 2931 20.120 2932 21.356 2933 22.952 2934 18.700 2935 20.480 2936 22.252 2937 19.796 2938 19.144 2939 19.204 2940 20.736 2941 19.556 2942 20.296 2943 25.188 2944 21.440 2945 21.660 2946 21.640 2947 18.812 2948 22.552 2949 21.020 2950 19.920 2951 19.740 2952 20.096 2953 19.276 2954 21.236 2955 20.888 2956 19.684 2957 18.956 2958 21.252 2959 21.364 2960 19.468 2961 19.280 2962 21.096 2963 21.376 2964 20.684 2965 23.888 2966 20.180 2967 21.204 2968 16.592 2969 19.472 2970 20.212 2971 19.888 2972 18.412 2973 20.084 2974 19.640 2975 19.616 2976 18.120 2977 20.724 2978 23.092 2979 18.984 2980 19.356 2981 19.300 2982 21.112 2983 19.088 2984 21.104 2985 19.212 2986 18.360 2987 20.964 2988 21.540 2989 22.276 2990 20.316 2991 17.848 2992 16.092 2993 17.600 2994 21.200 2995 20.396 2996 16.692 2997 19.768 2998 19.256 2999 23.500 3000 20.056 3001 23.336 3002 21.332 3003 22.200 3004 21.068 3005 21.204 3006 18.892 3007 17.788 3008 20.680 3009 19.500 3010 23.492 3011 16.788 3012 21.004 3013 23.728 3014 17.852 3015 18.520 3016 23.256 3017 20.016 3018 22.556 3019 18.496 3020 18.808 3021 20.560 3022 21.464 3023 20.300 3024 23.804 3025 22.644 3026 24.104 3027 20.620 3028 19.532 3029 20.180 3030 18.868 3031 19.600 3032 18.652 3033 18.380 3034 18.908 3035 19.920 3036 17.036 3037 19.644 3038 22.432 3039 17.692 3040 20.464 3041 21.636 3042 23.064 3043 20.568 3044 22.180 3045 16.960 3046 18.652 3047 18.816 3048 16.508 3049 21.404 3050 18.540 3051 19.044 3052 17.752 3053 18.412 3054 20.820 3055 20.844 3056 17.428 3057 21.484 3058 21.328 3059 20.920 3060 20.728 3061 21.536 3062 21.448 3063 19.308 3064 15.664 3065 17.896 3066 19.060 3067 18.736 3068 20.508 3069 23.420 3070 22.324 3071 16.612 3072 21.388 3073 18.244 3074 21.976 3075 22.292 3076 22.656 3077 22.292 3078 21.156 3079 17.960 3080 20.232 3081 18.600 3082 20.332 3083 21.088 3084 20.224 3085 20.592 3086 19.632 3087 22.780 3088 20.140 3089 21.412 3090 23.616 3091 20.796 3092 21.540 3093 18.160 3094 21.984 3095 19.180 3096 16.696 3097 21.816 3098 19.256 3099 21.372 3100 19.460 3101 23.868 3102 22.360 3103 24.016 3104 19.680 3105 19.224 3106 19.788 3107 22.144 3108 20.372 3109 21.188 3110 21.852 3111 21.456 3112 18.036 3113 21.820 3114 23.984 3115 21.812 3116 19.116 3117 20.084 3118 20.408 3119 20.024 3120 18.060 3121 22.884 3122 23.220 3123 20.784 3124 19.600 3125 23.568 3126 20.820 3127 23.632 3128 20.660 3129 21.280 3130 19.336 3131 20.184 3132 23.372 3133 21.200 3134 20.376 3135 18.692 3136 21.884 3137 19.812 3138 21.580 3139 20.508 3140 21.196 3141 18.460 3142 21.152 3143 20.684 3144 23.412 3145 20.292 3146 23.936 3147 19.420 3148 19.720 3149 18.768 3150 20.924 3151 17.552 3152 17.692 3153 20.976 3154 22.588 3155 20.516 3156 17.696 3157 18.244 3158 18.232 3159 25.312 3160 17.752 3161 22.068 3162 21.476 3163 21.220 3164 19.684 3165 20.600 3166 20.132 3167 22.036 3168 19.500 3169 23.292 3170 21.836 3171 24.624 3172 16.748 3173 21.844 3174 19.612 3175 20.988 3176 21.988 3177 19.552 3178 22.332 3179 21.404 3180 21.900 3181 19.920 3182 18.532 3183 21.284 3184 20.156 3185 18.500 3186 22.704 3187 21.060 3188 20.284 3189 22.648 3190 20.944 3191 18.572 3192 17.532 3193 21.764 3194 21.440 3195 18.912 3196 17.288 3197 18.104 3198 21.200 3199 20.024 3200 21.224 3201 19.656 3202 20.072 3203 21.996 3204 17.340 3205 18.132 3206 19.040 3207 17.068 3208 19.108 3209 20.712 3210 17.544 3211 20.124 3212 19.100 3213 21.060 3214 22.004 3215 20.536 3216 20.276 3217 18.884 3218 22.752 3219 20.568 3220 19.304 3221 20.552 3222 20.444 3223 20.060 3224 20.472 3225 15.908 3226 19.036 3227 19.720 3228 20.484 3229 21.112 3230 18.416 3231 21.132 3232 20.856 3233 19.912 3234 19.936 3235 22.244 3236 23.368 3237 22.180 3238 19.600 3239 19.984 3240 20.288 3241 21.064 3242 21.168 3243 18.712 3244 22.692 3245 20.548 3246 16.620 3247 23.092 3248 21.100 3249 19.836 3250 19.872 3251 20.004 3252 18.768 3253 24.240 3254 19.232 3255 20.896 3256 18.444 3257 19.200 3258 19.528 3259 20.444 3260 18.620 3261 20.500 3262 18.768 3263 20.248 3264 19.168 3265 24.532 3266 17.712 3267 20.532 3268 19.732 3269 17.624 3270 24.068 3271 20.956 3272 17.548 3273 19.124 3274 18.456 3275 17.928 3276 19.660 3277 19.832 3278 19.784 3279 19.084 3280 16.884 3281 19.848 3282 21.332 3283 22.856 3284 20.188 3285 20.592 3286 20.008 3287 20.704 3288 22.716 3289 21.044 3290 19.392 3291 20.408 3292 20.872 3293 21.792 3294 18.372 3295 22.664 3296 23.664 3297 18.988 3298 18.700 3299 19.700 3300 19.236 3301 21.144 3302 19.604 3303 21.864 3304 20.280 3305 20.412 3306 19.720 3307 19.756 3308 21.836 3309 22.448 3310 23.380 3311 20.368 3312 22.088 3313 18.112 3314 22.132 3315 21.468 3316 22.904 3317 19.972 3318 20.996 3319 17.684 3320 21.892 3321 19.300 3322 21.780 3323 19.464 3324 20.716 3325 23.268 3326 19.776 3327 20.656 3328 17.660 3329 20.076 3330 17.452 3331 20.376 3332 16.352 3333 20.080 3334 19.248 3335 20.168 3336 19.948 3337 21.856 3338 20.080 3339 19.836 3340 21.904 3341 18.624 3342 18.860 3343 18.788 3344 19.172 3345 23.044 3346 18.436 3347 19.480 3348 20.960 3349 20.296 3350 21.776 3351 22.208 3352 17.404 3353 18.912 3354 23.408 3355 18.752 3356 21.196 3357 23.036 3358 21.164 3359 20.464 3360 23.316 3361 21.276 3362 19.692 3363 21.900 3364 20.476 3365 18.832 3366 16.592 3367 23.236 3368 18.892 3369 18.948 3370 17.744 3371 17.280 3372 22.564 3373 20.556 3374 21.228 3375 18.528 3376 23.132 3377 16.172 3378 21.748 3379 20.104 3380 22.840 3381 23.156 3382 22.460 3383 22.220 3384 18.992 3385 21.944 3386 16.232 3387 18.656 3388 18.468 3389 23.944 3390 20.548 3391 19.804 3392 22.632 3393 19.532 3394 19.100 3395 19.904 3396 19.168 3397 23.868 3398 20.584 3399 18.768 3400 20.068 3401 19.440 3402 18.232 3403 22.524 3404 19.308 3405 20.652 3406 22.272 3407 16.380 3408 18.980 3409 18.016 3410 19.980 3411 20.684 3412 21.060 3413 21.272 3414 19.984 3415 20.980 3416 24.876 3417 20.072 3418 24.532 3419 21.316 3420 20.740 3421 18.504 3422 22.620 3423 22.292 3424 15.896 3425 19.140 3426 17.744 3427 19.376 3428 18.812 3429 22.032 3430 20.600 3431 19.712 3432 23.092 3433 20.200 3434 20.860 3435 20.496 3436 21.396 3437 16.848 3438 23.276 3439 23.000 3440 19.340 3441 19.908 3442 20.488 3443 16.284 3444 20.080 3445 17.652 3446 19.712 3447 20.560 3448 20.240 3449 17.188 3450 21.152 3451 22.420 3452 21.112 3453 19.408 3454 18.500 3455 18.292 3456 18.640 3457 19.152 3458 18.464 3459 17.080 3460 23.056 3461 18.756 3462 16.952 3463 19.580 3464 19.040 3465 21.196 3466 20.676 3467 20.868 3468 17.052 3469 17.396 3470 20.272 3471 21.916 3472 21.164 3473 19.436 3474 21.236 3475 17.156 3476 24.564 3477 22.420 3478 17.336 3479 19.968 3480 19.444 3481 19.256 3482 21.340 3483 20.680 3484 19.168 3485 19.452 3486 18.012 3487 20.204 3488 19.300 3489 20.288 3490 19.884 3491 20.136 3492 19.488 3493 18.472 3494 20.168 3495 18.132 3496 19.324 3497 21.420 3498 23.932 3499 20.112 3500 20.728 3501 20.776 3502 23.816 3503 17.420 3504 19.480 3505 21.520 3506 20.880 3507 20.396 3508 19.704 3509 18.616 3510 18.508 3511 21.632 3512 18.968 3513 15.952 3514 21.972 3515 19.884 3516 20.688 3517 24.384 3518 19.476 3519 21.184 3520 20.900 3521 20.280 3522 23.344 3523 21.584 3524 22.092 3525 18.052 3526 21.852 3527 20.104 3528 21.660 3529 23.204 3530 21.528 3531 20.824 3532 23.152 3533 21.456 3534 22.888 3535 21.096 3536 22.444 3537 19.148 3538 22.044 3539 21.928 3540 22.124 3541 21.932 3542 16.236 3543 21.460 3544 17.980 3545 17.412 3546 18.360 3547 19.040 3548 18.984 3549 16.632 3550 21.824 3551 22.156 3552 20.748 3553 21.236 3554 20.756 3555 17.856 3556 20.256 3557 22.368 3558 15.432 3559 19.692 3560 23.016 3561 19.652 3562 19.536 3563 19.648 3564 18.376 3565 20.212 3566 21.480 3567 22.176 3568 22.036 3569 19.916 3570 19.072 3571 19.312 3572 20.828 3573 18.724 3574 20.012 3575 17.236 3576 18.600 3577 18.612 3578 23.972 3579 20.576 3580 19.868 3581 18.820 3582 18.896 3583 20.700 3584 21.592 3585 18.108 3586 21.340 3587 22.924 3588 21.396 3589 18.896 3590 19.160 3591 19.396 3592 22.852 3593 22.260 3594 17.572 3595 19.092 3596 19.860 3597 22.376 3598 18.104 3599 20.616 3600 19.500 3601 22.220 3602 19.296 3603 21.640 3604 18.528 3605 19.400 3606 24.272 3607 19.784 3608 18.776 3609 20.156 3610 19.164 3611 17.592 3612 20.060 3613 19.872 3614 20.040 3615 20.452 3616 18.772 3617 17.796 3618 19.636 3619 17.772 3620 19.584 3621 17.712 3622 18.404 3623 18.988 3624 19.160 3625 23.088 3626 21.356 3627 17.024 3628 22.168 3629 19.068 3630 19.940 3631 19.660 3632 17.664 3633 21.160 3634 23.460 3635 18.100 3636 19.620 3637 19.372 3638 20.688 3639 20.176 3640 17.156 3641 17.704 3642 20.848 3643 17.304 3644 19.928 3645 22.244 3646 16.360 3647 19.752 3648 20.792 3649 21.256 3650 20.128 3651 19.568 3652 18.072 3653 22.180 3654 20.576 3655 19.888 3656 21.188 3657 18.936 3658 24.512 3659 19.284 3660 19.780 3661 21.316 3662 18.860 3663 21.668 3664 18.648 3665 23.368 3666 20.248 3667 20.204 3668 21.836 3669 24.036 3670 19.784 3671 18.820 3672 18.420 3673 20.804 3674 19.688 3675 18.356 3676 19.596 3677 22.096 3678 20.568 3679 19.764 3680 22.320 3681 18.564 3682 24.572 3683 16.660 3684 20.976 3685 23.184 3686 20.576 3687 20.584 3688 18.468 3689 22.572 3690 19.144 3691 20.856 3692 20.588 3693 21.648 3694 22.972 3695 20.340 3696 21.136 3697 19.980 3698 21.984 3699 21.340 3700 21.800 3701 19.840 3702 20.484 3703 23.972 3704 19.764 3705 20.368 3706 23.344 3707 20.176 3708 19.044 3709 20.984 3710 21.636 3711 16.856 3712 20.636 3713 23.268 3714 19.708 3715 25.240 3716 19.800 3717 20.616 3718 20.824 3719 22.344 3720 18.404 3721 20.204 3722 19.552 3723 19.136 3724 21.164 3725 19.864 3726 21.920 3727 20.472 3728 18.088 3729 17.240 3730 19.232 3731 19.032 3732 20.812 3733 18.932 3734 18.852 3735 20.404 3736 20.360 3737 22.104 3738 23.232 3739 21.612 3740 19.084 3741 20.712 3742 17.916 3743 18.624 3744 22.904 3745 24.148 3746 19.772 3747 18.884 3748 20.596 3749 20.184 3750 20.944 3751 20.672 3752 20.708 3753 21.628 3754 21.308 3755 17.820 3756 22.372 3757 19.604 3758 20.152 3759 20.196 3760 22.436 3761 22.608 3762 19.920 3763 21.544 3764 21.056 3765 22.332 3766 17.548 3767 16.940 3768 19.628 3769 17.976 3770 20.892 3771 19.412 3772 21.812 3773 18.896 3774 19.736 3775 19.452 3776 19.480 3777 18.156 3778 17.448 3779 16.004 3780 20.032 3781 20.836 3782 20.704 3783 22.932 3784 20.212 3785 21.328 3786 21.548 3787 19.820 3788 21.852 3789 20.248 3790 20.208 3791 19.612 3792 20.560 3793 16.184 3794 18.472 3795 19.244 3796 20.008 3797 19.752 3798 22.248 3799 19.260 3800 19.928 3801 18.568 3802 23.124 3803 21.332 3804 21.680 3805 19.544 3806 21.120 3807 19.976 3808 21.536 3809 22.912 3810 23.432 3811 23.836 3812 18.984 3813 21.384 3814 21.208 3815 22.544 3816 21.064 3817 20.244 3818 19.576 3819 21.132 3820 17.544 3821 23.544 3822 18.724 3823 21.552 3824 18.696 3825 21.128 3826 20.360 3827 21.532 3828 21.532 3829 19.912 3830 21.084 3831 21.556 3832 22.092 3833 22.700 3834 20.208 3835 19.044 3836 21.048 3837 22.516 3838 20.320 3839 18.100 3840 16.672 3841 20.008 3842 18.420 3843 23.076 3844 18.680 3845 17.908 3846 19.728 3847 20.784 3848 18.476 3849 21.556 3850 18.784 3851 20.544 3852 20.708 3853 17.436 3854 16.936 3855 19.544 3856 23.132 3857 18.432 3858 20.164 3859 18.668 3860 21.628 3861 22.092 3862 19.352 3863 22.200 3864 19.780 3865 20.092 3866 22.868 3867 18.228 3868 18.704 3869 22.316 3870 19.608 3871 20.232 3872 21.828 3873 16.260 3874 19.900 3875 21.568 3876 21.944 3877 19.948 3878 18.840 3879 22.204 3880 21.988 3881 19.404 3882 21.244 3883 20.344 3884 20.372 3885 19.404 3886 19.140 3887 21.444 3888 21.472 3889 21.680 3890 17.516 3891 22.452 3892 20.172 3893 18.616 3894 19.172 3895 22.924 3896 22.916 3897 21.276 3898 19.284 3899 19.060 3900 20.452 3901 20.156 3902 21.020 3903 21.516 3904 20.692 3905 18.812 3906 19.396 3907 20.776 3908 19.408 3909 18.172 3910 18.484 3911 19.620 3912 19.432 3913 21.400 3914 16.700 3915 20.916 3916 19.764 3917 19.672 3918 22.060 3919 19.784 3920 20.140 3921 19.080 3922 19.184 3923 22.520 3924 20.736 3925 22.604 3926 21.032 3927 20.880 3928 19.996 3929 19.144 3930 21.000 3931 20.456 3932 20.756 3933 23.400 3934 21.676 3935 21.524 3936 20.520 3937 20.260 3938 22.776 3939 21.900 3940 23.468 3941 22.904 3942 21.960 3943 21.820 3944 18.492 3945 21.408 3946 20.988 3947 20.840 3948 19.952 3949 23.708 3950 16.588 3951 21.248 3952 19.848 3953 21.828 3954 19.380 3955 18.676 3956 19.592 3957 21.204 3958 20.440 3959 21.376 3960 23.104 3961 24.744 3962 21.924 3963 23.264 3964 20.788 3965 19.556 3966 18.856 3967 17.780 3968 20.532 3969 20.264 3970 20.180 3971 20.688 3972 22.628 3973 19.072 3974 17.636 3975 20.556 3976 19.360 3977 21.244 3978 21.052 3979 22.860 3980 20.732 3981 22.584 3982 16.508 3983 17.940 3984 22.036 3985 19.176 3986 20.752 3987 20.332 3988 21.248 3989 17.740 3990 23.384 3991 22.504 3992 22.136 3993 20.756 3994 20.796 3995 18.228 3996 23.292 3997 23.416 3998 16.396 3999 17.552 4000 17.924 4001 17.776 4002 20.748 4003 22.192 4004 21.572 4005 22.344 4006 21.996 4007 21.680 4008 19.268 4009 22.252 4010 18.720 4011 20.344 4012 21.364 4013 17.700 4014 23.664 4015 20.280 4016 20.980 4017 20.136 4018 18.328 4019 20.852 4020 20.292 4021 20.504 4022 21.252 4023 20.300 4024 21.044 4025 24.608 4026 21.328 4027 19.736 4028 20.484 4029 22.912 4030 21.332 4031 21.212 4032 18.496 4033 21.512 4034 18.992 4035 16.596 4036 19.740 4037 24.096 4038 17.244 4039 18.956 4040 21.064 4041 22.620 4042 19.488 4043 20.344 4044 20.132 4045 22.004 4046 17.724 4047 23.020 4048 23.512 4049 20.432 4050 19.852 4051 17.528 4052 19.424 4053 22.056 4054 19.688 4055 17.792 4056 21.172 4057 20.560 4058 19.060 4059 23.868 4060 22.184 4061 17.372 4062 19.644 4063 21.124 4064 19.884 4065 22.440 4066 18.656 4067 19.352 4068 21.192 4069 20.028 4070 19.240 4071 21.384 4072 18.008 4073 19.876 4074 19.856 4075 18.464 4076 24.320 4077 20.200 4078 24.708 4079 19.828 4080 22.412 4081 22.012 4082 19.420 4083 21.968 4084 20.344 4085 20.792 4086 18.856 4087 21.312 4088 20.968 4089 18.524 4090 17.800 4091 22.356 4092 21.676 4093 20.460 4094 17.504 4095 18.972 4096 22.596 4097 19.136 4098 19.616 4099 20.832 4100 20.112 4101 21.180 4102 20.392 4103 21.548 4104 21.788 4105 24.032 4106 19.852 4107 16.000 4108 20.000 4109 22.164 4110 20.684 4111 18.572 4112 23.300 4113 19.632 4114 19.900 4115 21.636 4116 16.828 4117 21.520 4118 22.696 4119 22.148 4120 23.184 4121 19.008 4122 20.616 4123 18.428 4124 21.800 4125 19.716 4126 20.400 4127 23.160 4128 21.756 4129 17.932 4130 17.656 4131 19.316 4132 18.916 4133 19.772 4134 18.276 4135 18.336 4136 22.104 4137 18.176 4138 20.124 4139 17.876 4140 22.096 4141 18.380 4142 21.240 4143 21.456 4144 22.528 4145 22.520 4146 21.160 4147 16.812 4148 20.284 4149 19.560 4150 21.372 4151 21.412 4152 19.388 4153 20.064 4154 21.932 4155 19.320 4156 18.504 4157 19.324 4158 19.364 4159 21.408 4160 22.372 4161 24.348 4162 19.328 4163 21.884 4164 18.880 4165 21.376 4166 20.924 4167 21.332 4168 21.008 4169 22.192 4170 17.304 4171 20.548 4172 19.368 4173 22.200 4174 16.976 4175 21.528 4176 20.152 4177 22.224 4178 17.896 4179 21.876 4180 22.500 4181 17.524 4182 22.868 4183 21.088 4184 20.688 4185 21.392 4186 21.880 4187 20.576 4188 17.736 4189 23.092 4190 18.544 4191 18.396 4192 20.972 4193 19.332 4194 20.432 4195 19.868 4196 20.448 4197 19.764 4198 19.644 4199 21.228 4200 18.036 4201 20.136 4202 18.640 4203 20.956 4204 17.400 4205 19.552 4206 22.376 4207 18.452 4208 23.308 4209 21.668 4210 21.140 4211 21.764 4212 20.920 4213 20.840 4214 17.132 4215 18.044 4216 18.396 4217 18.976 4218 21.408 4219 21.064 4220 22.252 4221 20.856 4222 20.728 4223 20.328 4224 18.960 4225 17.824 4226 22.716 4227 19.872 4228 19.996 4229 19.344 4230 20.032 4231 22.568 4232 20.816 4233 20.188 4234 16.516 4235 22.436 4236 23.060 4237 21.108 4238 19.472 4239 21.396 4240 19.220 4241 22.856 4242 19.604 4243 17.676 4244 22.640 4245 19.532 4246 18.696 4247 16.728 4248 20.168 4249 19.408 4250 22.268 4251 17.860 4252 22.580 4253 20.840 4254 20.244 4255 19.932 4256 21.176 4257 20.404 4258 21.284 4259 20.108 4260 20.404 4261 19.656 4262 21.388 4263 18.600 4264 21.872 4265 18.376 4266 22.768 4267 18.184 4268 19.624 4269 18.572 4270 23.036 4271 19.936 4272 20.172 4273 19.084 4274 19.492 4275 17.628 4276 18.484 4277 21.860 4278 20.836 4279 23.028 4280 20.840 4281 20.784 4282 19.608 4283 22.416 4284 19.036 4285 21.520 4286 20.232 4287 22.032 4288 18.944 4289 18.656 4290 21.564 4291 23.052 4292 23.000 4293 23.372 4294 21.096 4295 20.940 4296 22.100 4297 18.496 4298 20.420 4299 21.068 4300 23.388 4301 17.060 4302 21.260 4303 19.128 4304 18.880 4305 20.036 4306 19.176 4307 23.268 4308 19.628 4309 22.004 4310 20.788 4311 23.468 4312 25.536 4313 22.624 4314 18.204 4315 18.812 4316 16.436 4317 22.112 4318 23.300 4319 19.008 4320 21.584 4321 19.892 4322 19.636 4323 19.032 4324 19.704 4325 17.628 4326 22.696 4327 18.856 4328 18.644 4329 23.040 4330 16.280 4331 19.364 4332 20.336 4333 22.116 4334 21.936 4335 18.324 4336 18.592 4337 19.132 4338 25.948 4339 19.068 4340 19.608 4341 19.900 4342 18.716 4343 20.492 4344 20.532 4345 22.808 4346 20.816 4347 19.128 4348 19.284 4349 21.468 4350 19.576 4351 22.716 4352 21.140 4353 20.624 4354 22.748 4355 22.896 4356 21.076 4357 18.980 4358 19.064 4359 21.904 4360 20.320 4361 22.092 4362 21.264 4363 16.704 4364 20.808 4365 18.884 4366 22.264 4367 23.004 4368 22.496 4369 22.068 4370 23.996 4371 19.412 4372 20.020 4373 20.560 4374 19.756 4375 21.912 4376 19.188 4377 25.184 4378 16.556 4379 22.712 4380 19.904 4381 20.664 4382 21.704 4383 19.532 4384 23.748 4385 16.208 4386 19.380 4387 18.028 4388 18.024 4389 16.644 4390 20.716 4391 17.800 4392 21.824 4393 21.344 4394 19.760 4395 21.384 4396 18.432 4397 20.384 4398 17.316 4399 22.688 4400 20.984 4401 20.856 4402 20.284 4403 21.464 4404 20.588 4405 19.704 4406 20.644 4407 22.984 4408 19.392 4409 16.716 4410 16.004 4411 18.788 4412 20.932 4413 19.680 4414 19.820 4415 17.208 4416 20.684 4417 21.264 4418 21.636 4419 19.508 4420 17.576 4421 19.736 4422 19.992 4423 20.260 4424 16.920 4425 19.836 4426 19.856 4427 17.880 4428 19.824 4429 21.392 4430 20.044 4431 22.532 4432 18.936 4433 17.636 4434 17.408 4435 21.504 4436 16.588 4437 22.968 4438 20.932 4439 18.864 4440 19.396 4441 21.588 4442 19.968 4443 22.700 4444 20.484 4445 21.860 4446 19.832 4447 23.208 4448 18.832 4449 21.428 4450 18.856 4451 22.020 4452 23.520 4453 20.052 4454 23.428 4455 19.640 4456 17.816 4457 23.096 4458 21.976 4459 19.376 4460 21.432 4461 18.524 4462 18.292 4463 22.788 4464 17.528 4465 21.636 4466 22.372 4467 18.272 4468 21.604 4469 22.268 4470 21.764 4471 21.348 4472 19.068 4473 19.224 4474 17.916 4475 20.076 4476 21.032 4477 20.592 4478 22.980 4479 21.704 4480 17.020 4481 19.596 4482 22.940 4483 21.344 4484 21.428 4485 18.532 4486 22.320 4487 21.320 4488 19.448 4489 21.200 4490 21.468 4491 20.872 4492 18.984 4493 22.804 4494 19.980 4495 18.772 4496 21.916 4497 22.780 4498 17.672 4499 21.656 4500 17.136 4501 21.020 4502 18.020 4503 17.904 4504 19.516 4505 21.808 4506 19.188 4507 23.100 4508 21.232 4509 19.012 4510 20.668 4511 19.216 4512 20.132 4513 23.516 4514 21.600 4515 19.460 4516 23.044 4517 19.036 4518 21.792 4519 19.496 4520 19.360 4521 21.560 4522 18.452 4523 19.720 4524 18.876 4525 20.264 4526 19.764 4527 18.616 4528 22.256 4529 21.548 4530 18.952 4531 19.952 4532 18.656 4533 22.256 4534 23.836 4535 19.644 4536 19.296 4537 19.116 4538 22.064 4539 22.700 4540 19.192 4541 21.424 4542 21.112 4543 20.368 4544 17.524 4545 21.280 4546 19.292 4547 21.848 4548 19.184 4549 18.912 4550 18.008 4551 19.960 4552 19.856 4553 23.332 4554 19.876 4555 21.256 4556 21.908 4557 21.232 4558 21.852 4559 18.028 4560 19.576 4561 20.984 4562 19.508 4563 25.556 4564 17.884 4565 19.304 4566 21.800 4567 19.812 4568 20.116 4569 20.460 4570 20.572 4571 19.916 4572 18.604 4573 19.828 4574 22.928 4575 17.380 4576 21.728 4577 20.584 4578 17.712 4579 22.960 4580 18.488 4581 17.776 4582 23.420 4583 20.224 4584 20.884 4585 22.364 4586 23.228 4587 22.268 4588 18.440 4589 22.516 4590 22.744 4591 21.232 4592 19.472 4593 18.832 4594 20.992 4595 20.688 4596 18.884 4597 20.200 4598 20.720 4599 22.412 4600 18.736 4601 17.176 4602 19.352 4603 22.416 4604 21.976 4605 18.688 4606 21.236 4607 16.900 4608 20.656 4609 19.204 4610 20.080 4611 19.308 4612 22.480 4613 19.500 4614 21.980 4615 19.972 4616 20.620 4617 20.676 4618 22.436 4619 21.084 4620 24.384 4621 22.088 4622 20.480 4623 19.768 4624 22.764 4625 20.156 4626 19.452 4627 18.436 4628 17.460 4629 22.288 4630 20.280 4631 17.984 4632 19.696 4633 20.040 4634 20.516 4635 20.872 4636 18.180 4637 19.324 4638 17.444 4639 18.956 4640 22.920 4641 21.492 4642 21.552 4643 21.728 4644 20.064 4645 20.420 4646 20.608 4647 22.964 4648 20.324 4649 20.984 4650 20.840 4651 18.016 4652 19.644 4653 19.972 4654 16.768 4655 21.540 4656 18.792 4657 19.488 4658 18.800 4659 23.020 4660 23.648 4661 19.676 4662 20.492 4663 22.380 4664 18.932 4665 21.180 4666 21.300 4667 19.428 4668 19.088 4669 20.136 4670 18.340 4671 20.284 4672 22.752 4673 20.044 4674 18.944 4675 22.192 4676 20.028 4677 22.532 4678 18.352 4679 21.040 4680 19.392 4681 19.864 4682 21.508 4683 20.776 4684 19.456 4685 23.280 4686 20.484 4687 20.932 4688 20.376 4689 20.828 4690 17.968 4691 21.028 4692 20.568 4693 19.444 4694 20.824 4695 21.100 4696 18.504 4697 19.440 4698 22.392 4699 18.192 4700 18.316 4701 20.072 4702 18.532 4703 20.756 4704 18.788 4705 23.376 4706 20.916 4707 22.464 4708 23.364 4709 17.184 4710 19.320 4711 21.636 4712 23.092 4713 19.480 4714 17.584 4715 22.400 4716 23.212 4717 20.468 4718 19.284 4719 22.900 4720 23.708 4721 22.452 4722 20.504 4723 19.908 4724 21.212 4725 21.228 4726 21.904 4727 21.844 4728 18.020 4729 20.920 4730 16.888 4731 21.116 4732 21.496 4733 21.148 4734 24.480 4735 19.416 4736 21.240 4737 17.920 4738 20.104 4739 22.168 4740 25.180 4741 21.124 4742 22.468 4743 20.728 4744 20.204 4745 18.116 4746 22.196 4747 19.848 4748 20.224 4749 19.032 4750 21.892 4751 28.372 4752 19.496 4753 18.712 4754 20.760 4755 19.604 4756 20.220 4757 20.960 4758 22.152 4759 16.860 4760 19.680 4761 22.248 4762 19.792 4763 16.616 4764 20.048 4765 19.896 4766 21.280 4767 19.784 4768 22.464 4769 18.668 4770 20.108 4771 16.092 4772 18.680 4773 19.440 4774 20.168 4775 17.012 4776 16.304 4777 20.860 4778 23.644 4779 25.064 4780 20.372 4781 20.488 4782 19.936 4783 21.352 4784 18.532 4785 19.372 4786 18.068 4787 17.616 4788 18.864 4789 18.028 4790 19.616 4791 17.516 4792 20.704 4793 21.120 4794 20.476 4795 21.120 4796 20.564 4797 16.572 4798 20.516 4799 20.292 4800 19.388 4801 19.976 4802 19.396 4803 20.600 4804 20.904 4805 20.184 4806 19.096 4807 19.620 4808 19.004 4809 20.756 4810 18.440 4811 16.472 4812 21.500 4813 20.844 4814 20.396 4815 22.344 4816 22.044 4817 20.524 4818 16.160 4819 17.224 4820 20.804 4821 20.260 4822 19.608 4823 19.020 4824 20.920 4825 19.396 4826 19.960 4827 17.844 4828 21.416 4829 18.780 4830 21.808 4831 22.220 4832 22.100 4833 22.076 4834 20.028 4835 18.608 4836 20.104 4837 21.116 4838 19.700 4839 21.516 4840 20.100 4841 21.744 4842 21.020 4843 21.528 4844 23.028 4845 19.528 4846 18.352 4847 23.392 4848 18.048 4849 21.528 4850 21.840 4851 24.676 4852 21.860 4853 18.696 4854 21.508 4855 21.768 4856 19.020 4857 19.504 4858 19.452 4859 20.468 4860 17.452 4861 20.904 4862 19.668 4863 18.204 4864 21.092 4865 19.120 4866 23.396 4867 18.548 4868 27.696 4869 21.180 4870 18.852 4871 21.684 4872 20.384 4873 17.700 4874 19.216 4875 18.260 4876 20.184 4877 18.828 4878 17.768 4879 20.240 4880 19.760 4881 18.800 4882 17.316 4883 19.568 4884 19.752 4885 23.776 4886 18.852 4887 20.076 4888 20.356 4889 17.788 4890 20.304 4891 22.032 4892 19.944 4893 20.236 4894 20.380 4895 17.080 4896 20.812 4897 20.820 4898 20.564 4899 20.152 4900 21.388 4901 21.424 4902 18.240 4903 23.280 4904 17.972 4905 21.624 4906 19.744 4907 17.544 4908 19.948 4909 21.248 4910 23.428 4911 19.196 4912 15.864 4913 21.164 4914 17.692 4915 17.980 4916 20.868 4917 19.544 4918 20.144 4919 19.388 4920 17.264 4921 19.228 4922 23.088 4923 21.168 4924 18.932 4925 20.252 4926 23.036 4927 21.788 4928 22.008 4929 19.768 4930 22.084 4931 22.424 4932 22.932 4933 18.388 4934 22.268 4935 19.248 4936 20.652 4937 18.420 4938 17.952 4939 20.012 4940 19.708 4941 20.228 4942 21.636 4943 19.484 4944 20.512 4945 18.148 4946 17.808 4947 22.320 4948 24.260 4949 20.448 4950 20.700 4951 20.716 4952 20.788 4953 19.600 4954 21.832 4955 18.052 4956 18.704 4957 21.492 4958 17.928 4959 21.180 4960 18.348 4961 19.904 4962 23.264 4963 18.200 4964 20.628 4965 18.860 4966 21.668 4967 16.948 4968 17.744 4969 19.416 4970 21.948 4971 15.872 4972 25.088 4973 20.256 4974 18.320 4975 21.968 4976 22.404 4977 18.844 4978 19.780 4979 18.748 4980 21.692 4981 18.744 4982 20.288 4983 20.028 4984 22.144 4985 19.512 4986 21.516 4987 19.232 4988 20.632 4989 20.028 4990 21.496 4991 21.900 4992 18.612 4993 20.848 4994 21.488 4995 20.776 4996 16.840 4997 19.784 4998 21.840 4999 22.608 5000 21.136 ``` --- ## Plot of sampling distribution ```r library(ggplot2) ggplot(data = sample_means, mapping = aes(x = mean_f)) + geom_histogram(color = "white", bins = 15) ``` 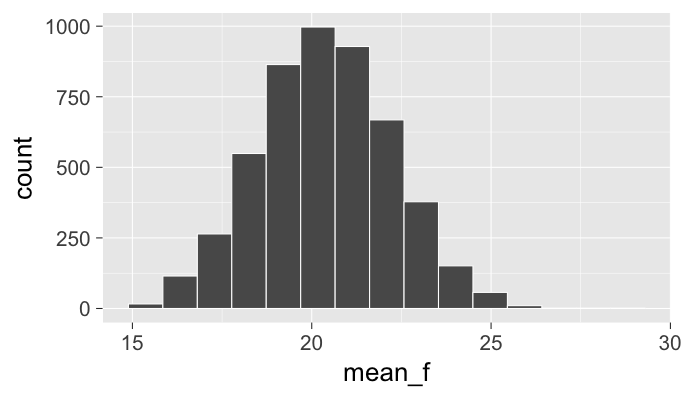<!-- --> --- name: meanofmeans ## Mean of the sampling distribution ### The mean of the sampling distribution of means (where the sampling distribution is centered) provides an estimate for our population mean: ```r sample_means %>% summarize(mean_samp_dist = mean(mean_f)) ``` ``` mean_samp_dist 1 20.38227 ``` -- ### Population mean ```r global13 %>% summarize(pop_mean = mean(FEMALEOFFICE)) ``` ``` # A tibble: 1 × 1 pop_mean <dbl> 1 20.38286 ``` --- name: stderror ## Standard deviation of the sampling distribution ### The standard deviation of the sampling distribution of means (also known as the <u>standard error</u>) provides an estimate of how much variability we can expect in the means as we go from one sample to another. ```r sample_means %>% summarize(std_error = sd(mean_f)) ``` ``` std_error 1 1.841328 ``` --- ## How could we improve our estimate of the population mean? -- - Increase the sample size -- - Run more simulations (Instead of `do()`ing 5000 we could `do()` 100,000, for example) --- ## What if we didn't have the whole population? -- ### We could <u>resample</u> one randomly selected sample multiple times to create a guess to what the sampling distribution might look like. -- ### Important note: This "guess" is called the <u>bootstrap distribution</u> and it is an approximation of the sampling distribution. -- ### The <u>bootstrap distribution</u> will always be centered near the original sample statistic so it is better used as an estimate of the shape of the sampling distribution and as an estimate of the standard error, but NOT as an estimate for the population center. --- ## Bootstrap our original sample - Let's use our original sample from `global13` which we called `sample1`. - Let's look to see what one such `resample()` of `sample1` gives us: ```r set.seed(2017) (boot1 <- sample1 %>% resample(orig.id = TRUE)) ``` ``` # A tibble: 25 × 3 country FEMALEOFFICE orig.id <chr> <dbl> <chr> 1 Argentina 39.8 24 2 Russia 11.5 14 3 Australia 29.7 12 4 Spain 33.6 8 5 Democratic Republic of the Congo 7.7 20 6 Democratic Republic of the Congo 7.7 20 7 South Africa 33.9 1 8 Chile 12.7 11 9 Australia 29.7 12 10 Canada 24.9 7 # ... with 15 more rows ``` --- ## Computing the bootstrap mean ```r boot1 %>% summarize(boot_mean = mean(FEMALEOFFICE)) ``` ``` # A tibble: 1 × 1 boot_mean <dbl> 1 23.596 ``` --- ## Repeating this process ### Repeating this process over and over again, we can create a <u>bootstrap distribution</u> from the means of the <u>bootstrap samples</u>. ```r boot_means <- do(5000) * sample1 %>% resample(orig.id = TRUE) %>% summarize(boot_mean = mean(FEMALEOFFICE)) ``` --- ## Plotting the bootstrap distribution ```r ggplot(data = boot_means, mapping = aes(x = boot_mean)) + geom_histogram(color = "white", bins = 15) ``` 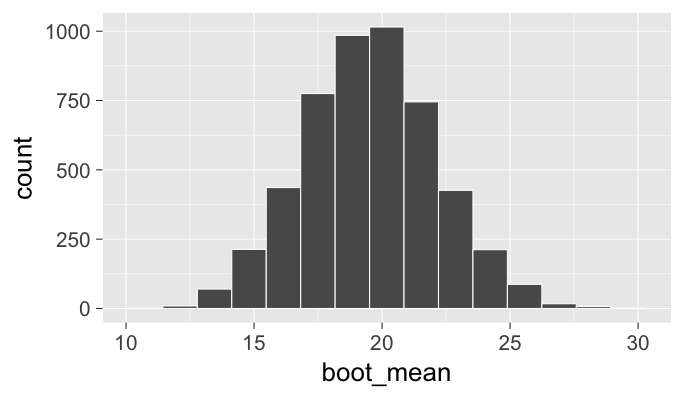<!-- --> --- ## Using the bootstrap distribution ### To estimate the <u>standard error</u> ```r boot_means %>% summarize(sd_boot_dist = sd(boot_mean)) ``` ``` sd_boot_dist 1 2.582481 ``` --- ## Using the bootstrap distribution ### To estimate the population mean ```r boot_means %>% summarize(mean_boot_dist = mean(boot_mean)) ``` ``` mean_boot_dist 1 19.54535 ``` -- - Hmm, that number looks familiar ```r sample1 %>% summarize(sample_mean = mean(FEMALEOFFICE)) ``` ``` # A tibble: 1 × 1 sample_mean <dbl> 1 19.576 ``` - What potential problems do we have with this estimate? --- # Providing a better estimate Point Estimate | Confidence Interval :-------------------------:|:-------------------------: <img src="figure/spear.jpg" alt="Drawing" style="width: 370px;"/> | <img src="figure/net.jpg" alt="Drawing" style="width: 370px;"/> --- ## Confidence Interval - A range of plausible values for a population parameter - Built using bootstrapping and percentiles of the bootstrap distribution ```r ( ciq_mean <- confint(boot_means, level = 0.95, method = "quantile") ) ``` ``` name lower upper level method estimate 1 boot_mean 14.6157 24.7641 0.95 percentile 19.576 ``` ### What does this mean? -- - Using a method that is 95% reliable, our range of plausible values for the true mean percentage of women holding national office is between around 14.6% to 24.8%. --- ## How did we fair using this sample? #### True population mean ```r global13 %>% summarize(pop_mean = mean(FEMALEOFFICE)) ``` ``` # A tibble: 1 × 1 pop_mean <dbl> 1 20.38286 ``` #### Confidence interval ```r ciq_mean ``` ``` name lower upper level method estimate 1 boot_mean 14.6157 24.7641 0.95 percentile 19.576 ``` -- - Would all random samples produce confidence intervals that include the population mean? If not, what percentage would you expect to? --- ## Summary - This activity was meant to show you how the concepts of **sampling** and **resampling** interact -- - Bootstrapping works by allowing us to create a guess as to what the sampling distribution looks like. - This allows us to test hypotheses and create confidence intervals on unknown population values. - It also allows us to not necessarily worry about formulas and mathematical probability. -- - It does still require us to have a random sample from our population as our **original sample** though. *Why is that?* --- name: ciprob ## Reason to be concerned - Remember that the original population distribution was right-skewed. Therefore, the mean doesn't make sense as a measure and may be causing some problems. - <u>Problem</u>: Repeat the **sampling**, **bootstrapping**, and **confidence interval** R code steps above, but this time using the median and the IQR instead of the mean and standard deviation. --- ## For PS13 (Due at the beginning of class on Tuesday) - Can be hand-written or shared as a Google Doc - Run the necessary code in an R script and explain your results from the Problem on the last slide. - Provide your modified code and explain what each line of code is doing. - What changes in the results of the analysis? - Do we get the same result as with the mean and standard deviation? - Read the article about [Margin of Errors and Polling](http://www.pewresearch.org/fact-tank/2016/09/08/understanding-the-margin-of-error-in-election-polls/) from Pew - In two to three paragraphs, summarize the article and discuss how confidence intervals are useful in polling. --- layout: true .footer[[Week 13 - Tuesday](https://ismayc.github.io/soc301_s2017/2017/04/25/week-13---tuesday/)] --- name: week13t class: center, middle # Week 13 - Tuesday # April 25, 2017 --- class: center, middle ## Warmup - Your answer should be 3-6 sentences. ### Discuss the importance of the "simulated data" step in the "There is Only One Test" framework. Why is it needed? What does it accomplish? <img src="figure/ht.png" width="1492" /> --- # Go over the "There is Only One Test" for Two Sample Independent problem ### Average income varies from one region of the country to another, and it often reflects both lifestyles and regional living expenses. Suppose a new graduate is considering a job in two locations, Cleveland, OH and Sacramento, CA, and he wants to see whether the average income in one of these cities is higher than the other. He would like to conduct a hypothesis test based on two randomly selected samples from the 2000 Census. --- class: center, middle # [Inference Mind-map](https://coggle.it/diagram/Vxlydu1akQFeqo6-) --- # Work through the problem in [R via R Markdown](http://ismayc.github.io/teaching/sample_problems/two-means-indep.html) - Skip the Confidence Interval parts (that's Chapter 8) --- ### How would this change if we were looking to potentially move to Seattle and Boston as well as Sacramento and Cleveland? <center> <img src="figure/ht.png" alt="Drawing" style="width: 580px;" /> </center> -- ### This is called an ANOVA (Analysis of Variance) test - It compares the variability in the sample means to the variability in the samples (Analyzes the variance) --- ## Chi-square test - The extension of a two sample proportion test is a chi-square test ### A random sample of 500 U.S. adults were questioned regarding their political affiliation (Democrat or Republican) and opinion on a tax reform bill (favor, indifferent, opposed). Based on this sample, do we have reason to believe that opinion on the bill can predict whether or not a respondent is a Democrat? - Here a success is "being a Democrat" - We want to test <small> `\(H_0: \pi_{favor} = \pi_{indiff} = \pi_{opp}\)` </small> versus <small> `\(H_A:\)` </small> at least one of the proportions is different --- Type | Test Statistic ------------- | ------------- | ------------- One-Sample | Sample Mean <br> `\(\overline{x}\)` One-Sample | Sample Proportion <br> `\(\widehat{p}\)` Two-Sample (Independent) | Diff of Sample Means <br> `\(\overline{x}_1 - \overline{x}_2\)` Two-Sample (Paired) | Mean Sample Difference <br> `\(\overline{x}_{diff}\)` Two-Sample Proportions | Diff of Sample Proportions <br> `\(\widehat{p}_1 - \widehat{p}_2\)` Multiple Sample (Independent) | MAD of Sample Means <br> `\(\text{Mean}_{i \ne j} \, ABS(\, \overline{x}_i - \overline{x}_j \, )\)` Multiple Sample Proportions | MAD of Sample Proportions <br> `\(\text{Mean}_{i \ne j} \, ABS(\, \hat{p}_i - \hat{p}_j \,)\)` --- class: center, middle # So when are the assumptions violated for traditional tests? # Why should we use randomization methods instead? --- ### Suppose we have the following two populations and we want to look for a difference in means ```r ggplot(data = skewed, mapping = aes(x = group, y = value)) + geom_boxplot() ``` 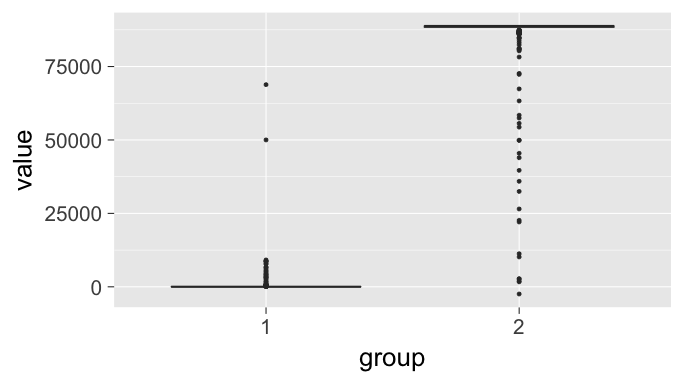<!-- --> --- ### We collect a sample of 20 from each ```r set.seed(2017) my_sample <- skewed %>% group_by(group) %>% sample_n(20) %>% ungroup() ggplot(data = my_sample, mapping = aes(x = value)) + geom_histogram(color = "white", bins = 10) + facet_wrap(~group, scales = "free_x") ``` 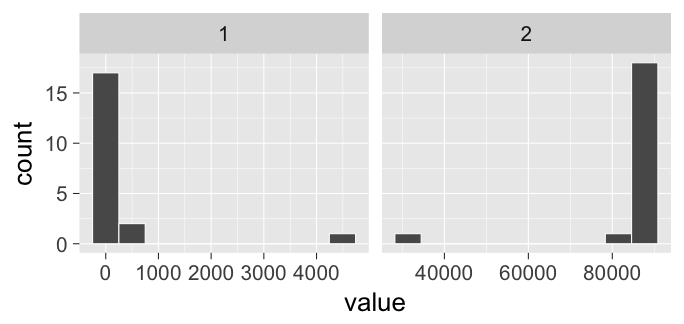<!-- --> --- ### A different plot ```r ggplot(data = my_sample, mapping = aes(x = group, y = value)) + geom_boxplot() ``` 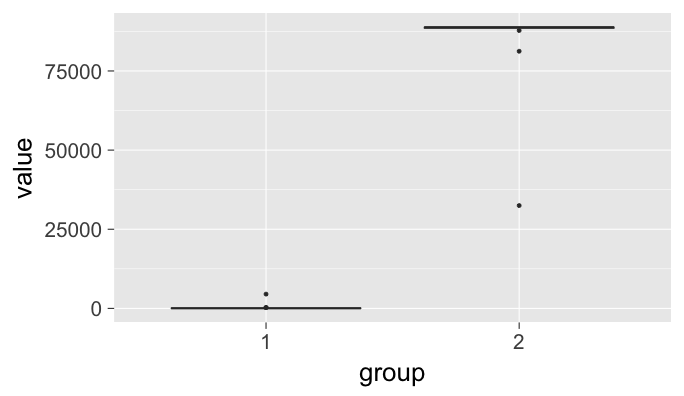<!-- --> --- ### We calculate the observed sample mean ```r (my_means <- my_sample %>% group_by(group) %>% summarize(my_mean = mean(value))) ``` ``` # A tibble: 2 × 2 group my_mean <chr> <dbl> 1 1 296.77 2 2 85509.68 ``` ```r (obs_diff <- diff(my_means$my_mean)) ``` ``` [1] 85212.9 ``` --- ### But what if we had collected a different sample instead <br> (assuming <small> `\(H_0\)` </small> is true) ```r library(mosaic) (shuffled_my_means <- my_sample %>% mutate(value = shuffle(value)) %>% group_by(group) %>% summarize(my_mean_shuf = mean(value))) ``` ``` # A tibble: 2 × 2 group my_mean_shuf <chr> <dbl> 1 1 45994.63 2 2 39811.81 ``` ```r (obs_shuf_diff <- diff(shuffled_my_means$my_mean_shuf)) ``` ``` [1] -6182.817 ``` --- ### And repeated this many times ```r many_shuffles <- do(5000) * (shuffled_my_means <- my_sample %>% mutate(value = shuffle(value)) %>% group_by(group) %>% summarize(my_mean_shuf = mean(value))) rand_distn <- many_shuffles %>% group_by(.index) %>% summarize(diffmean = diff(my_mean_shuf)) ``` --- ### And plotted the null distribution ```r ggplot(rand_distn, aes(x = diffmean)) + geom_histogram(color = "white", bins = 20) ``` <center> <img src="figure/Rplot.png" alt="Drawing" style="width: 330px;" /> </center> - This is not represented well by a bell-shaped curve --- # Moral of the story - Randomization methods will always work - Traditional methods will usually work, but with skewed distributions they can produce invalid/questionable results - It's usually good to run both types and compare them --- class: center, middle # Work on [Potential Problems for Quiz #4](https://docs.google.com/a/pacificu.edu/document/d/14XJgCJOt-F1LAxmG2PPeJJ0G5nzSWJ4ead-V76EvoNw/edit?usp=sharing) - Quiz 4 is only over Chapters 6 and 7 of ModernDive --- layout: true .footer[[Week 12 - Thursday](https://ismayc.github.io/soc301_s2017/2017/04/20/week-12---thursday/)] --- name: week12th class: center, middle # Week 12 - Thursday # April 20, 2017 --- # Warmup - Give the definition of _p_-value - Summarize the lecture from Tuesday in 3-5 sentences --- class: center, middle # [Adjusted class schedule](https://ismayc.github.io/soc301_s2017/schedule/) --- ## There is Only One Test <img src="figure/ht.png" alt="Drawing" style="width: 800px;"/> Image source [here](http://allendowney.blogspot.com/2016/06/there-is-still-only-one-test.html) --- ## Hypothesis Testing in General ### The **how** of these 3 points may change, but the **what** doesn't: 1. Identify the test statistic -- 2. Construct the null distribution of the test statistic based on the null hypothesis -- 3. Compare the observed test statistic to the null distribution to compute the **p-value** --- ## Specifics 1: Test Statistic We can do hypothesis testing for lots of different scenarios Type | Population Parameter | Test Statistic ------------- | ------------- | ------------- One-Sample | Mean <br> `\(\mu\)` | Sample Mean <br> `\(\overline{x}\)` One-Sample | Proportion <br> `\(\pi\)` | Sample Proportion <br> `\(\widehat{p}\)` Two-Sample (Independent) | Diff of Population Means <br> `\(\mu_1 - \mu_2\)` | Diff of Sample Means <br> `\(\overline{x}_1 - \overline{x}_2\)` Two-Sample (Paired) | Mean Population Difference <br> `\(\mu_{diff}\)` | Mean Sample Difference <br> `\(\overline{x}_{diff}\)` Two-Sample | Diff of Population Proportions <br> `\(\pi_1 - \pi_2\)` | Diff of Sample Proportions <br> `\(\widehat{p}_1 - \widehat{p}_2\)` We'll discuss more advanced procedures (ANOVA and Chi-Square) on Tuesday. --- ## Specifics 2: Null Distribution ### We can construct the null distribution of the test statistic either * Via computation i.e. simulation/shuffling - Ex: We used the **Permutation Test** i.e. we permuted things * Via mathematics i.e. analytically - Ex: Traditionally via Probability using the **Central Limit Theorem**: `\(\overline{X}\)` is Normally distributed as `\(n \rightarrow \infty\)` - **Note**: the null distribution isn't always bell shaped! i.e. not always Normal --- ## Specifics 3: _p_-value ### For Two-Sample Independent tests, we have either ### Two-sided _p_-values + Two-tailed: `\(H_A: \mu_{1} - \mu_{2} \neq 0\)` -- ### One-sided _p_-values -- + Left-tailed: `\(H_A: \mu_{1} - \mu_{2} < 0\)` -- + Right-tailed: `\(H_A: \mu_{1} - \mu_{2} > 0\)` --- ## Moral of the Story ### If you forget what hypothesis testing and/or _p_-values are remember: 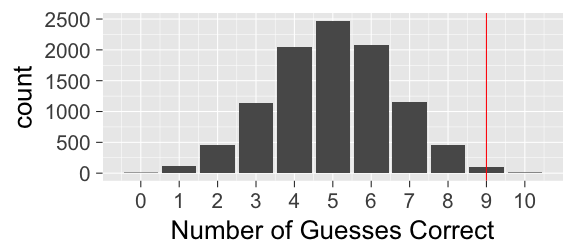<!-- --> - For this example (from this plot), since our observed results are far from what we expect, we reject our original "by chance" assumption in favor of the research hypothesis. --- # Split into groups of 3-4 ### For each of the following five problems BEFORE trying to identify which type of problem it is, identify - the variables of interest, - the types of variables they are, - and the observational units ### AFTER identifying the problem type for each of the five problems, start to layout what the null and alternative hypotheses are in symbols. --- ## Identify the type of problem: ### **Types**: One Mean, One Proportion, Two Means (Independent), Two Means (Paired), Two Proportions ### **Problem 1**: Average income varies from one region of the country to another, and it often reflects both lifestyles and regional living expenses. Suppose a new graduate is considering a job in two locations, Cleveland, OH and Sacramento, CA, and he wants to see whether the average income in one of these cities is higher than the other. He would like to conduct a hypothesis test based on two randomly selected samples from the 2000 Census. --- ## Identify the type of problem: ### **Types**: One Mean, One Proportion, Two Means (Independent), Two Means (Paired), Two Proportions ### **Problem 2**: A 2010 survey asked 827 randomly sampled registered voters in California "Do you support? Or do you oppose? Drilling for oil and natural gas off the Coast of California? Or do you not know enough to say?" Conduct a hypothesis test to determine if the data provide strong evidence that the proportion of college graduates who do not have an opinion on this issue is different than that of non-college graduates. --- ## Identify the type of problem: ### **Types**: One Mean, One Proportion, Two Means (Independent), Two Means (Paired), Two Proportions ### **Problem 3**: The CEO of a large electric utility claims that 80 percent of his 1,000,000 customers are satisfied with the service they receive. To test this claim, the local newspaper surveyed 100 customers, using simple random sampling. 73 were satisfied and the remaining were unsatisfied. Based on these findings from the sample, can we reject the CEO's hypothesis that 80% of the customers are satisfied? --- ## Identify the type of problem: ### **Types**: One Mean, One Proportion, Two Means (Independent), Two Means (Paired), Two Proportions ### **Problem 4**: The National Survey of Family Growth conducted by the Centers for Disease Control gathers information on family life, marriage and divorce, pregnancy, infertility, use of contraception, and men's and women's health. One of the variables collected on this survey is the age at first marriage. 5,534 randomly sampled US women between 2006 and 2010 completed the survey. The women sampled here had been married at least once. Do we have evidence that the mean age of first marriage for all US women from 2006 to 2010 is greater than 23 years? --- ## Identify the type of problem: ### **Types**: One Mean, One Proportion, Two Means (Independent), Two Means (Paired), Two Proportions ### **Problem 5**: Trace metals in drinking water affect the flavor and an unusually high concentration can pose a health hazard. Ten pairs of data were taken measuring zinc concentration in bottom water and surface water at 10 randomly selected locations on a stretch of river. Do the data suggest that the true average concentration in the surface water is smaller than that of bottom water? (Note that units are not given.) --- ## To do for next time (individually) - Read [this article](http://fivethirtyeight.com/features/you-cant-trust-what-you-read-about-nutrition/) about _p_-hacking from 538 - Summarize the main findings from the article and how it relates to the content in Chapter 7 of ModernDive in 3-5 paragraphs - Create AT LEAST five multiple choice questions covering Chapters 6 and 7 of [ModernDive](http://moderndive.com). More directions [here](https://ismayc.github.io/soc301_s2017/problem-sets/index.html#ps11). --- layout: true .footer[[Week 12 - Tuesday](https://ismayc.github.io/soc301_s2017/2017/04/18/week-12---tuesday/)] --- name: week12t class: center, middle # Week 12 - Tuesday # April 18, 2017 --- class: center, middle # WARMUP <!-- Summarize Sections 7.1 - 7.5 in your own words in 4-5 sentences --> --- ## Review Problem Set 10 from last time in pairs - If not listed, review [Problem Set 10 Discussion](https://ismayc.github.io/soc301_s2017/problem-sets/ps-key/index.html#ps10-key) *** <br> .left-column[ - Jayla, Jeremy - Rachel, Alicia F. - Ariel, Alicia O. - Alexandra, Tia - Hannah, Kelsey ] .right-column[ - Emily, Natasha - Fernando, Karla - Garrett, Daisy - Lina, Bree - Taylor, Cydni ] --- ## County Characteristics Datasets ### Annual County Resident Population Estimates by Age, Sex, Race, and Hispanic Origin - Downloaded from [here](https://www2.census.gov/programs-surveys/popest/datasets/2010-2015/counties/asrh/) via <br> <small> `cc-est2015-alldata.csv` </small> - Codebook is available [here](https://www2.census.gov/programs-surveys/popest/datasets/2010-2015/counties/asrh/cc-est2015-alldata.pdf) - This dataset is NOT **tidy**. I've tidied it and focused on only Total Population and Black or African American alone. ```r # Remember to load the needed packages library(dplyr) library(mosaic) library(ggplot2) library(readr) ``` --- ## Hypothesis Testing ### Comparing mean percentages in two states - Suppose we were interested in testing the mean percentage of black residents in two different states. Let's choose Oregon and Washington. - Washington has 39 counties and Oregon has 36. - Assume we only have access to 20 randomly selected counties from each state. ```r url <- "http://ismayc.github.io/wash-or_black.csv" wash_or <- readr::read_csv(url) ``` --- ## Problem set-up ### Do we have evidence that the mean percentage of black residents in Washington and Oregon is statistically different? - What's the alternative hypothesis? -- - <small> `\(H_A: \mu_{wa} \ne \mu_{or}\)` or `\(H_A: \mu_{wa} - \mu_{or} \ne 0\)` </small> - What is the null hypothesis? -- - <small> `\(H_0: \mu_{wa} = \mu_{or}\)` or `\(H_0: \mu_{wa} - \mu_{or} = 0\)` </small> --- ## The Hypotheses - `\(H_0: \mu_{wa} - \mu_{or} = 0\)` - `\(H_A: \mu_{wa} - \mu_{or} \ne 0\)` ### What do these mean in laymen terms? -- - We are assuming that the mean percentage of black residents in Washington and Oregon is THE SAME. - We will use hypothesis testing to look to see if we have evidence to go against this assumption in favor of the alternative hypothesis. --- class: center, middle ## The layout of all hypothesis tests <img src="figure/ht.png" width="895" /> --- ## For our problem ### What is the *observational unit*? <table> <thead> <tr> <th style="text-align:left;"> county </th> <th style="text-align:left;"> state </th> <th style="text-align:right;"> black_perc </th> </tr> </thead> <tbody> <tr> <td style="text-align:left;"> Polk County </td> <td style="text-align:left;"> Oregon </td> <td style="text-align:right;"> 0.8817120 </td> </tr> <tr> <td style="text-align:left;"> Lake County </td> <td style="text-align:left;"> Oregon </td> <td style="text-align:right;"> 0.7025163 </td> </tr> <tr> <td style="text-align:left;"> Lane County </td> <td style="text-align:left;"> Oregon </td> <td style="text-align:right;"> 1.1113407 </td> </tr> <tr> <td style="text-align:left;"> Clallam County </td> <td style="text-align:left;"> Washington </td> <td style="text-align:right;"> 1.0287674 </td> </tr> <tr> <td style="text-align:left;"> Pierce County </td> <td style="text-align:left;"> Washington </td> <td style="text-align:right;"> 7.4209021 </td> </tr> </tbody> </table> -- <br> - Counties in Washington and Oregon --- ## DATA: - We have collected our sample of 20 Washington counties and 20 Oregon counties -- ## TEST STATISTIC: - We are interested in the difference in sample means. --- ## OBSERVED EFFECT ```r (state_means <- wash_or %>% group_by(state) %>% summarize(mean_perc = mean(black_perc))) ``` ``` # A tibble: 2 × 2 state mean_perc <chr> <dbl> 1 Oregon 1.038969 2 Washington 1.553448 ``` ```r (obs_diff <- diff(state_means$mean_perc)) ``` ``` [1] 0.5144789 ``` - From our original (observed) sample, we have ### `$$\verb+obs_diff+ = \bar{x}_{wa} - \bar{x}_{or} = 0.5144789$$` --- ## MODEL OF <small> `\(H_0\)` </small> ### We assume that the two POPULATION mean percentages are the same: - `\(H_0: \mu_{wa} = \mu_{or}\)` <br> or <br> `\(H_0: \mu_{wa} - \mu_{or} = 0\)` - EXTREMELY IMPORTANT NOTE: Remember that PARAMETERS go in hypotheses NOT statistics! --- ## SIMULATED DATA ### We want to use the data we have collected to simulate a process following the model for `\(H_0\)`. -- ### *BIG STEP* : Assuming that <small> `\(H_0: \mu_{wa} = \mu_{or}\)` </small>, any one of the percentages we saw in our sample data could have occurred (equally) in either Washington or in Oregon. -- ### We can use the `shuffle` function in the `mosaic` package to shuffle the percentages between Oregon and Washington. --- ## One shuffle ```r set.seed(2016) (shuffled_state_means <- wash_or %>% mutate(black_perc = shuffle(black_perc)) %>% group_by(state) %>% summarize(mean_shuf_perc = mean(black_perc))) ``` ``` # A tibble: 2 × 2 state mean_shuf_perc <chr> <dbl> 1 Oregon 1.424482 2 Washington 1.167934 ``` ```r (obs_shuf_diff <- diff(shuffled_state_means$mean_shuf_perc)) ``` ``` [1] -0.2565485 ``` --- ## NULL DISTRIBUTION - Repeat this shuffling many times and then look at the distribution of all the resulting simulated test statistics ```r many_shuffles <- do(5000) * (shuffled_state_means <- wash_or %>% mutate(black_perc = shuffle(black_perc)) %>% group_by(state) %>% summarize(mean_shuf_perc = mean(black_perc))) rand_distn <- many_shuffles %>% group_by(.index) %>% summarize(diffmean = diff(mean_shuf_perc)) ``` --- ### Plot of NULL DISTRIBUTION ```r ggplot(rand_distn, aes(x = diffmean)) + geom_histogram(color = "white", bins = 20) ``` 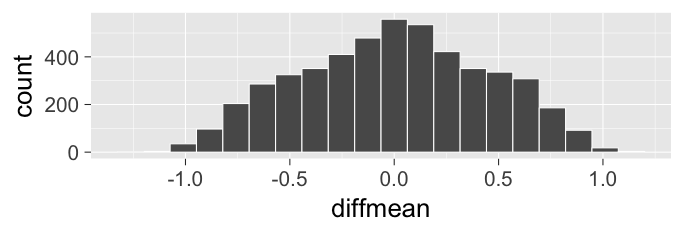<!-- --> - Where will the center of this distribution always be for tests of this sort? -- - 0 (We assumed the means were the same & used that process to build the distribution). --- ## View the _p_-value ### We want to see where our observed effect (the original different in sample means) falls on our NULL DISTRIBUTION. - Note that we check for both directions here since we had a two-sided <small> `\(H_A\)`. </small> ```r ggplot(rand_distn, aes(x = diffmean, fill = (abs(diffmean) >= obs_diff))) + geom_histogram(color = "white", bins = 20) ``` 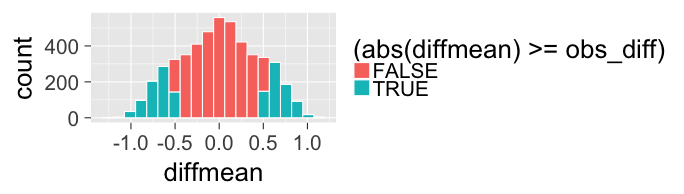<!-- --> --- ## View the `\(p\)`-value ```r ggplot(data = rand_distn, aes(x = diffmean)) + geom_histogram(color = "white", bins = 20) + geom_vline(xintercept = obs_diff, color = "red") + geom_vline(xintercept = -obs_diff, color = "red") ``` 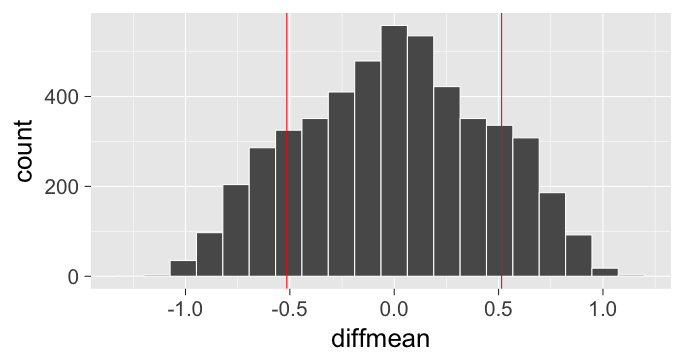<!-- --> --- ## Calculate the _p_-value ```r pvalue <- rand_distn %>% filter(abs(diffmean) >= obs_diff) %>% nrow() / nrow(rand_distn) pvalue ``` ``` [1] 0.305 ``` ### So there is around a 30% chance we could have seen results such as this (or MORE EXTREME) if the true population mean percentages were the same. What does that mean? --- ## Significance level - The "beyond a reasonable doubt" in statistics corresponds to setting the `\(\alpha\)` value before you conduct your test. Most often this is set to 5%. - So with our test, since 0.305 > 0.05, we fail to reject <small> `\(H_0\)` </small> in favor of <small> `\(H_A\)` </small>. - We DO NOT have evidence to support the claim that the mean percentages of black residents per county are different for Washington and Oregon. --- ## All data - The 2015 data for ALL counties is available via: ```r cc_full <- read_csv("http://ismayc.github.io/cc_full.csv") ``` - Practice: Determine what the actual difference is in mean percentage of black residents for ALL Washington and Oregon counties using code similar to what we did earlier. - Is the difference **practically** large? --- # Problem Set 11 - Due at the beginning of class this Thursday - Turn in individually [prefer pencil-and-paper] (You can work with your peers but you'll get more learning if you compare answers AFTER you have tried them on your own.) *** - Do problems LC7.1 - LC7.5 - Read the two articles from 538 - [Statisticians Found One Thing They Can Agree On: It’s Time To Stop Misusing P-Values](http://fivethirtyeight.com/features/statisticians-found-one-thing-they-can-agree-on-its-time-to-stop-misusing-p-values/) - [Science Isn't Broken](https://fivethirtyeight.com/features/science-isnt-broken/) - Answer the questions corresponding [here](https://ismayc.github.io/soc301_s2017/problem-sets/index.html#ps11) --- layout: true .footer[[Week 11 - Thursday](https://ismayc.github.io/soc301_s2017/2017/04/13/week-11---thursday/)] --- name: week11th class: center, middle # Week 11 - Thursday # April 13, 2017 --- # Review Midterm Exam - Here are the [solutions](Midterm_ExamKey.pdf) --- # RStudio Tools ### All hail the <small>`mosaic`</small> package. Load as usual: <small>`library(mosaic)`</small> ### The following four functions will give us (most of) the random simulation tools we need: 1. `rflip()`: Flip a coin 1. `shuffle()`: Shuffle a set of values (akin to cards) 1. `do()`: Do the same thing many, many, many times 1. `resample()`: the swiss army knife of functions --- ## R Simulations Example ### First load up RStudio - Create new R script file, type the following commands (not the comments), and run them in your R Console ```r # Load packages, including mosaic package library(dplyr) library(ggplot2) library(mosaic) # Flip a coin once. Try this multiple times: rflip() ``` ``` Flipping 1 coin [ Prob(Heads) = 0.5 ] ... T Number of Heads: 0 [Proportion Heads: 0] ``` --- ## R Simulations Example ```r # Flip a coin 10 times. Try this multiple times: rflip(10) ``` ``` Flipping 10 coins [ Prob(Heads) = 0.5 ] ... T H T T H H T T H H Number of Heads: 5 [Proportion Heads: 0.5] ``` --- ## R Simulations Example ```r # Flip a coin 10 times, but do this 5 times. Try this multiple times do(5) * rflip(10) ``` ``` n heads tails prop 1 10 5 5 0.5 2 10 5 5 0.5 3 10 5 5 0.5 4 10 4 6 0.4 5 10 7 3 0.7 ``` --- ## R Simulations Example ```r # Flip a coin 10 times, but do this 5000 times do(5000) * rflip(10) ``` ``` n heads tails prop 1 10 9 1 0.9 2 10 5 5 0.5 3 10 5 5 0.5 4 10 5 5 0.5 5 10 3 7 0.3 6 10 6 4 0.6 7 10 4 6 0.4 8 10 7 3 0.7 9 10 6 4 0.6 10 10 7 3 0.7 11 10 4 6 0.4 12 10 6 4 0.6 13 10 7 3 0.7 14 10 6 4 0.6 15 10 7 3 0.7 16 10 5 5 0.5 17 10 4 6 0.4 18 10 6 4 0.6 19 10 4 6 0.4 20 10 5 5 0.5 21 10 4 6 0.4 22 10 5 5 0.5 23 10 3 7 0.3 24 10 5 5 0.5 25 10 4 6 0.4 26 10 6 4 0.6 27 10 5 5 0.5 28 10 4 6 0.4 29 10 7 3 0.7 30 10 5 5 0.5 31 10 4 6 0.4 32 10 7 3 0.7 33 10 5 5 0.5 34 10 4 6 0.4 35 10 7 3 0.7 36 10 6 4 0.6 37 10 6 4 0.6 38 10 3 7 0.3 39 10 5 5 0.5 40 10 5 5 0.5 41 10 9 1 0.9 42 10 5 5 0.5 43 10 5 5 0.5 44 10 2 8 0.2 45 10 5 5 0.5 46 10 6 4 0.6 47 10 6 4 0.6 48 10 6 4 0.6 49 10 5 5 0.5 50 10 7 3 0.7 51 10 8 2 0.8 52 10 3 7 0.3 53 10 5 5 0.5 54 10 7 3 0.7 55 10 5 5 0.5 56 10 5 5 0.5 57 10 7 3 0.7 58 10 5 5 0.5 59 10 5 5 0.5 60 10 6 4 0.6 61 10 5 5 0.5 62 10 3 7 0.3 63 10 4 6 0.4 64 10 7 3 0.7 65 10 5 5 0.5 66 10 6 4 0.6 67 10 5 5 0.5 68 10 6 4 0.6 69 10 6 4 0.6 70 10 4 6 0.4 71 10 4 6 0.4 72 10 5 5 0.5 73 10 7 3 0.7 74 10 3 7 0.3 75 10 5 5 0.5 76 10 4 6 0.4 77 10 5 5 0.5 78 10 6 4 0.6 79 10 4 6 0.4 80 10 5 5 0.5 81 10 7 3 0.7 82 10 7 3 0.7 83 10 2 8 0.2 84 10 6 4 0.6 85 10 2 8 0.2 86 10 6 4 0.6 87 10 6 4 0.6 88 10 7 3 0.7 89 10 5 5 0.5 90 10 2 8 0.2 91 10 4 6 0.4 92 10 5 5 0.5 93 10 5 5 0.5 94 10 8 2 0.8 95 10 5 5 0.5 96 10 4 6 0.4 97 10 6 4 0.6 98 10 4 6 0.4 99 10 6 4 0.6 100 10 5 5 0.5 101 10 5 5 0.5 102 10 5 5 0.5 103 10 5 5 0.5 104 10 4 6 0.4 105 10 4 6 0.4 106 10 1 9 0.1 107 10 6 4 0.6 108 10 6 4 0.6 109 10 8 2 0.8 110 10 3 7 0.3 111 10 6 4 0.6 112 10 6 4 0.6 113 10 3 7 0.3 114 10 6 4 0.6 115 10 5 5 0.5 116 10 6 4 0.6 117 10 7 3 0.7 118 10 4 6 0.4 119 10 2 8 0.2 120 10 8 2 0.8 121 10 8 2 0.8 122 10 7 3 0.7 123 10 3 7 0.3 124 10 6 4 0.6 125 10 2 8 0.2 126 10 6 4 0.6 127 10 6 4 0.6 128 10 3 7 0.3 129 10 5 5 0.5 130 10 5 5 0.5 131 10 5 5 0.5 132 10 5 5 0.5 133 10 8 2 0.8 134 10 7 3 0.7 135 10 8 2 0.8 136 10 3 7 0.3 137 10 6 4 0.6 138 10 2 8 0.2 139 10 8 2 0.8 140 10 5 5 0.5 141 10 8 2 0.8 142 10 5 5 0.5 143 10 5 5 0.5 144 10 6 4 0.6 145 10 2 8 0.2 146 10 4 6 0.4 147 10 5 5 0.5 148 10 2 8 0.2 149 10 7 3 0.7 150 10 4 6 0.4 151 10 4 6 0.4 152 10 7 3 0.7 153 10 3 7 0.3 154 10 4 6 0.4 155 10 6 4 0.6 156 10 3 7 0.3 157 10 5 5 0.5 158 10 7 3 0.7 159 10 7 3 0.7 160 10 6 4 0.6 161 10 3 7 0.3 162 10 4 6 0.4 163 10 5 5 0.5 164 10 7 3 0.7 165 10 7 3 0.7 166 10 7 3 0.7 167 10 5 5 0.5 168 10 7 3 0.7 169 10 7 3 0.7 170 10 3 7 0.3 171 10 4 6 0.4 172 10 6 4 0.6 173 10 7 3 0.7 174 10 8 2 0.8 175 10 4 6 0.4 176 10 5 5 0.5 177 10 5 5 0.5 178 10 5 5 0.5 179 10 7 3 0.7 180 10 6 4 0.6 181 10 4 6 0.4 182 10 5 5 0.5 183 10 4 6 0.4 184 10 4 6 0.4 185 10 4 6 0.4 186 10 7 3 0.7 187 10 4 6 0.4 188 10 3 7 0.3 189 10 3 7 0.3 190 10 7 3 0.7 191 10 2 8 0.2 192 10 6 4 0.6 193 10 2 8 0.2 194 10 6 4 0.6 195 10 8 2 0.8 196 10 5 5 0.5 197 10 9 1 0.9 198 10 5 5 0.5 199 10 5 5 0.5 200 10 5 5 0.5 201 10 5 5 0.5 202 10 4 6 0.4 203 10 3 7 0.3 204 10 4 6 0.4 205 10 3 7 0.3 206 10 6 4 0.6 207 10 5 5 0.5 208 10 5 5 0.5 209 10 7 3 0.7 210 10 4 6 0.4 211 10 4 6 0.4 212 10 8 2 0.8 213 10 4 6 0.4 214 10 4 6 0.4 215 10 2 8 0.2 216 10 6 4 0.6 217 10 5 5 0.5 218 10 4 6 0.4 219 10 3 7 0.3 220 10 3 7 0.3 221 10 3 7 0.3 222 10 5 5 0.5 223 10 5 5 0.5 224 10 3 7 0.3 225 10 5 5 0.5 226 10 6 4 0.6 227 10 4 6 0.4 228 10 3 7 0.3 229 10 4 6 0.4 230 10 5 5 0.5 231 10 5 5 0.5 232 10 5 5 0.5 233 10 8 2 0.8 234 10 6 4 0.6 235 10 6 4 0.6 236 10 4 6 0.4 237 10 5 5 0.5 238 10 6 4 0.6 239 10 1 9 0.1 240 10 5 5 0.5 241 10 6 4 0.6 242 10 5 5 0.5 243 10 6 4 0.6 244 10 8 2 0.8 245 10 3 7 0.3 246 10 8 2 0.8 247 10 3 7 0.3 248 10 2 8 0.2 249 10 3 7 0.3 250 10 6 4 0.6 251 10 5 5 0.5 252 10 4 6 0.4 253 10 7 3 0.7 254 10 4 6 0.4 255 10 8 2 0.8 256 10 6 4 0.6 257 10 7 3 0.7 258 10 5 5 0.5 259 10 8 2 0.8 260 10 7 3 0.7 261 10 3 7 0.3 262 10 6 4 0.6 263 10 2 8 0.2 264 10 3 7 0.3 265 10 5 5 0.5 266 10 6 4 0.6 267 10 8 2 0.8 268 10 1 9 0.1 269 10 5 5 0.5 270 10 7 3 0.7 271 10 5 5 0.5 272 10 6 4 0.6 273 10 7 3 0.7 274 10 6 4 0.6 275 10 6 4 0.6 276 10 2 8 0.2 277 10 4 6 0.4 278 10 4 6 0.4 279 10 4 6 0.4 280 10 6 4 0.6 281 10 6 4 0.6 282 10 4 6 0.4 283 10 4 6 0.4 284 10 5 5 0.5 285 10 6 4 0.6 286 10 4 6 0.4 287 10 6 4 0.6 288 10 3 7 0.3 289 10 5 5 0.5 290 10 6 4 0.6 291 10 6 4 0.6 292 10 8 2 0.8 293 10 5 5 0.5 294 10 5 5 0.5 295 10 6 4 0.6 296 10 6 4 0.6 297 10 6 4 0.6 298 10 5 5 0.5 299 10 8 2 0.8 300 10 4 6 0.4 301 10 6 4 0.6 302 10 1 9 0.1 303 10 4 6 0.4 304 10 5 5 0.5 305 10 5 5 0.5 306 10 7 3 0.7 307 10 6 4 0.6 308 10 3 7 0.3 309 10 8 2 0.8 310 10 6 4 0.6 311 10 5 5 0.5 312 10 6 4 0.6 313 10 6 4 0.6 314 10 2 8 0.2 315 10 4 6 0.4 316 10 5 5 0.5 317 10 3 7 0.3 318 10 4 6 0.4 319 10 4 6 0.4 320 10 6 4 0.6 321 10 3 7 0.3 322 10 5 5 0.5 323 10 6 4 0.6 324 10 7 3 0.7 325 10 2 8 0.2 326 10 6 4 0.6 327 10 5 5 0.5 328 10 6 4 0.6 329 10 7 3 0.7 330 10 5 5 0.5 331 10 5 5 0.5 332 10 5 5 0.5 333 10 4 6 0.4 334 10 3 7 0.3 335 10 4 6 0.4 336 10 2 8 0.2 337 10 6 4 0.6 338 10 5 5 0.5 339 10 5 5 0.5 340 10 5 5 0.5 341 10 5 5 0.5 342 10 3 7 0.3 343 10 5 5 0.5 344 10 3 7 0.3 345 10 8 2 0.8 346 10 4 6 0.4 347 10 8 2 0.8 348 10 6 4 0.6 349 10 4 6 0.4 350 10 8 2 0.8 351 10 4 6 0.4 352 10 3 7 0.3 353 10 6 4 0.6 354 10 4 6 0.4 355 10 9 1 0.9 356 10 5 5 0.5 357 10 6 4 0.6 358 10 3 7 0.3 359 10 6 4 0.6 360 10 2 8 0.2 361 10 5 5 0.5 362 10 5 5 0.5 363 10 7 3 0.7 364 10 3 7 0.3 365 10 6 4 0.6 366 10 7 3 0.7 367 10 2 8 0.2 368 10 6 4 0.6 369 10 3 7 0.3 370 10 4 6 0.4 371 10 7 3 0.7 372 10 5 5 0.5 373 10 5 5 0.5 374 10 7 3 0.7 375 10 6 4 0.6 376 10 3 7 0.3 377 10 5 5 0.5 378 10 7 3 0.7 379 10 4 6 0.4 380 10 5 5 0.5 381 10 5 5 0.5 382 10 6 4 0.6 383 10 6 4 0.6 384 10 3 7 0.3 385 10 5 5 0.5 386 10 7 3 0.7 387 10 8 2 0.8 388 10 7 3 0.7 389 10 5 5 0.5 390 10 6 4 0.6 391 10 7 3 0.7 392 10 3 7 0.3 393 10 3 7 0.3 394 10 7 3 0.7 395 10 4 6 0.4 396 10 6 4 0.6 397 10 5 5 0.5 398 10 4 6 0.4 399 10 7 3 0.7 400 10 2 8 0.2 401 10 3 7 0.3 402 10 4 6 0.4 403 10 6 4 0.6 404 10 5 5 0.5 405 10 6 4 0.6 406 10 6 4 0.6 407 10 5 5 0.5 408 10 3 7 0.3 409 10 4 6 0.4 410 10 6 4 0.6 411 10 7 3 0.7 412 10 2 8 0.2 413 10 4 6 0.4 414 10 4 6 0.4 415 10 3 7 0.3 416 10 5 5 0.5 417 10 6 4 0.6 418 10 6 4 0.6 419 10 5 5 0.5 420 10 2 8 0.2 421 10 6 4 0.6 422 10 6 4 0.6 423 10 6 4 0.6 424 10 5 5 0.5 425 10 4 6 0.4 426 10 8 2 0.8 427 10 6 4 0.6 428 10 4 6 0.4 429 10 6 4 0.6 430 10 5 5 0.5 431 10 7 3 0.7 432 10 6 4 0.6 433 10 2 8 0.2 434 10 6 4 0.6 435 10 9 1 0.9 436 10 5 5 0.5 437 10 7 3 0.7 438 10 4 6 0.4 439 10 4 6 0.4 440 10 5 5 0.5 441 10 5 5 0.5 442 10 4 6 0.4 443 10 7 3 0.7 444 10 5 5 0.5 445 10 4 6 0.4 446 10 3 7 0.3 447 10 5 5 0.5 448 10 4 6 0.4 449 10 7 3 0.7 450 10 4 6 0.4 451 10 4 6 0.4 452 10 4 6 0.4 453 10 5 5 0.5 454 10 2 8 0.2 455 10 3 7 0.3 456 10 3 7 0.3 457 10 4 6 0.4 458 10 6 4 0.6 459 10 6 4 0.6 460 10 3 7 0.3 461 10 3 7 0.3 462 10 5 5 0.5 463 10 4 6 0.4 464 10 6 4 0.6 465 10 4 6 0.4 466 10 7 3 0.7 467 10 5 5 0.5 468 10 6 4 0.6 469 10 2 8 0.2 470 10 8 2 0.8 471 10 6 4 0.6 472 10 5 5 0.5 473 10 3 7 0.3 474 10 7 3 0.7 475 10 6 4 0.6 476 10 5 5 0.5 477 10 4 6 0.4 478 10 6 4 0.6 479 10 4 6 0.4 480 10 3 7 0.3 481 10 6 4 0.6 482 10 6 4 0.6 483 10 6 4 0.6 484 10 7 3 0.7 485 10 5 5 0.5 486 10 5 5 0.5 487 10 3 7 0.3 488 10 2 8 0.2 489 10 7 3 0.7 490 10 4 6 0.4 491 10 4 6 0.4 492 10 5 5 0.5 493 10 5 5 0.5 494 10 8 2 0.8 495 10 4 6 0.4 496 10 3 7 0.3 497 10 5 5 0.5 498 10 2 8 0.2 499 10 3 7 0.3 500 10 7 3 0.7 501 10 8 2 0.8 502 10 6 4 0.6 503 10 3 7 0.3 504 10 6 4 0.6 505 10 1 9 0.1 506 10 6 4 0.6 507 10 7 3 0.7 508 10 6 4 0.6 509 10 6 4 0.6 510 10 6 4 0.6 511 10 4 6 0.4 512 10 4 6 0.4 513 10 5 5 0.5 514 10 5 5 0.5 515 10 4 6 0.4 516 10 7 3 0.7 517 10 5 5 0.5 518 10 4 6 0.4 519 10 2 8 0.2 520 10 3 7 0.3 521 10 4 6 0.4 522 10 5 5 0.5 523 10 6 4 0.6 524 10 4 6 0.4 525 10 4 6 0.4 526 10 6 4 0.6 527 10 6 4 0.6 528 10 2 8 0.2 529 10 5 5 0.5 530 10 4 6 0.4 531 10 7 3 0.7 532 10 3 7 0.3 533 10 7 3 0.7 534 10 4 6 0.4 535 10 8 2 0.8 536 10 5 5 0.5 537 10 4 6 0.4 538 10 5 5 0.5 539 10 5 5 0.5 540 10 4 6 0.4 541 10 4 6 0.4 542 10 5 5 0.5 543 10 4 6 0.4 544 10 4 6 0.4 545 10 3 7 0.3 546 10 4 6 0.4 547 10 4 6 0.4 548 10 6 4 0.6 549 10 6 4 0.6 550 10 4 6 0.4 551 10 7 3 0.7 552 10 4 6 0.4 553 10 6 4 0.6 554 10 4 6 0.4 555 10 5 5 0.5 556 10 3 7 0.3 557 10 3 7 0.3 558 10 2 8 0.2 559 10 6 4 0.6 560 10 6 4 0.6 561 10 5 5 0.5 562 10 5 5 0.5 563 10 5 5 0.5 564 10 3 7 0.3 565 10 2 8 0.2 566 10 4 6 0.4 567 10 4 6 0.4 568 10 5 5 0.5 569 10 3 7 0.3 570 10 4 6 0.4 571 10 4 6 0.4 572 10 4 6 0.4 573 10 5 5 0.5 574 10 9 1 0.9 575 10 3 7 0.3 576 10 7 3 0.7 577 10 5 5 0.5 578 10 4 6 0.4 579 10 5 5 0.5 580 10 4 6 0.4 581 10 3 7 0.3 582 10 4 6 0.4 583 10 4 6 0.4 584 10 3 7 0.3 585 10 7 3 0.7 586 10 4 6 0.4 587 10 5 5 0.5 588 10 3 7 0.3 589 10 6 4 0.6 590 10 6 4 0.6 591 10 6 4 0.6 592 10 5 5 0.5 593 10 5 5 0.5 594 10 7 3 0.7 595 10 5 5 0.5 596 10 4 6 0.4 597 10 6 4 0.6 598 10 8 2 0.8 599 10 5 5 0.5 600 10 7 3 0.7 601 10 6 4 0.6 602 10 5 5 0.5 603 10 5 5 0.5 604 10 3 7 0.3 605 10 7 3 0.7 606 10 6 4 0.6 607 10 8 2 0.8 608 10 4 6 0.4 609 10 3 7 0.3 610 10 5 5 0.5 611 10 9 1 0.9 612 10 7 3 0.7 613 10 5 5 0.5 614 10 2 8 0.2 615 10 1 9 0.1 616 10 8 2 0.8 617 10 5 5 0.5 618 10 6 4 0.6 619 10 2 8 0.2 620 10 5 5 0.5 621 10 5 5 0.5 622 10 6 4 0.6 623 10 7 3 0.7 624 10 6 4 0.6 625 10 2 8 0.2 626 10 8 2 0.8 627 10 5 5 0.5 628 10 1 9 0.1 629 10 5 5 0.5 630 10 5 5 0.5 631 10 4 6 0.4 632 10 6 4 0.6 633 10 5 5 0.5 634 10 8 2 0.8 635 10 6 4 0.6 636 10 6 4 0.6 637 10 4 6 0.4 638 10 3 7 0.3 639 10 5 5 0.5 640 10 7 3 0.7 641 10 4 6 0.4 642 10 5 5 0.5 643 10 4 6 0.4 644 10 3 7 0.3 645 10 6 4 0.6 646 10 7 3 0.7 647 10 6 4 0.6 648 10 4 6 0.4 649 10 4 6 0.4 650 10 4 6 0.4 651 10 4 6 0.4 652 10 6 4 0.6 653 10 5 5 0.5 654 10 5 5 0.5 655 10 4 6 0.4 656 10 6 4 0.6 657 10 8 2 0.8 658 10 8 2 0.8 659 10 6 4 0.6 660 10 6 4 0.6 661 10 5 5 0.5 662 10 7 3 0.7 663 10 5 5 0.5 664 10 6 4 0.6 665 10 3 7 0.3 666 10 7 3 0.7 667 10 6 4 0.6 668 10 7 3 0.7 669 10 7 3 0.7 670 10 7 3 0.7 671 10 4 6 0.4 672 10 4 6 0.4 673 10 2 8 0.2 674 10 4 6 0.4 675 10 4 6 0.4 676 10 4 6 0.4 677 10 3 7 0.3 678 10 7 3 0.7 679 10 3 7 0.3 680 10 5 5 0.5 681 10 3 7 0.3 682 10 9 1 0.9 683 10 4 6 0.4 684 10 5 5 0.5 685 10 5 5 0.5 686 10 5 5 0.5 687 10 6 4 0.6 688 10 6 4 0.6 689 10 7 3 0.7 690 10 6 4 0.6 691 10 6 4 0.6 692 10 4 6 0.4 693 10 5 5 0.5 694 10 4 6 0.4 695 10 5 5 0.5 696 10 5 5 0.5 697 10 3 7 0.3 698 10 4 6 0.4 699 10 7 3 0.7 700 10 7 3 0.7 701 10 6 4 0.6 702 10 3 7 0.3 703 10 6 4 0.6 704 10 6 4 0.6 705 10 3 7 0.3 706 10 6 4 0.6 707 10 1 9 0.1 708 10 8 2 0.8 709 10 6 4 0.6 710 10 7 3 0.7 711 10 6 4 0.6 712 10 7 3 0.7 713 10 5 5 0.5 714 10 5 5 0.5 715 10 3 7 0.3 716 10 6 4 0.6 717 10 7 3 0.7 718 10 6 4 0.6 719 10 7 3 0.7 720 10 7 3 0.7 721 10 5 5 0.5 722 10 5 5 0.5 723 10 5 5 0.5 724 10 5 5 0.5 725 10 5 5 0.5 726 10 5 5 0.5 727 10 4 6 0.4 728 10 4 6 0.4 729 10 8 2 0.8 730 10 4 6 0.4 731 10 3 7 0.3 732 10 3 7 0.3 733 10 5 5 0.5 734 10 2 8 0.2 735 10 7 3 0.7 736 10 4 6 0.4 737 10 4 6 0.4 738 10 8 2 0.8 739 10 6 4 0.6 740 10 4 6 0.4 741 10 4 6 0.4 742 10 4 6 0.4 743 10 4 6 0.4 744 10 6 4 0.6 745 10 7 3 0.7 746 10 5 5 0.5 747 10 6 4 0.6 748 10 3 7 0.3 749 10 5 5 0.5 750 10 6 4 0.6 751 10 7 3 0.7 752 10 5 5 0.5 753 10 4 6 0.4 754 10 5 5 0.5 755 10 7 3 0.7 756 10 5 5 0.5 757 10 7 3 0.7 758 10 7 3 0.7 759 10 3 7 0.3 760 10 4 6 0.4 761 10 5 5 0.5 762 10 6 4 0.6 763 10 6 4 0.6 764 10 5 5 0.5 765 10 9 1 0.9 766 10 5 5 0.5 767 10 3 7 0.3 768 10 6 4 0.6 769 10 7 3 0.7 770 10 4 6 0.4 771 10 5 5 0.5 772 10 5 5 0.5 773 10 4 6 0.4 774 10 5 5 0.5 775 10 5 5 0.5 776 10 6 4 0.6 777 10 5 5 0.5 778 10 8 2 0.8 779 10 4 6 0.4 780 10 7 3 0.7 781 10 3 7 0.3 782 10 4 6 0.4 783 10 6 4 0.6 784 10 5 5 0.5 785 10 7 3 0.7 786 10 5 5 0.5 787 10 3 7 0.3 788 10 6 4 0.6 789 10 4 6 0.4 790 10 4 6 0.4 791 10 6 4 0.6 792 10 6 4 0.6 793 10 7 3 0.7 794 10 7 3 0.7 795 10 8 2 0.8 796 10 5 5 0.5 797 10 4 6 0.4 798 10 3 7 0.3 799 10 6 4 0.6 800 10 2 8 0.2 801 10 6 4 0.6 802 10 4 6 0.4 803 10 5 5 0.5 804 10 6 4 0.6 805 10 8 2 0.8 806 10 5 5 0.5 807 10 5 5 0.5 808 10 4 6 0.4 809 10 5 5 0.5 810 10 3 7 0.3 811 10 4 6 0.4 812 10 4 6 0.4 813 10 5 5 0.5 814 10 5 5 0.5 815 10 3 7 0.3 816 10 7 3 0.7 817 10 5 5 0.5 818 10 4 6 0.4 819 10 4 6 0.4 820 10 5 5 0.5 821 10 3 7 0.3 822 10 3 7 0.3 823 10 6 4 0.6 824 10 5 5 0.5 825 10 3 7 0.3 826 10 7 3 0.7 827 10 5 5 0.5 828 10 5 5 0.5 829 10 5 5 0.5 830 10 5 5 0.5 831 10 5 5 0.5 832 10 3 7 0.3 833 10 4 6 0.4 834 10 6 4 0.6 835 10 4 6 0.4 836 10 6 4 0.6 837 10 4 6 0.4 838 10 6 4 0.6 839 10 5 5 0.5 840 10 6 4 0.6 841 10 5 5 0.5 842 10 6 4 0.6 843 10 6 4 0.6 844 10 6 4 0.6 845 10 5 5 0.5 846 10 6 4 0.6 847 10 5 5 0.5 848 10 5 5 0.5 849 10 6 4 0.6 850 10 6 4 0.6 851 10 5 5 0.5 852 10 5 5 0.5 853 10 3 7 0.3 854 10 5 5 0.5 855 10 6 4 0.6 856 10 6 4 0.6 857 10 3 7 0.3 858 10 4 6 0.4 859 10 5 5 0.5 860 10 7 3 0.7 861 10 7 3 0.7 862 10 5 5 0.5 863 10 8 2 0.8 864 10 8 2 0.8 865 10 5 5 0.5 866 10 7 3 0.7 867 10 5 5 0.5 868 10 3 7 0.3 869 10 4 6 0.4 870 10 5 5 0.5 871 10 7 3 0.7 872 10 4 6 0.4 873 10 4 6 0.4 874 10 7 3 0.7 875 10 6 4 0.6 876 10 5 5 0.5 877 10 6 4 0.6 878 10 4 6 0.4 879 10 4 6 0.4 880 10 6 4 0.6 881 10 5 5 0.5 882 10 2 8 0.2 883 10 5 5 0.5 884 10 6 4 0.6 885 10 4 6 0.4 886 10 5 5 0.5 887 10 4 6 0.4 888 10 5 5 0.5 889 10 3 7 0.3 890 10 3 7 0.3 891 10 7 3 0.7 892 10 4 6 0.4 893 10 4 6 0.4 894 10 7 3 0.7 895 10 6 4 0.6 896 10 6 4 0.6 897 10 3 7 0.3 898 10 6 4 0.6 899 10 2 8 0.2 900 10 5 5 0.5 901 10 5 5 0.5 902 10 3 7 0.3 903 10 6 4 0.6 904 10 7 3 0.7 905 10 6 4 0.6 906 10 4 6 0.4 907 10 7 3 0.7 908 10 4 6 0.4 909 10 5 5 0.5 910 10 5 5 0.5 911 10 6 4 0.6 912 10 6 4 0.6 913 10 6 4 0.6 914 10 5 5 0.5 915 10 7 3 0.7 916 10 5 5 0.5 917 10 6 4 0.6 918 10 6 4 0.6 919 10 4 6 0.4 920 10 7 3 0.7 921 10 4 6 0.4 922 10 8 2 0.8 923 10 4 6 0.4 924 10 3 7 0.3 925 10 6 4 0.6 926 10 3 7 0.3 927 10 6 4 0.6 928 10 4 6 0.4 929 10 6 4 0.6 930 10 4 6 0.4 931 10 5 5 0.5 932 10 7 3 0.7 933 10 9 1 0.9 934 10 7 3 0.7 935 10 5 5 0.5 936 10 4 6 0.4 937 10 4 6 0.4 938 10 5 5 0.5 939 10 5 5 0.5 940 10 3 7 0.3 941 10 8 2 0.8 942 10 5 5 0.5 943 10 4 6 0.4 944 10 8 2 0.8 945 10 6 4 0.6 946 10 7 3 0.7 947 10 8 2 0.8 948 10 7 3 0.7 949 10 3 7 0.3 950 10 3 7 0.3 951 10 3 7 0.3 952 10 3 7 0.3 953 10 6 4 0.6 954 10 6 4 0.6 955 10 6 4 0.6 956 10 7 3 0.7 957 10 5 5 0.5 958 10 5 5 0.5 959 10 3 7 0.3 960 10 5 5 0.5 961 10 4 6 0.4 962 10 4 6 0.4 963 10 6 4 0.6 964 10 8 2 0.8 965 10 6 4 0.6 966 10 6 4 0.6 967 10 2 8 0.2 968 10 5 5 0.5 969 10 4 6 0.4 970 10 5 5 0.5 971 10 5 5 0.5 972 10 5 5 0.5 973 10 4 6 0.4 974 10 4 6 0.4 975 10 5 5 0.5 976 10 6 4 0.6 977 10 4 6 0.4 978 10 4 6 0.4 979 10 7 3 0.7 980 10 4 6 0.4 981 10 6 4 0.6 982 10 5 5 0.5 983 10 6 4 0.6 984 10 4 6 0.4 985 10 5 5 0.5 986 10 7 3 0.7 987 10 8 2 0.8 988 10 5 5 0.5 989 10 3 7 0.3 990 10 6 4 0.6 991 10 5 5 0.5 992 10 5 5 0.5 993 10 4 6 0.4 994 10 6 4 0.6 995 10 6 4 0.6 996 10 5 5 0.5 997 10 5 5 0.5 998 10 5 5 0.5 999 10 3 7 0.3 1000 10 5 5 0.5 1001 10 5 5 0.5 1002 10 5 5 0.5 1003 10 4 6 0.4 1004 10 3 7 0.3 1005 10 6 4 0.6 1006 10 5 5 0.5 1007 10 4 6 0.4 1008 10 5 5 0.5 1009 10 7 3 0.7 1010 10 3 7 0.3 1011 10 7 3 0.7 1012 10 6 4 0.6 1013 10 4 6 0.4 1014 10 4 6 0.4 1015 10 4 6 0.4 1016 10 7 3 0.7 1017 10 7 3 0.7 1018 10 2 8 0.2 1019 10 2 8 0.2 1020 10 7 3 0.7 1021 10 5 5 0.5 1022 10 8 2 0.8 1023 10 7 3 0.7 1024 10 5 5 0.5 1025 10 5 5 0.5 1026 10 3 7 0.3 1027 10 4 6 0.4 1028 10 5 5 0.5 1029 10 6 4 0.6 1030 10 4 6 0.4 1031 10 7 3 0.7 1032 10 5 5 0.5 1033 10 4 6 0.4 1034 10 4 6 0.4 1035 10 3 7 0.3 1036 10 7 3 0.7 1037 10 7 3 0.7 1038 10 9 1 0.9 1039 10 4 6 0.4 1040 10 7 3 0.7 1041 10 6 4 0.6 1042 10 2 8 0.2 1043 10 5 5 0.5 1044 10 6 4 0.6 1045 10 5 5 0.5 1046 10 3 7 0.3 1047 10 3 7 0.3 1048 10 4 6 0.4 1049 10 5 5 0.5 1050 10 4 6 0.4 1051 10 7 3 0.7 1052 10 6 4 0.6 1053 10 4 6 0.4 1054 10 8 2 0.8 1055 10 4 6 0.4 1056 10 4 6 0.4 1057 10 7 3 0.7 1058 10 6 4 0.6 1059 10 5 5 0.5 1060 10 4 6 0.4 1061 10 4 6 0.4 1062 10 4 6 0.4 1063 10 3 7 0.3 1064 10 3 7 0.3 1065 10 3 7 0.3 1066 10 5 5 0.5 1067 10 4 6 0.4 1068 10 4 6 0.4 1069 10 6 4 0.6 1070 10 4 6 0.4 1071 10 5 5 0.5 1072 10 7 3 0.7 1073 10 5 5 0.5 1074 10 6 4 0.6 1075 10 8 2 0.8 1076 10 6 4 0.6 1077 10 7 3 0.7 1078 10 4 6 0.4 1079 10 3 7 0.3 1080 10 4 6 0.4 1081 10 7 3 0.7 1082 10 8 2 0.8 1083 10 5 5 0.5 1084 10 4 6 0.4 1085 10 4 6 0.4 1086 10 6 4 0.6 1087 10 6 4 0.6 1088 10 2 8 0.2 1089 10 4 6 0.4 1090 10 4 6 0.4 1091 10 4 6 0.4 1092 10 5 5 0.5 1093 10 5 5 0.5 1094 10 5 5 0.5 1095 10 2 8 0.2 1096 10 4 6 0.4 1097 10 3 7 0.3 1098 10 5 5 0.5 1099 10 5 5 0.5 1100 10 8 2 0.8 1101 10 4 6 0.4 1102 10 6 4 0.6 1103 10 5 5 0.5 1104 10 5 5 0.5 1105 10 6 4 0.6 1106 10 6 4 0.6 1107 10 5 5 0.5 1108 10 4 6 0.4 1109 10 4 6 0.4 1110 10 2 8 0.2 1111 10 10 0 1.0 1112 10 4 6 0.4 1113 10 5 5 0.5 1114 10 6 4 0.6 1115 10 6 4 0.6 1116 10 6 4 0.6 1117 10 6 4 0.6 1118 10 5 5 0.5 1119 10 2 8 0.2 1120 10 2 8 0.2 1121 10 5 5 0.5 1122 10 7 3 0.7 1123 10 8 2 0.8 1124 10 3 7 0.3 1125 10 3 7 0.3 1126 10 4 6 0.4 1127 10 7 3 0.7 1128 10 5 5 0.5 1129 10 4 6 0.4 1130 10 5 5 0.5 1131 10 4 6 0.4 1132 10 5 5 0.5 1133 10 4 6 0.4 1134 10 8 2 0.8 1135 10 6 4 0.6 1136 10 7 3 0.7 1137 10 5 5 0.5 1138 10 6 4 0.6 1139 10 6 4 0.6 1140 10 5 5 0.5 1141 10 8 2 0.8 1142 10 5 5 0.5 1143 10 4 6 0.4 1144 10 4 6 0.4 1145 10 6 4 0.6 1146 10 5 5 0.5 1147 10 4 6 0.4 1148 10 6 4 0.6 1149 10 3 7 0.3 1150 10 7 3 0.7 1151 10 6 4 0.6 1152 10 6 4 0.6 1153 10 6 4 0.6 1154 10 4 6 0.4 1155 10 5 5 0.5 1156 10 6 4 0.6 1157 10 5 5 0.5 1158 10 8 2 0.8 1159 10 6 4 0.6 1160 10 7 3 0.7 1161 10 6 4 0.6 1162 10 8 2 0.8 1163 10 7 3 0.7 1164 10 5 5 0.5 1165 10 4 6 0.4 1166 10 7 3 0.7 1167 10 6 4 0.6 1168 10 6 4 0.6 1169 10 7 3 0.7 1170 10 4 6 0.4 1171 10 5 5 0.5 1172 10 7 3 0.7 1173 10 9 1 0.9 1174 10 7 3 0.7 1175 10 6 4 0.6 1176 10 7 3 0.7 1177 10 6 4 0.6 1178 10 6 4 0.6 1179 10 7 3 0.7 1180 10 3 7 0.3 1181 10 5 5 0.5 1182 10 4 6 0.4 1183 10 5 5 0.5 1184 10 5 5 0.5 1185 10 9 1 0.9 1186 10 6 4 0.6 1187 10 5 5 0.5 1188 10 6 4 0.6 1189 10 4 6 0.4 1190 10 5 5 0.5 1191 10 2 8 0.2 1192 10 7 3 0.7 1193 10 5 5 0.5 1194 10 6 4 0.6 1195 10 7 3 0.7 1196 10 3 7 0.3 1197 10 6 4 0.6 1198 10 3 7 0.3 1199 10 4 6 0.4 1200 10 8 2 0.8 1201 10 5 5 0.5 1202 10 6 4 0.6 1203 10 4 6 0.4 1204 10 5 5 0.5 1205 10 5 5 0.5 1206 10 3 7 0.3 1207 10 5 5 0.5 1208 10 3 7 0.3 1209 10 6 4 0.6 1210 10 3 7 0.3 1211 10 5 5 0.5 1212 10 5 5 0.5 1213 10 3 7 0.3 1214 10 6 4 0.6 1215 10 4 6 0.4 1216 10 4 6 0.4 1217 10 9 1 0.9 1218 10 6 4 0.6 1219 10 4 6 0.4 1220 10 5 5 0.5 1221 10 7 3 0.7 1222 10 6 4 0.6 1223 10 4 6 0.4 1224 10 5 5 0.5 1225 10 3 7 0.3 1226 10 5 5 0.5 1227 10 4 6 0.4 1228 10 4 6 0.4 1229 10 4 6 0.4 1230 10 4 6 0.4 1231 10 5 5 0.5 1232 10 1 9 0.1 1233 10 8 2 0.8 1234 10 7 3 0.7 1235 10 7 3 0.7 1236 10 6 4 0.6 1237 10 3 7 0.3 1238 10 8 2 0.8 1239 10 7 3 0.7 1240 10 7 3 0.7 1241 10 7 3 0.7 1242 10 5 5 0.5 1243 10 5 5 0.5 1244 10 4 6 0.4 1245 10 6 4 0.6 1246 10 2 8 0.2 1247 10 6 4 0.6 1248 10 3 7 0.3 1249 10 5 5 0.5 1250 10 5 5 0.5 1251 10 5 5 0.5 1252 10 6 4 0.6 1253 10 4 6 0.4 1254 10 7 3 0.7 1255 10 6 4 0.6 1256 10 6 4 0.6 1257 10 3 7 0.3 1258 10 5 5 0.5 1259 10 3 7 0.3 1260 10 6 4 0.6 1261 10 5 5 0.5 1262 10 4 6 0.4 1263 10 3 7 0.3 1264 10 8 2 0.8 1265 10 8 2 0.8 1266 10 3 7 0.3 1267 10 8 2 0.8 1268 10 3 7 0.3 1269 10 4 6 0.4 1270 10 5 5 0.5 1271 10 4 6 0.4 1272 10 3 7 0.3 1273 10 4 6 0.4 1274 10 2 8 0.2 1275 10 6 4 0.6 1276 10 4 6 0.4 1277 10 3 7 0.3 1278 10 4 6 0.4 1279 10 7 3 0.7 1280 10 5 5 0.5 1281 10 7 3 0.7 1282 10 5 5 0.5 1283 10 5 5 0.5 1284 10 3 7 0.3 1285 10 1 9 0.1 1286 10 5 5 0.5 1287 10 6 4 0.6 1288 10 4 6 0.4 1289 10 6 4 0.6 1290 10 10 0 1.0 1291 10 5 5 0.5 1292 10 7 3 0.7 1293 10 5 5 0.5 1294 10 5 5 0.5 1295 10 4 6 0.4 1296 10 5 5 0.5 1297 10 6 4 0.6 1298 10 6 4 0.6 1299 10 4 6 0.4 1300 10 7 3 0.7 1301 10 4 6 0.4 1302 10 4 6 0.4 1303 10 5 5 0.5 1304 10 5 5 0.5 1305 10 8 2 0.8 1306 10 5 5 0.5 1307 10 7 3 0.7 1308 10 5 5 0.5 1309 10 2 8 0.2 1310 10 3 7 0.3 1311 10 7 3 0.7 1312 10 7 3 0.7 1313 10 4 6 0.4 1314 10 4 6 0.4 1315 10 5 5 0.5 1316 10 4 6 0.4 1317 10 4 6 0.4 1318 10 5 5 0.5 1319 10 3 7 0.3 1320 10 4 6 0.4 1321 10 6 4 0.6 1322 10 6 4 0.6 1323 10 7 3 0.7 1324 10 6 4 0.6 1325 10 6 4 0.6 1326 10 7 3 0.7 1327 10 4 6 0.4 1328 10 6 4 0.6 1329 10 8 2 0.8 1330 10 6 4 0.6 1331 10 5 5 0.5 1332 10 6 4 0.6 1333 10 7 3 0.7 1334 10 2 8 0.2 1335 10 3 7 0.3 1336 10 8 2 0.8 1337 10 3 7 0.3 1338 10 3 7 0.3 1339 10 6 4 0.6 1340 10 6 4 0.6 1341 10 5 5 0.5 1342 10 4 6 0.4 1343 10 7 3 0.7 1344 10 5 5 0.5 1345 10 2 8 0.2 1346 10 4 6 0.4 1347 10 4 6 0.4 1348 10 6 4 0.6 1349 10 7 3 0.7 1350 10 7 3 0.7 1351 10 6 4 0.6 1352 10 7 3 0.7 1353 10 5 5 0.5 1354 10 4 6 0.4 1355 10 8 2 0.8 1356 10 6 4 0.6 1357 10 5 5 0.5 1358 10 6 4 0.6 1359 10 5 5 0.5 1360 10 5 5 0.5 1361 10 4 6 0.4 1362 10 6 4 0.6 1363 10 3 7 0.3 1364 10 4 6 0.4 1365 10 6 4 0.6 1366 10 6 4 0.6 1367 10 3 7 0.3 1368 10 7 3 0.7 1369 10 6 4 0.6 1370 10 2 8 0.2 1371 10 4 6 0.4 1372 10 7 3 0.7 1373 10 5 5 0.5 1374 10 4 6 0.4 1375 10 4 6 0.4 1376 10 6 4 0.6 1377 10 7 3 0.7 1378 10 5 5 0.5 1379 10 5 5 0.5 1380 10 8 2 0.8 1381 10 5 5 0.5 1382 10 6 4 0.6 1383 10 7 3 0.7 1384 10 4 6 0.4 1385 10 4 6 0.4 1386 10 3 7 0.3 1387 10 8 2 0.8 1388 10 7 3 0.7 1389 10 3 7 0.3 1390 10 5 5 0.5 1391 10 5 5 0.5 1392 10 2 8 0.2 1393 10 8 2 0.8 1394 10 8 2 0.8 1395 10 4 6 0.4 1396 10 6 4 0.6 1397 10 5 5 0.5 1398 10 5 5 0.5 1399 10 4 6 0.4 1400 10 6 4 0.6 1401 10 5 5 0.5 1402 10 2 8 0.2 1403 10 5 5 0.5 1404 10 3 7 0.3 1405 10 5 5 0.5 1406 10 3 7 0.3 1407 10 3 7 0.3 1408 10 5 5 0.5 1409 10 5 5 0.5 1410 10 4 6 0.4 1411 10 6 4 0.6 1412 10 4 6 0.4 1413 10 9 1 0.9 1414 10 5 5 0.5 1415 10 8 2 0.8 1416 10 6 4 0.6 1417 10 5 5 0.5 1418 10 6 4 0.6 1419 10 3 7 0.3 1420 10 3 7 0.3 1421 10 5 5 0.5 1422 10 7 3 0.7 1423 10 2 8 0.2 1424 10 6 4 0.6 1425 10 6 4 0.6 1426 10 4 6 0.4 1427 10 6 4 0.6 1428 10 3 7 0.3 1429 10 5 5 0.5 1430 10 7 3 0.7 1431 10 8 2 0.8 1432 10 7 3 0.7 1433 10 5 5 0.5 1434 10 8 2 0.8 1435 10 7 3 0.7 1436 10 4 6 0.4 1437 10 6 4 0.6 1438 10 6 4 0.6 1439 10 4 6 0.4 1440 10 4 6 0.4 1441 10 4 6 0.4 1442 10 4 6 0.4 1443 10 3 7 0.3 1444 10 8 2 0.8 1445 10 5 5 0.5 1446 10 6 4 0.6 1447 10 8 2 0.8 1448 10 6 4 0.6 1449 10 4 6 0.4 1450 10 5 5 0.5 1451 10 5 5 0.5 1452 10 4 6 0.4 1453 10 8 2 0.8 1454 10 6 4 0.6 1455 10 4 6 0.4 1456 10 5 5 0.5 1457 10 2 8 0.2 1458 10 3 7 0.3 1459 10 5 5 0.5 1460 10 3 7 0.3 1461 10 8 2 0.8 1462 10 6 4 0.6 1463 10 3 7 0.3 1464 10 6 4 0.6 1465 10 4 6 0.4 1466 10 6 4 0.6 1467 10 7 3 0.7 1468 10 7 3 0.7 1469 10 4 6 0.4 1470 10 4 6 0.4 1471 10 7 3 0.7 1472 10 7 3 0.7 1473 10 4 6 0.4 1474 10 1 9 0.1 1475 10 7 3 0.7 1476 10 8 2 0.8 1477 10 4 6 0.4 1478 10 4 6 0.4 1479 10 6 4 0.6 1480 10 5 5 0.5 1481 10 2 8 0.2 1482 10 5 5 0.5 1483 10 1 9 0.1 1484 10 4 6 0.4 1485 10 8 2 0.8 1486 10 5 5 0.5 1487 10 5 5 0.5 1488 10 5 5 0.5 1489 10 5 5 0.5 1490 10 5 5 0.5 1491 10 6 4 0.6 1492 10 4 6 0.4 1493 10 4 6 0.4 1494 10 2 8 0.2 1495 10 3 7 0.3 1496 10 6 4 0.6 1497 10 4 6 0.4 1498 10 7 3 0.7 1499 10 6 4 0.6 1500 10 1 9 0.1 1501 10 4 6 0.4 1502 10 6 4 0.6 1503 10 3 7 0.3 1504 10 5 5 0.5 1505 10 5 5 0.5 1506 10 4 6 0.4 1507 10 4 6 0.4 1508 10 5 5 0.5 1509 10 4 6 0.4 1510 10 5 5 0.5 1511 10 2 8 0.2 1512 10 3 7 0.3 1513 10 3 7 0.3 1514 10 4 6 0.4 1515 10 6 4 0.6 1516 10 5 5 0.5 1517 10 4 6 0.4 1518 10 4 6 0.4 1519 10 4 6 0.4 1520 10 4 6 0.4 1521 10 3 7 0.3 1522 10 5 5 0.5 1523 10 6 4 0.6 1524 10 3 7 0.3 1525 10 5 5 0.5 1526 10 5 5 0.5 1527 10 6 4 0.6 1528 10 7 3 0.7 1529 10 7 3 0.7 1530 10 6 4 0.6 1531 10 6 4 0.6 1532 10 3 7 0.3 1533 10 6 4 0.6 1534 10 6 4 0.6 1535 10 6 4 0.6 1536 10 6 4 0.6 1537 10 6 4 0.6 1538 10 4 6 0.4 1539 10 5 5 0.5 1540 10 5 5 0.5 1541 10 6 4 0.6 1542 10 5 5 0.5 1543 10 7 3 0.7 1544 10 5 5 0.5 1545 10 3 7 0.3 1546 10 5 5 0.5 1547 10 7 3 0.7 1548 10 5 5 0.5 1549 10 5 5 0.5 1550 10 3 7 0.3 1551 10 6 4 0.6 1552 10 5 5 0.5 1553 10 5 5 0.5 1554 10 4 6 0.4 1555 10 5 5 0.5 1556 10 5 5 0.5 1557 10 4 6 0.4 1558 10 3 7 0.3 1559 10 3 7 0.3 1560 10 5 5 0.5 1561 10 7 3 0.7 1562 10 4 6 0.4 1563 10 2 8 0.2 1564 10 5 5 0.5 1565 10 5 5 0.5 1566 10 6 4 0.6 1567 10 5 5 0.5 1568 10 6 4 0.6 1569 10 2 8 0.2 1570 10 3 7 0.3 1571 10 7 3 0.7 1572 10 5 5 0.5 1573 10 7 3 0.7 1574 10 1 9 0.1 1575 10 6 4 0.6 1576 10 7 3 0.7 1577 10 4 6 0.4 1578 10 4 6 0.4 1579 10 6 4 0.6 1580 10 7 3 0.7 1581 10 4 6 0.4 1582 10 7 3 0.7 1583 10 5 5 0.5 1584 10 7 3 0.7 1585 10 6 4 0.6 1586 10 7 3 0.7 1587 10 6 4 0.6 1588 10 5 5 0.5 1589 10 3 7 0.3 1590 10 4 6 0.4 1591 10 2 8 0.2 1592 10 4 6 0.4 1593 10 4 6 0.4 1594 10 3 7 0.3 1595 10 5 5 0.5 1596 10 5 5 0.5 1597 10 7 3 0.7 1598 10 6 4 0.6 1599 10 5 5 0.5 1600 10 6 4 0.6 1601 10 6 4 0.6 1602 10 5 5 0.5 1603 10 5 5 0.5 1604 10 5 5 0.5 1605 10 4 6 0.4 1606 10 3 7 0.3 1607 10 3 7 0.3 1608 10 5 5 0.5 1609 10 6 4 0.6 1610 10 6 4 0.6 1611 10 4 6 0.4 1612 10 5 5 0.5 1613 10 5 5 0.5 1614 10 4 6 0.4 1615 10 4 6 0.4 1616 10 6 4 0.6 1617 10 6 4 0.6 1618 10 5 5 0.5 1619 10 4 6 0.4 1620 10 5 5 0.5 1621 10 5 5 0.5 1622 10 7 3 0.7 1623 10 7 3 0.7 1624 10 5 5 0.5 1625 10 4 6 0.4 1626 10 2 8 0.2 1627 10 7 3 0.7 1628 10 6 4 0.6 1629 10 3 7 0.3 1630 10 7 3 0.7 1631 10 5 5 0.5 1632 10 4 6 0.4 1633 10 7 3 0.7 1634 10 6 4 0.6 1635 10 6 4 0.6 1636 10 3 7 0.3 1637 10 5 5 0.5 1638 10 6 4 0.6 1639 10 4 6 0.4 1640 10 4 6 0.4 1641 10 5 5 0.5 1642 10 5 5 0.5 1643 10 3 7 0.3 1644 10 7 3 0.7 1645 10 6 4 0.6 1646 10 5 5 0.5 1647 10 6 4 0.6 1648 10 6 4 0.6 1649 10 7 3 0.7 1650 10 6 4 0.6 1651 10 5 5 0.5 1652 10 7 3 0.7 1653 10 7 3 0.7 1654 10 8 2 0.8 1655 10 5 5 0.5 1656 10 5 5 0.5 1657 10 5 5 0.5 1658 10 6 4 0.6 1659 10 4 6 0.4 1660 10 5 5 0.5 1661 10 6 4 0.6 1662 10 5 5 0.5 1663 10 4 6 0.4 1664 10 5 5 0.5 1665 10 9 1 0.9 1666 10 7 3 0.7 1667 10 1 9 0.1 1668 10 6 4 0.6 1669 10 3 7 0.3 1670 10 7 3 0.7 1671 10 6 4 0.6 1672 10 4 6 0.4 1673 10 7 3 0.7 1674 10 5 5 0.5 1675 10 7 3 0.7 1676 10 7 3 0.7 1677 10 4 6 0.4 1678 10 4 6 0.4 1679 10 7 3 0.7 1680 10 4 6 0.4 1681 10 6 4 0.6 1682 10 6 4 0.6 1683 10 8 2 0.8 1684 10 4 6 0.4 1685 10 4 6 0.4 1686 10 4 6 0.4 1687 10 7 3 0.7 1688 10 5 5 0.5 1689 10 5 5 0.5 1690 10 3 7 0.3 1691 10 5 5 0.5 1692 10 5 5 0.5 1693 10 7 3 0.7 1694 10 4 6 0.4 1695 10 3 7 0.3 1696 10 6 4 0.6 1697 10 4 6 0.4 1698 10 3 7 0.3 1699 10 6 4 0.6 1700 10 6 4 0.6 1701 10 4 6 0.4 1702 10 6 4 0.6 1703 10 5 5 0.5 1704 10 2 8 0.2 1705 10 5 5 0.5 1706 10 5 5 0.5 1707 10 6 4 0.6 1708 10 8 2 0.8 1709 10 5 5 0.5 1710 10 5 5 0.5 1711 10 4 6 0.4 1712 10 4 6 0.4 1713 10 6 4 0.6 1714 10 7 3 0.7 1715 10 6 4 0.6 1716 10 2 8 0.2 1717 10 3 7 0.3 1718 10 3 7 0.3 1719 10 5 5 0.5 1720 10 7 3 0.7 1721 10 4 6 0.4 1722 10 5 5 0.5 1723 10 6 4 0.6 1724 10 3 7 0.3 1725 10 5 5 0.5 1726 10 3 7 0.3 1727 10 6 4 0.6 1728 10 3 7 0.3 1729 10 6 4 0.6 1730 10 7 3 0.7 1731 10 6 4 0.6 1732 10 5 5 0.5 1733 10 5 5 0.5 1734 10 3 7 0.3 1735 10 5 5 0.5 1736 10 3 7 0.3 1737 10 6 4 0.6 1738 10 4 6 0.4 1739 10 5 5 0.5 1740 10 5 5 0.5 1741 10 7 3 0.7 1742 10 4 6 0.4 1743 10 6 4 0.6 1744 10 5 5 0.5 1745 10 5 5 0.5 1746 10 7 3 0.7 1747 10 3 7 0.3 1748 10 6 4 0.6 1749 10 3 7 0.3 1750 10 4 6 0.4 1751 10 2 8 0.2 1752 10 4 6 0.4 1753 10 3 7 0.3 1754 10 4 6 0.4 1755 10 6 4 0.6 1756 10 5 5 0.5 1757 10 9 1 0.9 1758 10 3 7 0.3 1759 10 7 3 0.7 1760 10 4 6 0.4 1761 10 5 5 0.5 1762 10 4 6 0.4 1763 10 9 1 0.9 1764 10 3 7 0.3 1765 10 4 6 0.4 1766 10 7 3 0.7 1767 10 3 7 0.3 1768 10 3 7 0.3 1769 10 5 5 0.5 1770 10 5 5 0.5 1771 10 5 5 0.5 1772 10 3 7 0.3 1773 10 3 7 0.3 1774 10 4 6 0.4 1775 10 5 5 0.5 1776 10 5 5 0.5 1777 10 6 4 0.6 1778 10 4 6 0.4 1779 10 5 5 0.5 1780 10 7 3 0.7 1781 10 4 6 0.4 1782 10 5 5 0.5 1783 10 3 7 0.3 1784 10 5 5 0.5 1785 10 5 5 0.5 1786 10 4 6 0.4 1787 10 5 5 0.5 1788 10 5 5 0.5 1789 10 8 2 0.8 1790 10 3 7 0.3 1791 10 4 6 0.4 1792 10 4 6 0.4 1793 10 5 5 0.5 1794 10 4 6 0.4 1795 10 8 2 0.8 1796 10 4 6 0.4 1797 10 7 3 0.7 1798 10 5 5 0.5 1799 10 6 4 0.6 1800 10 4 6 0.4 1801 10 5 5 0.5 1802 10 3 7 0.3 1803 10 5 5 0.5 1804 10 4 6 0.4 1805 10 6 4 0.6 1806 10 3 7 0.3 1807 10 2 8 0.2 1808 10 7 3 0.7 1809 10 7 3 0.7 1810 10 4 6 0.4 1811 10 7 3 0.7 1812 10 5 5 0.5 1813 10 5 5 0.5 1814 10 6 4 0.6 1815 10 8 2 0.8 1816 10 7 3 0.7 1817 10 6 4 0.6 1818 10 4 6 0.4 1819 10 5 5 0.5 1820 10 6 4 0.6 1821 10 8 2 0.8 1822 10 6 4 0.6 1823 10 5 5 0.5 1824 10 4 6 0.4 1825 10 5 5 0.5 1826 10 3 7 0.3 1827 10 7 3 0.7 1828 10 5 5 0.5 1829 10 5 5 0.5 1830 10 3 7 0.3 1831 10 6 4 0.6 1832 10 5 5 0.5 1833 10 6 4 0.6 1834 10 4 6 0.4 1835 10 5 5 0.5 1836 10 7 3 0.7 1837 10 3 7 0.3 1838 10 4 6 0.4 1839 10 5 5 0.5 1840 10 7 3 0.7 1841 10 4 6 0.4 1842 10 5 5 0.5 1843 10 4 6 0.4 1844 10 6 4 0.6 1845 10 3 7 0.3 1846 10 3 7 0.3 1847 10 5 5 0.5 1848 10 6 4 0.6 1849 10 5 5 0.5 1850 10 6 4 0.6 1851 10 6 4 0.6 1852 10 4 6 0.4 1853 10 2 8 0.2 1854 10 4 6 0.4 1855 10 4 6 0.4 1856 10 3 7 0.3 1857 10 4 6 0.4 1858 10 3 7 0.3 1859 10 7 3 0.7 1860 10 4 6 0.4 1861 10 6 4 0.6 1862 10 4 6 0.4 1863 10 5 5 0.5 1864 10 3 7 0.3 1865 10 5 5 0.5 1866 10 6 4 0.6 1867 10 4 6 0.4 1868 10 3 7 0.3 1869 10 4 6 0.4 1870 10 5 5 0.5 1871 10 5 5 0.5 1872 10 5 5 0.5 1873 10 9 1 0.9 1874 10 4 6 0.4 1875 10 5 5 0.5 1876 10 5 5 0.5 1877 10 4 6 0.4 1878 10 7 3 0.7 1879 10 7 3 0.7 1880 10 6 4 0.6 1881 10 4 6 0.4 1882 10 7 3 0.7 1883 10 4 6 0.4 1884 10 8 2 0.8 1885 10 4 6 0.4 1886 10 3 7 0.3 1887 10 4 6 0.4 1888 10 3 7 0.3 1889 10 6 4 0.6 1890 10 5 5 0.5 1891 10 6 4 0.6 1892 10 3 7 0.3 1893 10 4 6 0.4 1894 10 8 2 0.8 1895 10 5 5 0.5 1896 10 3 7 0.3 1897 10 6 4 0.6 1898 10 5 5 0.5 1899 10 4 6 0.4 1900 10 4 6 0.4 1901 10 5 5 0.5 1902 10 2 8 0.2 1903 10 4 6 0.4 1904 10 5 5 0.5 1905 10 2 8 0.2 1906 10 5 5 0.5 1907 10 5 5 0.5 1908 10 5 5 0.5 1909 10 5 5 0.5 1910 10 4 6 0.4 1911 10 8 2 0.8 1912 10 5 5 0.5 1913 10 4 6 0.4 1914 10 5 5 0.5 1915 10 5 5 0.5 1916 10 6 4 0.6 1917 10 2 8 0.2 1918 10 5 5 0.5 1919 10 4 6 0.4 1920 10 5 5 0.5 1921 10 3 7 0.3 1922 10 4 6 0.4 1923 10 5 5 0.5 1924 10 5 5 0.5 1925 10 5 5 0.5 1926 10 8 2 0.8 1927 10 4 6 0.4 1928 10 9 1 0.9 1929 10 6 4 0.6 1930 10 6 4 0.6 1931 10 6 4 0.6 1932 10 4 6 0.4 1933 10 6 4 0.6 1934 10 9 1 0.9 1935 10 6 4 0.6 1936 10 6 4 0.6 1937 10 5 5 0.5 1938 10 5 5 0.5 1939 10 4 6 0.4 1940 10 4 6 0.4 1941 10 3 7 0.3 1942 10 4 6 0.4 1943 10 3 7 0.3 1944 10 2 8 0.2 1945 10 5 5 0.5 1946 10 3 7 0.3 1947 10 4 6 0.4 1948 10 4 6 0.4 1949 10 3 7 0.3 1950 10 7 3 0.7 1951 10 4 6 0.4 1952 10 6 4 0.6 1953 10 5 5 0.5 1954 10 6 4 0.6 1955 10 4 6 0.4 1956 10 7 3 0.7 1957 10 7 3 0.7 1958 10 5 5 0.5 1959 10 5 5 0.5 1960 10 3 7 0.3 1961 10 6 4 0.6 1962 10 7 3 0.7 1963 10 6 4 0.6 1964 10 5 5 0.5 1965 10 5 5 0.5 1966 10 4 6 0.4 1967 10 6 4 0.6 1968 10 5 5 0.5 1969 10 8 2 0.8 1970 10 4 6 0.4 1971 10 2 8 0.2 1972 10 4 6 0.4 1973 10 4 6 0.4 1974 10 7 3 0.7 1975 10 7 3 0.7 1976 10 6 4 0.6 1977 10 4 6 0.4 1978 10 7 3 0.7 1979 10 6 4 0.6 1980 10 4 6 0.4 1981 10 3 7 0.3 1982 10 4 6 0.4 1983 10 4 6 0.4 1984 10 6 4 0.6 1985 10 5 5 0.5 1986 10 7 3 0.7 1987 10 5 5 0.5 1988 10 4 6 0.4 1989 10 4 6 0.4 1990 10 6 4 0.6 1991 10 5 5 0.5 1992 10 7 3 0.7 1993 10 5 5 0.5 1994 10 3 7 0.3 1995 10 5 5 0.5 1996 10 2 8 0.2 1997 10 7 3 0.7 1998 10 6 4 0.6 1999 10 6 4 0.6 2000 10 3 7 0.3 2001 10 4 6 0.4 2002 10 5 5 0.5 2003 10 6 4 0.6 2004 10 2 8 0.2 2005 10 5 5 0.5 2006 10 6 4 0.6 2007 10 4 6 0.4 2008 10 4 6 0.4 2009 10 8 2 0.8 2010 10 8 2 0.8 2011 10 6 4 0.6 2012 10 5 5 0.5 2013 10 5 5 0.5 2014 10 6 4 0.6 2015 10 4 6 0.4 2016 10 5 5 0.5 2017 10 5 5 0.5 2018 10 6 4 0.6 2019 10 5 5 0.5 2020 10 4 6 0.4 2021 10 5 5 0.5 2022 10 5 5 0.5 2023 10 8 2 0.8 2024 10 6 4 0.6 2025 10 3 7 0.3 2026 10 4 6 0.4 2027 10 4 6 0.4 2028 10 4 6 0.4 2029 10 7 3 0.7 2030 10 5 5 0.5 2031 10 4 6 0.4 2032 10 4 6 0.4 2033 10 6 4 0.6 2034 10 5 5 0.5 2035 10 6 4 0.6 2036 10 5 5 0.5 2037 10 3 7 0.3 2038 10 5 5 0.5 2039 10 6 4 0.6 2040 10 5 5 0.5 2041 10 4 6 0.4 2042 10 5 5 0.5 2043 10 5 5 0.5 2044 10 5 5 0.5 2045 10 3 7 0.3 2046 10 6 4 0.6 2047 10 4 6 0.4 2048 10 4 6 0.4 2049 10 5 5 0.5 2050 10 5 5 0.5 2051 10 5 5 0.5 2052 10 10 0 1.0 2053 10 6 4 0.6 2054 10 5 5 0.5 2055 10 4 6 0.4 2056 10 3 7 0.3 2057 10 6 4 0.6 2058 10 6 4 0.6 2059 10 4 6 0.4 2060 10 5 5 0.5 2061 10 6 4 0.6 2062 10 5 5 0.5 2063 10 5 5 0.5 2064 10 4 6 0.4 2065 10 6 4 0.6 2066 10 4 6 0.4 2067 10 6 4 0.6 2068 10 6 4 0.6 2069 10 6 4 0.6 2070 10 4 6 0.4 2071 10 5 5 0.5 2072 10 4 6 0.4 2073 10 5 5 0.5 2074 10 5 5 0.5 2075 10 4 6 0.4 2076 10 5 5 0.5 2077 10 6 4 0.6 2078 10 7 3 0.7 2079 10 5 5 0.5 2080 10 6 4 0.6 2081 10 7 3 0.7 2082 10 5 5 0.5 2083 10 4 6 0.4 2084 10 6 4 0.6 2085 10 8 2 0.8 2086 10 5 5 0.5 2087 10 4 6 0.4 2088 10 4 6 0.4 2089 10 7 3 0.7 2090 10 7 3 0.7 2091 10 5 5 0.5 2092 10 5 5 0.5 2093 10 5 5 0.5 2094 10 8 2 0.8 2095 10 7 3 0.7 2096 10 6 4 0.6 2097 10 9 1 0.9 2098 10 6 4 0.6 2099 10 5 5 0.5 2100 10 3 7 0.3 2101 10 5 5 0.5 2102 10 4 6 0.4 2103 10 6 4 0.6 2104 10 2 8 0.2 2105 10 6 4 0.6 2106 10 6 4 0.6 2107 10 5 5 0.5 2108 10 6 4 0.6 2109 10 7 3 0.7 2110 10 6 4 0.6 2111 10 7 3 0.7 2112 10 6 4 0.6 2113 10 8 2 0.8 2114 10 4 6 0.4 2115 10 7 3 0.7 2116 10 5 5 0.5 2117 10 6 4 0.6 2118 10 6 4 0.6 2119 10 2 8 0.2 2120 10 6 4 0.6 2121 10 4 6 0.4 2122 10 4 6 0.4 2123 10 7 3 0.7 2124 10 5 5 0.5 2125 10 6 4 0.6 2126 10 2 8 0.2 2127 10 4 6 0.4 2128 10 3 7 0.3 2129 10 2 8 0.2 2130 10 1 9 0.1 2131 10 5 5 0.5 2132 10 4 6 0.4 2133 10 6 4 0.6 2134 10 8 2 0.8 2135 10 4 6 0.4 2136 10 5 5 0.5 2137 10 3 7 0.3 2138 10 6 4 0.6 2139 10 4 6 0.4 2140 10 3 7 0.3 2141 10 7 3 0.7 2142 10 5 5 0.5 2143 10 3 7 0.3 2144 10 6 4 0.6 2145 10 5 5 0.5 2146 10 6 4 0.6 2147 10 7 3 0.7 2148 10 6 4 0.6 2149 10 6 4 0.6 2150 10 6 4 0.6 2151 10 4 6 0.4 2152 10 4 6 0.4 2153 10 6 4 0.6 2154 10 4 6 0.4 2155 10 5 5 0.5 2156 10 6 4 0.6 2157 10 4 6 0.4 2158 10 7 3 0.7 2159 10 7 3 0.7 2160 10 5 5 0.5 2161 10 5 5 0.5 2162 10 6 4 0.6 2163 10 7 3 0.7 2164 10 6 4 0.6 2165 10 8 2 0.8 2166 10 4 6 0.4 2167 10 6 4 0.6 2168 10 4 6 0.4 2169 10 8 2 0.8 2170 10 8 2 0.8 2171 10 5 5 0.5 2172 10 6 4 0.6 2173 10 4 6 0.4 2174 10 7 3 0.7 2175 10 3 7 0.3 2176 10 5 5 0.5 2177 10 6 4 0.6 2178 10 6 4 0.6 2179 10 6 4 0.6 2180 10 5 5 0.5 2181 10 5 5 0.5 2182 10 4 6 0.4 2183 10 7 3 0.7 2184 10 7 3 0.7 2185 10 5 5 0.5 2186 10 5 5 0.5 2187 10 3 7 0.3 2188 10 5 5 0.5 2189 10 5 5 0.5 2190 10 7 3 0.7 2191 10 1 9 0.1 2192 10 5 5 0.5 2193 10 4 6 0.4 2194 10 4 6 0.4 2195 10 5 5 0.5 2196 10 3 7 0.3 2197 10 2 8 0.2 2198 10 5 5 0.5 2199 10 5 5 0.5 2200 10 7 3 0.7 2201 10 7 3 0.7 2202 10 9 1 0.9 2203 10 4 6 0.4 2204 10 4 6 0.4 2205 10 6 4 0.6 2206 10 5 5 0.5 2207 10 6 4 0.6 2208 10 7 3 0.7 2209 10 4 6 0.4 2210 10 3 7 0.3 2211 10 8 2 0.8 2212 10 4 6 0.4 2213 10 8 2 0.8 2214 10 3 7 0.3 2215 10 3 7 0.3 2216 10 6 4 0.6 2217 10 6 4 0.6 2218 10 7 3 0.7 2219 10 3 7 0.3 2220 10 8 2 0.8 2221 10 6 4 0.6 2222 10 3 7 0.3 2223 10 6 4 0.6 2224 10 4 6 0.4 2225 10 3 7 0.3 2226 10 7 3 0.7 2227 10 3 7 0.3 2228 10 8 2 0.8 2229 10 3 7 0.3 2230 10 4 6 0.4 2231 10 2 8 0.2 2232 10 4 6 0.4 2233 10 8 2 0.8 2234 10 5 5 0.5 2235 10 6 4 0.6 2236 10 3 7 0.3 2237 10 4 6 0.4 2238 10 6 4 0.6 2239 10 5 5 0.5 2240 10 7 3 0.7 2241 10 4 6 0.4 2242 10 3 7 0.3 2243 10 7 3 0.7 2244 10 7 3 0.7 2245 10 5 5 0.5 2246 10 4 6 0.4 2247 10 6 4 0.6 2248 10 6 4 0.6 2249 10 6 4 0.6 2250 10 7 3 0.7 2251 10 6 4 0.6 2252 10 7 3 0.7 2253 10 3 7 0.3 2254 10 7 3 0.7 2255 10 3 7 0.3 2256 10 4 6 0.4 2257 10 5 5 0.5 2258 10 6 4 0.6 2259 10 4 6 0.4 2260 10 2 8 0.2 2261 10 2 8 0.2 2262 10 2 8 0.2 2263 10 6 4 0.6 2264 10 7 3 0.7 2265 10 6 4 0.6 2266 10 5 5 0.5 2267 10 4 6 0.4 2268 10 7 3 0.7 2269 10 5 5 0.5 2270 10 4 6 0.4 2271 10 7 3 0.7 2272 10 5 5 0.5 2273 10 8 2 0.8 2274 10 3 7 0.3 2275 10 5 5 0.5 2276 10 4 6 0.4 2277 10 4 6 0.4 2278 10 7 3 0.7 2279 10 5 5 0.5 2280 10 4 6 0.4 2281 10 6 4 0.6 2282 10 4 6 0.4 2283 10 6 4 0.6 2284 10 6 4 0.6 2285 10 4 6 0.4 2286 10 4 6 0.4 2287 10 3 7 0.3 2288 10 4 6 0.4 2289 10 5 5 0.5 2290 10 4 6 0.4 2291 10 6 4 0.6 2292 10 8 2 0.8 2293 10 3 7 0.3 2294 10 4 6 0.4 2295 10 8 2 0.8 2296 10 7 3 0.7 2297 10 6 4 0.6 2298 10 6 4 0.6 2299 10 4 6 0.4 2300 10 8 2 0.8 2301 10 6 4 0.6 2302 10 3 7 0.3 2303 10 5 5 0.5 2304 10 5 5 0.5 2305 10 2 8 0.2 2306 10 4 6 0.4 2307 10 5 5 0.5 2308 10 4 6 0.4 2309 10 6 4 0.6 2310 10 7 3 0.7 2311 10 6 4 0.6 2312 10 7 3 0.7 2313 10 6 4 0.6 2314 10 7 3 0.7 2315 10 3 7 0.3 2316 10 7 3 0.7 2317 10 5 5 0.5 2318 10 5 5 0.5 2319 10 5 5 0.5 2320 10 5 5 0.5 2321 10 5 5 0.5 2322 10 6 4 0.6 2323 10 8 2 0.8 2324 10 7 3 0.7 2325 10 3 7 0.3 2326 10 6 4 0.6 2327 10 4 6 0.4 2328 10 8 2 0.8 2329 10 8 2 0.8 2330 10 7 3 0.7 2331 10 6 4 0.6 2332 10 3 7 0.3 2333 10 5 5 0.5 2334 10 3 7 0.3 2335 10 4 6 0.4 2336 10 5 5 0.5 2337 10 3 7 0.3 2338 10 3 7 0.3 2339 10 5 5 0.5 2340 10 6 4 0.6 2341 10 4 6 0.4 2342 10 7 3 0.7 2343 10 4 6 0.4 2344 10 3 7 0.3 2345 10 4 6 0.4 2346 10 2 8 0.2 2347 10 5 5 0.5 2348 10 7 3 0.7 2349 10 6 4 0.6 2350 10 7 3 0.7 2351 10 6 4 0.6 2352 10 6 4 0.6 2353 10 6 4 0.6 2354 10 9 1 0.9 2355 10 5 5 0.5 2356 10 4 6 0.4 2357 10 3 7 0.3 2358 10 6 4 0.6 2359 10 5 5 0.5 2360 10 4 6 0.4 2361 10 4 6 0.4 2362 10 5 5 0.5 2363 10 4 6 0.4 2364 10 5 5 0.5 2365 10 8 2 0.8 2366 10 6 4 0.6 2367 10 5 5 0.5 2368 10 6 4 0.6 2369 10 3 7 0.3 2370 10 5 5 0.5 2371 10 8 2 0.8 2372 10 6 4 0.6 2373 10 3 7 0.3 2374 10 6 4 0.6 2375 10 3 7 0.3 2376 10 3 7 0.3 2377 10 4 6 0.4 2378 10 3 7 0.3 2379 10 6 4 0.6 2380 10 5 5 0.5 2381 10 7 3 0.7 2382 10 6 4 0.6 2383 10 4 6 0.4 2384 10 6 4 0.6 2385 10 6 4 0.6 2386 10 5 5 0.5 2387 10 5 5 0.5 2388 10 7 3 0.7 2389 10 5 5 0.5 2390 10 4 6 0.4 2391 10 4 6 0.4 2392 10 5 5 0.5 2393 10 6 4 0.6 2394 10 3 7 0.3 2395 10 7 3 0.7 2396 10 5 5 0.5 2397 10 6 4 0.6 2398 10 4 6 0.4 2399 10 5 5 0.5 2400 10 3 7 0.3 2401 10 5 5 0.5 2402 10 6 4 0.6 2403 10 3 7 0.3 2404 10 4 6 0.4 2405 10 1 9 0.1 2406 10 5 5 0.5 2407 10 6 4 0.6 2408 10 7 3 0.7 2409 10 3 7 0.3 2410 10 8 2 0.8 2411 10 2 8 0.2 2412 10 5 5 0.5 2413 10 6 4 0.6 2414 10 8 2 0.8 2415 10 4 6 0.4 2416 10 1 9 0.1 2417 10 4 6 0.4 2418 10 8 2 0.8 2419 10 4 6 0.4 2420 10 5 5 0.5 2421 10 5 5 0.5 2422 10 3 7 0.3 2423 10 6 4 0.6 2424 10 2 8 0.2 2425 10 6 4 0.6 2426 10 4 6 0.4 2427 10 7 3 0.7 2428 10 4 6 0.4 2429 10 8 2 0.8 2430 10 6 4 0.6 2431 10 5 5 0.5 2432 10 4 6 0.4 2433 10 6 4 0.6 2434 10 5 5 0.5 2435 10 2 8 0.2 2436 10 6 4 0.6 2437 10 5 5 0.5 2438 10 8 2 0.8 2439 10 3 7 0.3 2440 10 4 6 0.4 2441 10 7 3 0.7 2442 10 7 3 0.7 2443 10 4 6 0.4 2444 10 5 5 0.5 2445 10 4 6 0.4 2446 10 2 8 0.2 2447 10 4 6 0.4 2448 10 5 5 0.5 2449 10 4 6 0.4 2450 10 2 8 0.2 2451 10 7 3 0.7 2452 10 6 4 0.6 2453 10 6 4 0.6 2454 10 7 3 0.7 2455 10 5 5 0.5 2456 10 5 5 0.5 2457 10 6 4 0.6 2458 10 5 5 0.5 2459 10 4 6 0.4 2460 10 4 6 0.4 2461 10 4 6 0.4 2462 10 6 4 0.6 2463 10 6 4 0.6 2464 10 4 6 0.4 2465 10 6 4 0.6 2466 10 4 6 0.4 2467 10 2 8 0.2 2468 10 4 6 0.4 2469 10 7 3 0.7 2470 10 2 8 0.2 2471 10 3 7 0.3 2472 10 4 6 0.4 2473 10 8 2 0.8 2474 10 5 5 0.5 2475 10 1 9 0.1 2476 10 7 3 0.7 2477 10 4 6 0.4 2478 10 5 5 0.5 2479 10 7 3 0.7 2480 10 6 4 0.6 2481 10 7 3 0.7 2482 10 5 5 0.5 2483 10 4 6 0.4 2484 10 4 6 0.4 2485 10 4 6 0.4 2486 10 4 6 0.4 2487 10 5 5 0.5 2488 10 2 8 0.2 2489 10 5 5 0.5 2490 10 6 4 0.6 2491 10 7 3 0.7 2492 10 4 6 0.4 2493 10 5 5 0.5 2494 10 6 4 0.6 2495 10 3 7 0.3 2496 10 6 4 0.6 2497 10 6 4 0.6 2498 10 4 6 0.4 2499 10 8 2 0.8 2500 10 4 6 0.4 2501 10 2 8 0.2 2502 10 5 5 0.5 2503 10 6 4 0.6 2504 10 4 6 0.4 2505 10 4 6 0.4 2506 10 6 4 0.6 2507 10 4 6 0.4 2508 10 6 4 0.6 2509 10 6 4 0.6 2510 10 3 7 0.3 2511 10 4 6 0.4 2512 10 6 4 0.6 2513 10 6 4 0.6 2514 10 4 6 0.4 2515 10 5 5 0.5 2516 10 7 3 0.7 2517 10 2 8 0.2 2518 10 6 4 0.6 2519 10 4 6 0.4 2520 10 6 4 0.6 2521 10 6 4 0.6 2522 10 4 6 0.4 2523 10 6 4 0.6 2524 10 4 6 0.4 2525 10 6 4 0.6 2526 10 6 4 0.6 2527 10 7 3 0.7 2528 10 6 4 0.6 2529 10 4 6 0.4 2530 10 7 3 0.7 2531 10 7 3 0.7 2532 10 7 3 0.7 2533 10 5 5 0.5 2534 10 5 5 0.5 2535 10 3 7 0.3 2536 10 5 5 0.5 2537 10 3 7 0.3 2538 10 6 4 0.6 2539 10 3 7 0.3 2540 10 9 1 0.9 2541 10 3 7 0.3 2542 10 5 5 0.5 2543 10 4 6 0.4 2544 10 5 5 0.5 2545 10 5 5 0.5 2546 10 5 5 0.5 2547 10 6 4 0.6 2548 10 2 8 0.2 2549 10 5 5 0.5 2550 10 6 4 0.6 2551 10 2 8 0.2 2552 10 6 4 0.6 2553 10 7 3 0.7 2554 10 5 5 0.5 2555 10 7 3 0.7 2556 10 7 3 0.7 2557 10 6 4 0.6 2558 10 3 7 0.3 2559 10 6 4 0.6 2560 10 8 2 0.8 2561 10 2 8 0.2 2562 10 4 6 0.4 2563 10 5 5 0.5 2564 10 7 3 0.7 2565 10 2 8 0.2 2566 10 7 3 0.7 2567 10 6 4 0.6 2568 10 9 1 0.9 2569 10 6 4 0.6 2570 10 4 6 0.4 2571 10 7 3 0.7 2572 10 6 4 0.6 2573 10 4 6 0.4 2574 10 8 2 0.8 2575 10 6 4 0.6 2576 10 5 5 0.5 2577 10 5 5 0.5 2578 10 2 8 0.2 2579 10 5 5 0.5 2580 10 5 5 0.5 2581 10 3 7 0.3 2582 10 4 6 0.4 2583 10 5 5 0.5 2584 10 6 4 0.6 2585 10 6 4 0.6 2586 10 6 4 0.6 2587 10 4 6 0.4 2588 10 4 6 0.4 2589 10 7 3 0.7 2590 10 4 6 0.4 2591 10 5 5 0.5 2592 10 6 4 0.6 2593 10 5 5 0.5 2594 10 5 5 0.5 2595 10 5 5 0.5 2596 10 7 3 0.7 2597 10 5 5 0.5 2598 10 6 4 0.6 2599 10 5 5 0.5 2600 10 6 4 0.6 2601 10 5 5 0.5 2602 10 7 3 0.7 2603 10 8 2 0.8 2604 10 8 2 0.8 2605 10 6 4 0.6 2606 10 6 4 0.6 2607 10 1 9 0.1 2608 10 6 4 0.6 2609 10 5 5 0.5 2610 10 1 9 0.1 2611 10 7 3 0.7 2612 10 2 8 0.2 2613 10 6 4 0.6 2614 10 6 4 0.6 2615 10 2 8 0.2 2616 10 6 4 0.6 2617 10 7 3 0.7 2618 10 5 5 0.5 2619 10 3 7 0.3 2620 10 2 8 0.2 2621 10 6 4 0.6 2622 10 3 7 0.3 2623 10 7 3 0.7 2624 10 6 4 0.6 2625 10 5 5 0.5 2626 10 2 8 0.2 2627 10 6 4 0.6 2628 10 3 7 0.3 2629 10 5 5 0.5 2630 10 5 5 0.5 2631 10 3 7 0.3 2632 10 7 3 0.7 2633 10 4 6 0.4 2634 10 6 4 0.6 2635 10 2 8 0.2 2636 10 4 6 0.4 2637 10 8 2 0.8 2638 10 6 4 0.6 2639 10 6 4 0.6 2640 10 4 6 0.4 2641 10 4 6 0.4 2642 10 3 7 0.3 2643 10 5 5 0.5 2644 10 3 7 0.3 2645 10 6 4 0.6 2646 10 5 5 0.5 2647 10 9 1 0.9 2648 10 8 2 0.8 2649 10 5 5 0.5 2650 10 7 3 0.7 2651 10 6 4 0.6 2652 10 5 5 0.5 2653 10 5 5 0.5 2654 10 5 5 0.5 2655 10 4 6 0.4 2656 10 5 5 0.5 2657 10 4 6 0.4 2658 10 5 5 0.5 2659 10 4 6 0.4 2660 10 5 5 0.5 2661 10 3 7 0.3 2662 10 4 6 0.4 2663 10 7 3 0.7 2664 10 4 6 0.4 2665 10 6 4 0.6 2666 10 5 5 0.5 2667 10 2 8 0.2 2668 10 2 8 0.2 2669 10 5 5 0.5 2670 10 5 5 0.5 2671 10 5 5 0.5 2672 10 8 2 0.8 2673 10 3 7 0.3 2674 10 2 8 0.2 2675 10 4 6 0.4 2676 10 7 3 0.7 2677 10 5 5 0.5 2678 10 6 4 0.6 2679 10 4 6 0.4 2680 10 5 5 0.5 2681 10 5 5 0.5 2682 10 2 8 0.2 2683 10 5 5 0.5 2684 10 6 4 0.6 2685 10 2 8 0.2 2686 10 7 3 0.7 2687 10 6 4 0.6 2688 10 4 6 0.4 2689 10 2 8 0.2 2690 10 9 1 0.9 2691 10 8 2 0.8 2692 10 6 4 0.6 2693 10 2 8 0.2 2694 10 5 5 0.5 2695 10 5 5 0.5 2696 10 6 4 0.6 2697 10 3 7 0.3 2698 10 5 5 0.5 2699 10 3 7 0.3 2700 10 6 4 0.6 2701 10 3 7 0.3 2702 10 2 8 0.2 2703 10 5 5 0.5 2704 10 6 4 0.6 2705 10 3 7 0.3 2706 10 5 5 0.5 2707 10 5 5 0.5 2708 10 5 5 0.5 2709 10 4 6 0.4 2710 10 3 7 0.3 2711 10 5 5 0.5 2712 10 4 6 0.4 2713 10 4 6 0.4 2714 10 6 4 0.6 2715 10 6 4 0.6 2716 10 3 7 0.3 2717 10 4 6 0.4 2718 10 4 6 0.4 2719 10 4 6 0.4 2720 10 6 4 0.6 2721 10 6 4 0.6 2722 10 4 6 0.4 2723 10 4 6 0.4 2724 10 3 7 0.3 2725 10 5 5 0.5 2726 10 5 5 0.5 2727 10 7 3 0.7 2728 10 5 5 0.5 2729 10 8 2 0.8 2730 10 6 4 0.6 2731 10 7 3 0.7 2732 10 3 7 0.3 2733 10 7 3 0.7 2734 10 3 7 0.3 2735 10 3 7 0.3 2736 10 5 5 0.5 2737 10 5 5 0.5 2738 10 3 7 0.3 2739 10 8 2 0.8 2740 10 4 6 0.4 2741 10 5 5 0.5 2742 10 8 2 0.8 2743 10 4 6 0.4 2744 10 6 4 0.6 2745 10 4 6 0.4 2746 10 8 2 0.8 2747 10 2 8 0.2 2748 10 8 2 0.8 2749 10 4 6 0.4 2750 10 3 7 0.3 2751 10 5 5 0.5 2752 10 7 3 0.7 2753 10 7 3 0.7 2754 10 2 8 0.2 2755 10 8 2 0.8 2756 10 3 7 0.3 2757 10 7 3 0.7 2758 10 7 3 0.7 2759 10 1 9 0.1 2760 10 6 4 0.6 2761 10 4 6 0.4 2762 10 3 7 0.3 2763 10 4 6 0.4 2764 10 6 4 0.6 2765 10 6 4 0.6 2766 10 5 5 0.5 2767 10 5 5 0.5 2768 10 6 4 0.6 2769 10 2 8 0.2 2770 10 5 5 0.5 2771 10 4 6 0.4 2772 10 5 5 0.5 2773 10 3 7 0.3 2774 10 3 7 0.3 2775 10 7 3 0.7 2776 10 4 6 0.4 2777 10 1 9 0.1 2778 10 7 3 0.7 2779 10 6 4 0.6 2780 10 4 6 0.4 2781 10 4 6 0.4 2782 10 6 4 0.6 2783 10 9 1 0.9 2784 10 3 7 0.3 2785 10 4 6 0.4 2786 10 6 4 0.6 2787 10 6 4 0.6 2788 10 5 5 0.5 2789 10 5 5 0.5 2790 10 5 5 0.5 2791 10 7 3 0.7 2792 10 6 4 0.6 2793 10 4 6 0.4 2794 10 5 5 0.5 2795 10 3 7 0.3 2796 10 7 3 0.7 2797 10 6 4 0.6 2798 10 4 6 0.4 2799 10 3 7 0.3 2800 10 7 3 0.7 2801 10 2 8 0.2 2802 10 5 5 0.5 2803 10 6 4 0.6 2804 10 4 6 0.4 2805 10 3 7 0.3 2806 10 6 4 0.6 2807 10 5 5 0.5 2808 10 3 7 0.3 2809 10 2 8 0.2 2810 10 7 3 0.7 2811 10 5 5 0.5 2812 10 5 5 0.5 2813 10 8 2 0.8 2814 10 6 4 0.6 2815 10 5 5 0.5 2816 10 7 3 0.7 2817 10 2 8 0.2 2818 10 5 5 0.5 2819 10 4 6 0.4 2820 10 5 5 0.5 2821 10 3 7 0.3 2822 10 7 3 0.7 2823 10 3 7 0.3 2824 10 7 3 0.7 2825 10 4 6 0.4 2826 10 8 2 0.8 2827 10 6 4 0.6 2828 10 8 2 0.8 2829 10 3 7 0.3 2830 10 7 3 0.7 2831 10 4 6 0.4 2832 10 5 5 0.5 2833 10 7 3 0.7 2834 10 4 6 0.4 2835 10 6 4 0.6 2836 10 3 7 0.3 2837 10 7 3 0.7 2838 10 5 5 0.5 2839 10 5 5 0.5 2840 10 7 3 0.7 2841 10 7 3 0.7 2842 10 6 4 0.6 2843 10 4 6 0.4 2844 10 5 5 0.5 2845 10 5 5 0.5 2846 10 4 6 0.4 2847 10 4 6 0.4 2848 10 4 6 0.4 2849 10 4 6 0.4 2850 10 3 7 0.3 2851 10 2 8 0.2 2852 10 2 8 0.2 2853 10 3 7 0.3 2854 10 6 4 0.6 2855 10 6 4 0.6 2856 10 7 3 0.7 2857 10 5 5 0.5 2858 10 4 6 0.4 2859 10 6 4 0.6 2860 10 5 5 0.5 2861 10 4 6 0.4 2862 10 4 6 0.4 2863 10 6 4 0.6 2864 10 5 5 0.5 2865 10 3 7 0.3 2866 10 4 6 0.4 2867 10 6 4 0.6 2868 10 4 6 0.4 2869 10 7 3 0.7 2870 10 3 7 0.3 2871 10 5 5 0.5 2872 10 8 2 0.8 2873 10 6 4 0.6 2874 10 4 6 0.4 2875 10 3 7 0.3 2876 10 6 4 0.6 2877 10 5 5 0.5 2878 10 5 5 0.5 2879 10 4 6 0.4 2880 10 8 2 0.8 2881 10 4 6 0.4 2882 10 5 5 0.5 2883 10 5 5 0.5 2884 10 6 4 0.6 2885 10 5 5 0.5 2886 10 4 6 0.4 2887 10 6 4 0.6 2888 10 5 5 0.5 2889 10 4 6 0.4 2890 10 5 5 0.5 2891 10 6 4 0.6 2892 10 3 7 0.3 2893 10 7 3 0.7 2894 10 5 5 0.5 2895 10 4 6 0.4 2896 10 3 7 0.3 2897 10 2 8 0.2 2898 10 2 8 0.2 2899 10 5 5 0.5 2900 10 5 5 0.5 2901 10 6 4 0.6 2902 10 7 3 0.7 2903 10 4 6 0.4 2904 10 7 3 0.7 2905 10 4 6 0.4 2906 10 5 5 0.5 2907 10 3 7 0.3 2908 10 0 10 0.0 2909 10 6 4 0.6 2910 10 7 3 0.7 2911 10 6 4 0.6 2912 10 3 7 0.3 2913 10 3 7 0.3 2914 10 5 5 0.5 2915 10 10 0 1.0 2916 10 5 5 0.5 2917 10 4 6 0.4 2918 10 2 8 0.2 2919 10 5 5 0.5 2920 10 4 6 0.4 2921 10 7 3 0.7 2922 10 9 1 0.9 2923 10 5 5 0.5 2924 10 5 5 0.5 2925 10 5 5 0.5 2926 10 3 7 0.3 2927 10 2 8 0.2 2928 10 4 6 0.4 2929 10 3 7 0.3 2930 10 4 6 0.4 2931 10 3 7 0.3 2932 10 4 6 0.4 2933 10 5 5 0.5 2934 10 4 6 0.4 2935 10 7 3 0.7 2936 10 6 4 0.6 2937 10 5 5 0.5 2938 10 3 7 0.3 2939 10 6 4 0.6 2940 10 3 7 0.3 2941 10 5 5 0.5 2942 10 8 2 0.8 2943 10 6 4 0.6 2944 10 2 8 0.2 2945 10 3 7 0.3 2946 10 7 3 0.7 2947 10 2 8 0.2 2948 10 5 5 0.5 2949 10 6 4 0.6 2950 10 6 4 0.6 2951 10 6 4 0.6 2952 10 3 7 0.3 2953 10 4 6 0.4 2954 10 6 4 0.6 2955 10 5 5 0.5 2956 10 3 7 0.3 2957 10 5 5 0.5 2958 10 2 8 0.2 2959 10 7 3 0.7 2960 10 6 4 0.6 2961 10 5 5 0.5 2962 10 5 5 0.5 2963 10 5 5 0.5 2964 10 2 8 0.2 2965 10 6 4 0.6 2966 10 7 3 0.7 2967 10 4 6 0.4 2968 10 4 6 0.4 2969 10 4 6 0.4 2970 10 4 6 0.4 2971 10 4 6 0.4 2972 10 3 7 0.3 2973 10 2 8 0.2 2974 10 4 6 0.4 2975 10 3 7 0.3 2976 10 4 6 0.4 2977 10 5 5 0.5 2978 10 3 7 0.3 2979 10 7 3 0.7 2980 10 4 6 0.4 2981 10 4 6 0.4 2982 10 7 3 0.7 2983 10 5 5 0.5 2984 10 7 3 0.7 2985 10 7 3 0.7 2986 10 2 8 0.2 2987 10 4 6 0.4 2988 10 5 5 0.5 2989 10 5 5 0.5 2990 10 6 4 0.6 2991 10 3 7 0.3 2992 10 4 6 0.4 2993 10 4 6 0.4 2994 10 7 3 0.7 2995 10 3 7 0.3 2996 10 4 6 0.4 2997 10 4 6 0.4 2998 10 8 2 0.8 2999 10 4 6 0.4 3000 10 4 6 0.4 3001 10 4 6 0.4 3002 10 8 2 0.8 3003 10 3 7 0.3 3004 10 6 4 0.6 3005 10 7 3 0.7 3006 10 3 7 0.3 3007 10 5 5 0.5 3008 10 3 7 0.3 3009 10 5 5 0.5 3010 10 5 5 0.5 3011 10 4 6 0.4 3012 10 8 2 0.8 3013 10 5 5 0.5 3014 10 5 5 0.5 3015 10 4 6 0.4 3016 10 4 6 0.4 3017 10 2 8 0.2 3018 10 4 6 0.4 3019 10 7 3 0.7 3020 10 7 3 0.7 3021 10 3 7 0.3 3022 10 6 4 0.6 3023 10 6 4 0.6 3024 10 5 5 0.5 3025 10 3 7 0.3 3026 10 4 6 0.4 3027 10 4 6 0.4 3028 10 6 4 0.6 3029 10 5 5 0.5 3030 10 4 6 0.4 3031 10 7 3 0.7 3032 10 4 6 0.4 3033 10 5 5 0.5 3034 10 7 3 0.7 3035 10 8 2 0.8 3036 10 4 6 0.4 3037 10 7 3 0.7 3038 10 4 6 0.4 3039 10 7 3 0.7 3040 10 5 5 0.5 3041 10 6 4 0.6 3042 10 3 7 0.3 3043 10 5 5 0.5 3044 10 4 6 0.4 3045 10 7 3 0.7 3046 10 7 3 0.7 3047 10 4 6 0.4 3048 10 7 3 0.7 3049 10 3 7 0.3 3050 10 5 5 0.5 3051 10 4 6 0.4 3052 10 5 5 0.5 3053 10 4 6 0.4 3054 10 6 4 0.6 3055 10 4 6 0.4 3056 10 6 4 0.6 3057 10 5 5 0.5 3058 10 5 5 0.5 3059 10 4 6 0.4 3060 10 6 4 0.6 3061 10 5 5 0.5 3062 10 6 4 0.6 3063 10 7 3 0.7 3064 10 4 6 0.4 3065 10 6 4 0.6 3066 10 3 7 0.3 3067 10 3 7 0.3 3068 10 3 7 0.3 3069 10 2 8 0.2 3070 10 5 5 0.5 3071 10 8 2 0.8 3072 10 8 2 0.8 3073 10 8 2 0.8 3074 10 4 6 0.4 3075 10 6 4 0.6 3076 10 6 4 0.6 3077 10 3 7 0.3 3078 10 5 5 0.5 3079 10 5 5 0.5 3080 10 4 6 0.4 3081 10 3 7 0.3 3082 10 2 8 0.2 3083 10 4 6 0.4 3084 10 6 4 0.6 3085 10 5 5 0.5 3086 10 6 4 0.6 3087 10 5 5 0.5 3088 10 5 5 0.5 3089 10 7 3 0.7 3090 10 3 7 0.3 3091 10 5 5 0.5 3092 10 4 6 0.4 3093 10 7 3 0.7 3094 10 5 5 0.5 3095 10 8 2 0.8 3096 10 7 3 0.7 3097 10 2 8 0.2 3098 10 5 5 0.5 3099 10 7 3 0.7 3100 10 5 5 0.5 3101 10 4 6 0.4 3102 10 5 5 0.5 3103 10 2 8 0.2 3104 10 7 3 0.7 3105 10 4 6 0.4 3106 10 6 4 0.6 3107 10 4 6 0.4 3108 10 6 4 0.6 3109 10 4 6 0.4 3110 10 7 3 0.7 3111 10 4 6 0.4 3112 10 6 4 0.6 3113 10 6 4 0.6 3114 10 7 3 0.7 3115 10 5 5 0.5 3116 10 8 2 0.8 3117 10 3 7 0.3 3118 10 2 8 0.2 3119 10 2 8 0.2 3120 10 4 6 0.4 3121 10 6 4 0.6 3122 10 5 5 0.5 3123 10 8 2 0.8 3124 10 3 7 0.3 3125 10 4 6 0.4 3126 10 5 5 0.5 3127 10 5 5 0.5 3128 10 6 4 0.6 3129 10 4 6 0.4 3130 10 6 4 0.6 3131 10 5 5 0.5 3132 10 3 7 0.3 3133 10 2 8 0.2 3134 10 7 3 0.7 3135 10 4 6 0.4 3136 10 6 4 0.6 3137 10 7 3 0.7 3138 10 3 7 0.3 3139 10 6 4 0.6 3140 10 2 8 0.2 3141 10 7 3 0.7 3142 10 5 5 0.5 3143 10 5 5 0.5 3144 10 4 6 0.4 3145 10 3 7 0.3 3146 10 7 3 0.7 3147 10 6 4 0.6 3148 10 3 7 0.3 3149 10 3 7 0.3 3150 10 5 5 0.5 3151 10 4 6 0.4 3152 10 6 4 0.6 3153 10 5 5 0.5 3154 10 7 3 0.7 3155 10 8 2 0.8 3156 10 5 5 0.5 3157 10 5 5 0.5 3158 10 5 5 0.5 3159 10 1 9 0.1 3160 10 8 2 0.8 3161 10 6 4 0.6 3162 10 6 4 0.6 3163 10 5 5 0.5 3164 10 5 5 0.5 3165 10 4 6 0.4 3166 10 6 4 0.6 3167 10 4 6 0.4 3168 10 5 5 0.5 3169 10 5 5 0.5 3170 10 7 3 0.7 3171 10 7 3 0.7 3172 10 6 4 0.6 3173 10 4 6 0.4 3174 10 6 4 0.6 3175 10 6 4 0.6 3176 10 4 6 0.4 3177 10 7 3 0.7 3178 10 5 5 0.5 3179 10 4 6 0.4 3180 10 2 8 0.2 3181 10 4 6 0.4 3182 10 6 4 0.6 3183 10 5 5 0.5 3184 10 2 8 0.2 3185 10 6 4 0.6 3186 10 5 5 0.5 3187 10 3 7 0.3 3188 10 4 6 0.4 3189 10 7 3 0.7 3190 10 4 6 0.4 3191 10 4 6 0.4 3192 10 4 6 0.4 3193 10 3 7 0.3 3194 10 3 7 0.3 3195 10 7 3 0.7 3196 10 6 4 0.6 3197 10 6 4 0.6 3198 10 3 7 0.3 3199 10 3 7 0.3 3200 10 3 7 0.3 3201 10 4 6 0.4 3202 10 8 2 0.8 3203 10 4 6 0.4 3204 10 7 3 0.7 3205 10 3 7 0.3 3206 10 4 6 0.4 3207 10 5 5 0.5 3208 10 6 4 0.6 3209 10 6 4 0.6 3210 10 4 6 0.4 3211 10 2 8 0.2 3212 10 5 5 0.5 3213 10 5 5 0.5 3214 10 5 5 0.5 3215 10 4 6 0.4 3216 10 6 4 0.6 3217 10 5 5 0.5 3218 10 7 3 0.7 3219 10 5 5 0.5 3220 10 4 6 0.4 3221 10 6 4 0.6 3222 10 5 5 0.5 3223 10 3 7 0.3 3224 10 5 5 0.5 3225 10 6 4 0.6 3226 10 5 5 0.5 3227 10 5 5 0.5 3228 10 3 7 0.3 3229 10 5 5 0.5 3230 10 6 4 0.6 3231 10 4 6 0.4 3232 10 4 6 0.4 3233 10 1 9 0.1 3234 10 4 6 0.4 3235 10 7 3 0.7 3236 10 6 4 0.6 3237 10 8 2 0.8 3238 10 5 5 0.5 3239 10 7 3 0.7 3240 10 5 5 0.5 3241 10 4 6 0.4 3242 10 6 4 0.6 3243 10 3 7 0.3 3244 10 6 4 0.6 3245 10 2 8 0.2 3246 10 6 4 0.6 3247 10 4 6 0.4 3248 10 5 5 0.5 3249 10 8 2 0.8 3250 10 7 3 0.7 3251 10 4 6 0.4 3252 10 7 3 0.7 3253 10 5 5 0.5 3254 10 7 3 0.7 3255 10 4 6 0.4 3256 10 7 3 0.7 3257 10 7 3 0.7 3258 10 5 5 0.5 3259 10 5 5 0.5 3260 10 1 9 0.1 3261 10 5 5 0.5 3262 10 2 8 0.2 3263 10 4 6 0.4 3264 10 6 4 0.6 3265 10 5 5 0.5 3266 10 6 4 0.6 3267 10 7 3 0.7 3268 10 6 4 0.6 3269 10 4 6 0.4 3270 10 6 4 0.6 3271 10 6 4 0.6 3272 10 6 4 0.6 3273 10 4 6 0.4 3274 10 6 4 0.6 3275 10 4 6 0.4 3276 10 4 6 0.4 3277 10 7 3 0.7 3278 10 8 2 0.8 3279 10 8 2 0.8 3280 10 5 5 0.5 3281 10 1 9 0.1 3282 10 5 5 0.5 3283 10 6 4 0.6 3284 10 6 4 0.6 3285 10 8 2 0.8 3286 10 4 6 0.4 3287 10 5 5 0.5 3288 10 3 7 0.3 3289 10 3 7 0.3 3290 10 4 6 0.4 3291 10 5 5 0.5 3292 10 5 5 0.5 3293 10 2 8 0.2 3294 10 7 3 0.7 3295 10 9 1 0.9 3296 10 8 2 0.8 3297 10 6 4 0.6 3298 10 6 4 0.6 3299 10 5 5 0.5 3300 10 4 6 0.4 3301 10 7 3 0.7 3302 10 4 6 0.4 3303 10 4 6 0.4 3304 10 5 5 0.5 3305 10 6 4 0.6 3306 10 7 3 0.7 3307 10 5 5 0.5 3308 10 3 7 0.3 3309 10 4 6 0.4 3310 10 6 4 0.6 3311 10 3 7 0.3 3312 10 5 5 0.5 3313 10 4 6 0.4 3314 10 7 3 0.7 3315 10 6 4 0.6 3316 10 5 5 0.5 3317 10 5 5 0.5 3318 10 5 5 0.5 3319 10 7 3 0.7 3320 10 4 6 0.4 3321 10 6 4 0.6 3322 10 7 3 0.7 3323 10 5 5 0.5 3324 10 5 5 0.5 3325 10 4 6 0.4 3326 10 8 2 0.8 3327 10 4 6 0.4 3328 10 6 4 0.6 3329 10 4 6 0.4 3330 10 5 5 0.5 3331 10 4 6 0.4 3332 10 4 6 0.4 3333 10 5 5 0.5 3334 10 5 5 0.5 3335 10 4 6 0.4 3336 10 5 5 0.5 3337 10 5 5 0.5 3338 10 4 6 0.4 3339 10 5 5 0.5 3340 10 3 7 0.3 3341 10 9 1 0.9 3342 10 6 4 0.6 3343 10 4 6 0.4 3344 10 2 8 0.2 3345 10 3 7 0.3 3346 10 6 4 0.6 3347 10 7 3 0.7 3348 10 5 5 0.5 3349 10 7 3 0.7 3350 10 6 4 0.6 3351 10 5 5 0.5 3352 10 6 4 0.6 3353 10 7 3 0.7 3354 10 4 6 0.4 3355 10 4 6 0.4 3356 10 5 5 0.5 3357 10 6 4 0.6 3358 10 5 5 0.5 3359 10 7 3 0.7 3360 10 5 5 0.5 3361 10 5 5 0.5 3362 10 6 4 0.6 3363 10 3 7 0.3 3364 10 6 4 0.6 3365 10 3 7 0.3 3366 10 5 5 0.5 3367 10 4 6 0.4 3368 10 6 4 0.6 3369 10 8 2 0.8 3370 10 5 5 0.5 3371 10 3 7 0.3 3372 10 5 5 0.5 3373 10 5 5 0.5 3374 10 4 6 0.4 3375 10 5 5 0.5 3376 10 5 5 0.5 3377 10 7 3 0.7 3378 10 3 7 0.3 3379 10 4 6 0.4 3380 10 6 4 0.6 3381 10 3 7 0.3 3382 10 6 4 0.6 3383 10 7 3 0.7 3384 10 4 6 0.4 3385 10 6 4 0.6 3386 10 7 3 0.7 3387 10 4 6 0.4 3388 10 4 6 0.4 3389 10 4 6 0.4 3390 10 3 7 0.3 3391 10 6 4 0.6 3392 10 6 4 0.6 3393 10 1 9 0.1 3394 10 6 4 0.6 3395 10 3 7 0.3 3396 10 7 3 0.7 3397 10 4 6 0.4 3398 10 7 3 0.7 3399 10 6 4 0.6 3400 10 4 6 0.4 3401 10 3 7 0.3 3402 10 4 6 0.4 3403 10 2 8 0.2 3404 10 3 7 0.3 3405 10 4 6 0.4 3406 10 5 5 0.5 3407 10 3 7 0.3 3408 10 7 3 0.7 3409 10 4 6 0.4 3410 10 6 4 0.6 3411 10 2 8 0.2 3412 10 6 4 0.6 3413 10 3 7 0.3 3414 10 4 6 0.4 3415 10 4 6 0.4 3416 10 4 6 0.4 3417 10 6 4 0.6 3418 10 5 5 0.5 3419 10 3 7 0.3 3420 10 5 5 0.5 3421 10 5 5 0.5 3422 10 5 5 0.5 3423 10 4 6 0.4 3424 10 5 5 0.5 3425 10 5 5 0.5 3426 10 7 3 0.7 3427 10 3 7 0.3 3428 10 6 4 0.6 3429 10 8 2 0.8 3430 10 2 8 0.2 3431 10 6 4 0.6 3432 10 4 6 0.4 3433 10 3 7 0.3 3434 10 3 7 0.3 3435 10 5 5 0.5 3436 10 4 6 0.4 3437 10 7 3 0.7 3438 10 3 7 0.3 3439 10 5 5 0.5 3440 10 6 4 0.6 3441 10 4 6 0.4 3442 10 5 5 0.5 3443 10 4 6 0.4 3444 10 6 4 0.6 3445 10 1 9 0.1 3446 10 5 5 0.5 3447 10 8 2 0.8 3448 10 7 3 0.7 3449 10 4 6 0.4 3450 10 3 7 0.3 3451 10 6 4 0.6 3452 10 3 7 0.3 3453 10 3 7 0.3 3454 10 6 4 0.6 3455 10 5 5 0.5 3456 10 2 8 0.2 3457 10 6 4 0.6 3458 10 4 6 0.4 3459 10 6 4 0.6 3460 10 6 4 0.6 3461 10 4 6 0.4 3462 10 6 4 0.6 3463 10 4 6 0.4 3464 10 7 3 0.7 3465 10 7 3 0.7 3466 10 1 9 0.1 3467 10 5 5 0.5 3468 10 4 6 0.4 3469 10 4 6 0.4 3470 10 3 7 0.3 3471 10 6 4 0.6 3472 10 5 5 0.5 3473 10 3 7 0.3 3474 10 6 4 0.6 3475 10 6 4 0.6 3476 10 6 4 0.6 3477 10 6 4 0.6 3478 10 8 2 0.8 3479 10 5 5 0.5 3480 10 3 7 0.3 3481 10 5 5 0.5 3482 10 10 0 1.0 3483 10 4 6 0.4 3484 10 3 7 0.3 3485 10 6 4 0.6 3486 10 6 4 0.6 3487 10 5 5 0.5 3488 10 7 3 0.7 3489 10 4 6 0.4 3490 10 5 5 0.5 3491 10 4 6 0.4 3492 10 2 8 0.2 3493 10 5 5 0.5 3494 10 5 5 0.5 3495 10 4 6 0.4 3496 10 5 5 0.5 3497 10 7 3 0.7 3498 10 7 3 0.7 3499 10 5 5 0.5 3500 10 4 6 0.4 3501 10 7 3 0.7 3502 10 7 3 0.7 3503 10 7 3 0.7 3504 10 5 5 0.5 3505 10 6 4 0.6 3506 10 6 4 0.6 3507 10 5 5 0.5 3508 10 7 3 0.7 3509 10 9 1 0.9 3510 10 6 4 0.6 3511 10 5 5 0.5 3512 10 5 5 0.5 3513 10 4 6 0.4 3514 10 8 2 0.8 3515 10 5 5 0.5 3516 10 4 6 0.4 3517 10 5 5 0.5 3518 10 7 3 0.7 3519 10 5 5 0.5 3520 10 6 4 0.6 3521 10 5 5 0.5 3522 10 5 5 0.5 3523 10 6 4 0.6 3524 10 7 3 0.7 3525 10 4 6 0.4 3526 10 5 5 0.5 3527 10 2 8 0.2 3528 10 5 5 0.5 3529 10 4 6 0.4 3530 10 4 6 0.4 3531 10 4 6 0.4 3532 10 4 6 0.4 3533 10 5 5 0.5 3534 10 5 5 0.5 3535 10 8 2 0.8 3536 10 4 6 0.4 3537 10 5 5 0.5 3538 10 5 5 0.5 3539 10 6 4 0.6 3540 10 3 7 0.3 3541 10 9 1 0.9 3542 10 4 6 0.4 3543 10 5 5 0.5 3544 10 6 4 0.6 3545 10 6 4 0.6 3546 10 6 4 0.6 3547 10 7 3 0.7 3548 10 4 6 0.4 3549 10 5 5 0.5 3550 10 3 7 0.3 3551 10 3 7 0.3 3552 10 5 5 0.5 3553 10 5 5 0.5 3554 10 4 6 0.4 3555 10 8 2 0.8 3556 10 5 5 0.5 3557 10 7 3 0.7 3558 10 2 8 0.2 3559 10 4 6 0.4 3560 10 4 6 0.4 3561 10 6 4 0.6 3562 10 4 6 0.4 3563 10 5 5 0.5 3564 10 6 4 0.6 3565 10 8 2 0.8 3566 10 7 3 0.7 3567 10 6 4 0.6 3568 10 7 3 0.7 3569 10 6 4 0.6 3570 10 3 7 0.3 3571 10 2 8 0.2 3572 10 3 7 0.3 3573 10 6 4 0.6 3574 10 4 6 0.4 3575 10 6 4 0.6 3576 10 5 5 0.5 3577 10 4 6 0.4 3578 10 5 5 0.5 3579 10 6 4 0.6 3580 10 3 7 0.3 3581 10 4 6 0.4 3582 10 9 1 0.9 3583 10 5 5 0.5 3584 10 7 3 0.7 3585 10 3 7 0.3 3586 10 5 5 0.5 3587 10 4 6 0.4 3588 10 5 5 0.5 3589 10 5 5 0.5 3590 10 7 3 0.7 3591 10 3 7 0.3 3592 10 3 7 0.3 3593 10 4 6 0.4 3594 10 7 3 0.7 3595 10 6 4 0.6 3596 10 6 4 0.6 3597 10 4 6 0.4 3598 10 4 6 0.4 3599 10 4 6 0.4 3600 10 7 3 0.7 3601 10 6 4 0.6 3602 10 5 5 0.5 3603 10 5 5 0.5 3604 10 4 6 0.4 3605 10 6 4 0.6 3606 10 6 4 0.6 3607 10 7 3 0.7 3608 10 6 4 0.6 3609 10 6 4 0.6 3610 10 2 8 0.2 3611 10 4 6 0.4 3612 10 7 3 0.7 3613 10 7 3 0.7 3614 10 6 4 0.6 3615 10 7 3 0.7 3616 10 7 3 0.7 3617 10 5 5 0.5 3618 10 4 6 0.4 3619 10 4 6 0.4 3620 10 4 6 0.4 3621 10 5 5 0.5 3622 10 5 5 0.5 3623 10 3 7 0.3 3624 10 3 7 0.3 3625 10 3 7 0.3 3626 10 8 2 0.8 3627 10 5 5 0.5 3628 10 6 4 0.6 3629 10 8 2 0.8 3630 10 5 5 0.5 3631 10 3 7 0.3 3632 10 6 4 0.6 3633 10 5 5 0.5 3634 10 1 9 0.1 3635 10 5 5 0.5 3636 10 6 4 0.6 3637 10 4 6 0.4 3638 10 4 6 0.4 3639 10 5 5 0.5 3640 10 6 4 0.6 3641 10 5 5 0.5 3642 10 5 5 0.5 3643 10 9 1 0.9 3644 10 6 4 0.6 3645 10 3 7 0.3 3646 10 6 4 0.6 3647 10 7 3 0.7 3648 10 5 5 0.5 3649 10 4 6 0.4 3650 10 5 5 0.5 3651 10 6 4 0.6 3652 10 4 6 0.4 3653 10 3 7 0.3 3654 10 6 4 0.6 3655 10 5 5 0.5 3656 10 6 4 0.6 3657 10 5 5 0.5 3658 10 4 6 0.4 3659 10 5 5 0.5 3660 10 4 6 0.4 3661 10 7 3 0.7 3662 10 7 3 0.7 3663 10 5 5 0.5 3664 10 5 5 0.5 3665 10 6 4 0.6 3666 10 7 3 0.7 3667 10 5 5 0.5 3668 10 3 7 0.3 3669 10 6 4 0.6 3670 10 5 5 0.5 3671 10 6 4 0.6 3672 10 8 2 0.8 3673 10 3 7 0.3 3674 10 6 4 0.6 3675 10 6 4 0.6 3676 10 4 6 0.4 3677 10 4 6 0.4 3678 10 4 6 0.4 3679 10 2 8 0.2 3680 10 6 4 0.6 3681 10 4 6 0.4 3682 10 4 6 0.4 3683 10 5 5 0.5 3684 10 4 6 0.4 3685 10 5 5 0.5 3686 10 7 3 0.7 3687 10 6 4 0.6 3688 10 4 6 0.4 3689 10 6 4 0.6 3690 10 6 4 0.6 3691 10 7 3 0.7 3692 10 3 7 0.3 3693 10 6 4 0.6 3694 10 4 6 0.4 3695 10 4 6 0.4 3696 10 4 6 0.4 3697 10 7 3 0.7 3698 10 6 4 0.6 3699 10 4 6 0.4 3700 10 6 4 0.6 3701 10 2 8 0.2 3702 10 5 5 0.5 3703 10 6 4 0.6 3704 10 5 5 0.5 3705 10 4 6 0.4 3706 10 6 4 0.6 3707 10 2 8 0.2 3708 10 2 8 0.2 3709 10 6 4 0.6 3710 10 4 6 0.4 3711 10 4 6 0.4 3712 10 6 4 0.6 3713 10 6 4 0.6 3714 10 1 9 0.1 3715 10 6 4 0.6 3716 10 3 7 0.3 3717 10 6 4 0.6 3718 10 6 4 0.6 3719 10 4 6 0.4 3720 10 5 5 0.5 3721 10 5 5 0.5 3722 10 5 5 0.5 3723 10 7 3 0.7 3724 10 5 5 0.5 3725 10 4 6 0.4 3726 10 5 5 0.5 3727 10 4 6 0.4 3728 10 4 6 0.4 3729 10 7 3 0.7 3730 10 4 6 0.4 3731 10 2 8 0.2 3732 10 4 6 0.4 3733 10 3 7 0.3 3734 10 6 4 0.6 3735 10 8 2 0.8 3736 10 5 5 0.5 3737 10 3 7 0.3 3738 10 5 5 0.5 3739 10 8 2 0.8 3740 10 4 6 0.4 3741 10 7 3 0.7 3742 10 8 2 0.8 3743 10 3 7 0.3 3744 10 3 7 0.3 3745 10 4 6 0.4 3746 10 3 7 0.3 3747 10 4 6 0.4 3748 10 6 4 0.6 3749 10 5 5 0.5 3750 10 5 5 0.5 3751 10 6 4 0.6 3752 10 5 5 0.5 3753 10 4 6 0.4 3754 10 4 6 0.4 3755 10 7 3 0.7 3756 10 6 4 0.6 3757 10 4 6 0.4 3758 10 7 3 0.7 3759 10 3 7 0.3 3760 10 9 1 0.9 3761 10 6 4 0.6 3762 10 3 7 0.3 3763 10 3 7 0.3 3764 10 4 6 0.4 3765 10 3 7 0.3 3766 10 5 5 0.5 3767 10 3 7 0.3 3768 10 9 1 0.9 3769 10 2 8 0.2 3770 10 5 5 0.5 3771 10 7 3 0.7 3772 10 6 4 0.6 3773 10 5 5 0.5 3774 10 4 6 0.4 3775 10 2 8 0.2 3776 10 7 3 0.7 3777 10 9 1 0.9 3778 10 5 5 0.5 3779 10 5 5 0.5 3780 10 5 5 0.5 3781 10 4 6 0.4 3782 10 5 5 0.5 3783 10 7 3 0.7 3784 10 4 6 0.4 3785 10 5 5 0.5 3786 10 4 6 0.4 3787 10 3 7 0.3 3788 10 5 5 0.5 3789 10 7 3 0.7 3790 10 6 4 0.6 3791 10 4 6 0.4 3792 10 5 5 0.5 3793 10 6 4 0.6 3794 10 2 8 0.2 3795 10 6 4 0.6 3796 10 6 4 0.6 3797 10 4 6 0.4 3798 10 8 2 0.8 3799 10 6 4 0.6 3800 10 7 3 0.7 3801 10 4 6 0.4 3802 10 3 7 0.3 3803 10 7 3 0.7 3804 10 3 7 0.3 3805 10 6 4 0.6 3806 10 8 2 0.8 3807 10 6 4 0.6 3808 10 4 6 0.4 3809 10 4 6 0.4 3810 10 6 4 0.6 3811 10 4 6 0.4 3812 10 3 7 0.3 3813 10 4 6 0.4 3814 10 7 3 0.7 3815 10 8 2 0.8 3816 10 5 5 0.5 3817 10 3 7 0.3 3818 10 3 7 0.3 3819 10 6 4 0.6 3820 10 4 6 0.4 3821 10 5 5 0.5 3822 10 5 5 0.5 3823 10 4 6 0.4 3824 10 5 5 0.5 3825 10 7 3 0.7 3826 10 4 6 0.4 3827 10 2 8 0.2 3828 10 5 5 0.5 3829 10 7 3 0.7 3830 10 8 2 0.8 3831 10 4 6 0.4 3832 10 5 5 0.5 3833 10 5 5 0.5 3834 10 4 6 0.4 3835 10 4 6 0.4 3836 10 6 4 0.6 3837 10 5 5 0.5 3838 10 4 6 0.4 3839 10 5 5 0.5 3840 10 5 5 0.5 3841 10 3 7 0.3 3842 10 3 7 0.3 3843 10 7 3 0.7 3844 10 5 5 0.5 3845 10 3 7 0.3 3846 10 3 7 0.3 3847 10 8 2 0.8 3848 10 6 4 0.6 3849 10 6 4 0.6 3850 10 3 7 0.3 3851 10 4 6 0.4 3852 10 5 5 0.5 3853 10 5 5 0.5 3854 10 4 6 0.4 3855 10 5 5 0.5 3856 10 7 3 0.7 3857 10 5 5 0.5 3858 10 3 7 0.3 3859 10 4 6 0.4 3860 10 5 5 0.5 3861 10 5 5 0.5 3862 10 6 4 0.6 3863 10 8 2 0.8 3864 10 6 4 0.6 3865 10 3 7 0.3 3866 10 3 7 0.3 3867 10 5 5 0.5 3868 10 7 3 0.7 3869 10 6 4 0.6 3870 10 4 6 0.4 3871 10 5 5 0.5 3872 10 6 4 0.6 3873 10 7 3 0.7 3874 10 5 5 0.5 3875 10 4 6 0.4 3876 10 6 4 0.6 3877 10 5 5 0.5 3878 10 7 3 0.7 3879 10 6 4 0.6 3880 10 4 6 0.4 3881 10 6 4 0.6 3882 10 7 3 0.7 3883 10 6 4 0.6 3884 10 7 3 0.7 3885 10 7 3 0.7 3886 10 6 4 0.6 3887 10 4 6 0.4 3888 10 3 7 0.3 3889 10 6 4 0.6 3890 10 5 5 0.5 3891 10 5 5 0.5 3892 10 7 3 0.7 3893 10 6 4 0.6 3894 10 6 4 0.6 3895 10 6 4 0.6 3896 10 5 5 0.5 3897 10 5 5 0.5 3898 10 5 5 0.5 3899 10 2 8 0.2 3900 10 5 5 0.5 3901 10 4 6 0.4 3902 10 8 2 0.8 3903 10 7 3 0.7 3904 10 4 6 0.4 3905 10 4 6 0.4 3906 10 8 2 0.8 3907 10 4 6 0.4 3908 10 7 3 0.7 3909 10 4 6 0.4 3910 10 5 5 0.5 3911 10 3 7 0.3 3912 10 5 5 0.5 3913 10 6 4 0.6 3914 10 4 6 0.4 3915 10 4 6 0.4 3916 10 7 3 0.7 3917 10 6 4 0.6 3918 10 5 5 0.5 3919 10 5 5 0.5 3920 10 5 5 0.5 3921 10 4 6 0.4 3922 10 4 6 0.4 3923 10 4 6 0.4 3924 10 6 4 0.6 3925 10 4 6 0.4 3926 10 3 7 0.3 3927 10 3 7 0.3 3928 10 5 5 0.5 3929 10 6 4 0.6 3930 10 6 4 0.6 3931 10 4 6 0.4 3932 10 4 6 0.4 3933 10 6 4 0.6 3934 10 4 6 0.4 3935 10 4 6 0.4 3936 10 7 3 0.7 3937 10 3 7 0.3 3938 10 6 4 0.6 3939 10 5 5 0.5 3940 10 5 5 0.5 3941 10 4 6 0.4 3942 10 6 4 0.6 3943 10 6 4 0.6 3944 10 4 6 0.4 3945 10 8 2 0.8 3946 10 4 6 0.4 3947 10 7 3 0.7 3948 10 7 3 0.7 3949 10 5 5 0.5 3950 10 2 8 0.2 3951 10 7 3 0.7 3952 10 4 6 0.4 3953 10 2 8 0.2 3954 10 5 5 0.5 3955 10 2 8 0.2 3956 10 4 6 0.4 3957 10 4 6 0.4 3958 10 7 3 0.7 3959 10 3 7 0.3 3960 10 4 6 0.4 3961 10 6 4 0.6 3962 10 8 2 0.8 3963 10 5 5 0.5 3964 10 5 5 0.5 3965 10 5 5 0.5 3966 10 8 2 0.8 3967 10 4 6 0.4 3968 10 5 5 0.5 3969 10 5 5 0.5 3970 10 6 4 0.6 3971 10 8 2 0.8 3972 10 4 6 0.4 3973 10 3 7 0.3 3974 10 4 6 0.4 3975 10 4 6 0.4 3976 10 4 6 0.4 3977 10 6 4 0.6 3978 10 3 7 0.3 3979 10 4 6 0.4 3980 10 7 3 0.7 3981 10 6 4 0.6 3982 10 5 5 0.5 3983 10 6 4 0.6 3984 10 6 4 0.6 3985 10 6 4 0.6 3986 10 4 6 0.4 3987 10 5 5 0.5 3988 10 7 3 0.7 3989 10 4 6 0.4 3990 10 8 2 0.8 3991 10 3 7 0.3 3992 10 4 6 0.4 3993 10 2 8 0.2 3994 10 5 5 0.5 3995 10 5 5 0.5 3996 10 8 2 0.8 3997 10 3 7 0.3 3998 10 2 8 0.2 3999 10 4 6 0.4 4000 10 7 3 0.7 4001 10 5 5 0.5 4002 10 3 7 0.3 4003 10 5 5 0.5 4004 10 7 3 0.7 4005 10 4 6 0.4 4006 10 8 2 0.8 4007 10 4 6 0.4 4008 10 4 6 0.4 4009 10 2 8 0.2 4010 10 4 6 0.4 4011 10 4 6 0.4 4012 10 8 2 0.8 4013 10 2 8 0.2 4014 10 8 2 0.8 4015 10 3 7 0.3 4016 10 7 3 0.7 4017 10 6 4 0.6 4018 10 3 7 0.3 4019 10 3 7 0.3 4020 10 4 6 0.4 4021 10 2 8 0.2 4022 10 6 4 0.6 4023 10 5 5 0.5 4024 10 4 6 0.4 4025 10 5 5 0.5 4026 10 5 5 0.5 4027 10 6 4 0.6 4028 10 3 7 0.3 4029 10 6 4 0.6 4030 10 6 4 0.6 4031 10 5 5 0.5 4032 10 4 6 0.4 4033 10 7 3 0.7 4034 10 10 0 1.0 4035 10 2 8 0.2 4036 10 5 5 0.5 4037 10 5 5 0.5 4038 10 5 5 0.5 4039 10 6 4 0.6 4040 10 5 5 0.5 4041 10 6 4 0.6 4042 10 4 6 0.4 4043 10 6 4 0.6 4044 10 8 2 0.8 4045 10 6 4 0.6 4046 10 3 7 0.3 4047 10 7 3 0.7 4048 10 5 5 0.5 4049 10 3 7 0.3 4050 10 6 4 0.6 4051 10 5 5 0.5 4052 10 8 2 0.8 4053 10 6 4 0.6 4054 10 5 5 0.5 4055 10 5 5 0.5 4056 10 5 5 0.5 4057 10 6 4 0.6 4058 10 7 3 0.7 4059 10 5 5 0.5 4060 10 6 4 0.6 4061 10 10 0 1.0 4062 10 4 6 0.4 4063 10 7 3 0.7 4064 10 7 3 0.7 4065 10 7 3 0.7 4066 10 3 7 0.3 4067 10 1 9 0.1 4068 10 4 6 0.4 4069 10 6 4 0.6 4070 10 6 4 0.6 4071 10 6 4 0.6 4072 10 4 6 0.4 4073 10 7 3 0.7 4074 10 6 4 0.6 4075 10 7 3 0.7 4076 10 5 5 0.5 4077 10 4 6 0.4 4078 10 5 5 0.5 4079 10 6 4 0.6 4080 10 3 7 0.3 4081 10 5 5 0.5 4082 10 6 4 0.6 4083 10 4 6 0.4 4084 10 6 4 0.6 4085 10 6 4 0.6 4086 10 6 4 0.6 4087 10 5 5 0.5 4088 10 3 7 0.3 4089 10 4 6 0.4 4090 10 4 6 0.4 4091 10 6 4 0.6 4092 10 3 7 0.3 4093 10 4 6 0.4 4094 10 5 5 0.5 4095 10 6 4 0.6 4096 10 4 6 0.4 4097 10 5 5 0.5 4098 10 6 4 0.6 4099 10 4 6 0.4 4100 10 5 5 0.5 4101 10 5 5 0.5 4102 10 5 5 0.5 4103 10 5 5 0.5 4104 10 7 3 0.7 4105 10 3 7 0.3 4106 10 5 5 0.5 4107 10 4 6 0.4 4108 10 5 5 0.5 4109 10 4 6 0.4 4110 10 6 4 0.6 4111 10 3 7 0.3 4112 10 4 6 0.4 4113 10 7 3 0.7 4114 10 8 2 0.8 4115 10 3 7 0.3 4116 10 5 5 0.5 4117 10 7 3 0.7 4118 10 5 5 0.5 4119 10 5 5 0.5 4120 10 5 5 0.5 4121 10 5 5 0.5 4122 10 4 6 0.4 4123 10 6 4 0.6 4124 10 5 5 0.5 4125 10 7 3 0.7 4126 10 5 5 0.5 4127 10 5 5 0.5 4128 10 5 5 0.5 4129 10 4 6 0.4 4130 10 5 5 0.5 4131 10 4 6 0.4 4132 10 7 3 0.7 4133 10 7 3 0.7 4134 10 8 2 0.8 4135 10 5 5 0.5 4136 10 6 4 0.6 4137 10 5 5 0.5 4138 10 5 5 0.5 4139 10 5 5 0.5 4140 10 7 3 0.7 4141 10 4 6 0.4 4142 10 5 5 0.5 4143 10 4 6 0.4 4144 10 6 4 0.6 4145 10 6 4 0.6 4146 10 3 7 0.3 4147 10 5 5 0.5 4148 10 6 4 0.6 4149 10 5 5 0.5 4150 10 2 8 0.2 4151 10 9 1 0.9 4152 10 2 8 0.2 4153 10 4 6 0.4 4154 10 2 8 0.2 4155 10 4 6 0.4 4156 10 4 6 0.4 4157 10 6 4 0.6 4158 10 4 6 0.4 4159 10 4 6 0.4 4160 10 5 5 0.5 4161 10 5 5 0.5 4162 10 3 7 0.3 4163 10 4 6 0.4 4164 10 5 5 0.5 4165 10 5 5 0.5 4166 10 6 4 0.6 4167 10 6 4 0.6 4168 10 3 7 0.3 4169 10 5 5 0.5 4170 10 6 4 0.6 4171 10 6 4 0.6 4172 10 2 8 0.2 4173 10 4 6 0.4 4174 10 7 3 0.7 4175 10 3 7 0.3 4176 10 7 3 0.7 4177 10 3 7 0.3 4178 10 2 8 0.2 4179 10 6 4 0.6 4180 10 7 3 0.7 4181 10 7 3 0.7 4182 10 5 5 0.5 4183 10 6 4 0.6 4184 10 5 5 0.5 4185 10 6 4 0.6 4186 10 6 4 0.6 4187 10 8 2 0.8 4188 10 6 4 0.6 4189 10 6 4 0.6 4190 10 7 3 0.7 4191 10 4 6 0.4 4192 10 6 4 0.6 4193 10 6 4 0.6 4194 10 4 6 0.4 4195 10 5 5 0.5 4196 10 5 5 0.5 4197 10 4 6 0.4 4198 10 2 8 0.2 4199 10 4 6 0.4 4200 10 2 8 0.2 4201 10 6 4 0.6 4202 10 6 4 0.6 4203 10 8 2 0.8 4204 10 8 2 0.8 4205 10 7 3 0.7 4206 10 7 3 0.7 4207 10 3 7 0.3 4208 10 5 5 0.5 4209 10 5 5 0.5 4210 10 5 5 0.5 4211 10 8 2 0.8 4212 10 6 4 0.6 4213 10 5 5 0.5 4214 10 6 4 0.6 4215 10 3 7 0.3 4216 10 4 6 0.4 4217 10 4 6 0.4 4218 10 5 5 0.5 4219 10 6 4 0.6 4220 10 5 5 0.5 4221 10 3 7 0.3 4222 10 4 6 0.4 4223 10 4 6 0.4 4224 10 6 4 0.6 4225 10 4 6 0.4 4226 10 6 4 0.6 4227 10 6 4 0.6 4228 10 5 5 0.5 4229 10 4 6 0.4 4230 10 6 4 0.6 4231 10 1 9 0.1 4232 10 4 6 0.4 4233 10 3 7 0.3 4234 10 7 3 0.7 4235 10 3 7 0.3 4236 10 6 4 0.6 4237 10 6 4 0.6 4238 10 3 7 0.3 4239 10 3 7 0.3 4240 10 4 6 0.4 4241 10 5 5 0.5 4242 10 4 6 0.4 4243 10 3 7 0.3 4244 10 4 6 0.4 4245 10 5 5 0.5 4246 10 3 7 0.3 4247 10 4 6 0.4 4248 10 6 4 0.6 4249 10 5 5 0.5 4250 10 6 4 0.6 4251 10 5 5 0.5 4252 10 4 6 0.4 4253 10 4 6 0.4 4254 10 6 4 0.6 4255 10 4 6 0.4 4256 10 3 7 0.3 4257 10 6 4 0.6 4258 10 1 9 0.1 4259 10 4 6 0.4 4260 10 3 7 0.3 4261 10 7 3 0.7 4262 10 4 6 0.4 4263 10 7 3 0.7 4264 10 9 1 0.9 4265 10 6 4 0.6 4266 10 3 7 0.3 4267 10 5 5 0.5 4268 10 10 0 1.0 4269 10 7 3 0.7 4270 10 5 5 0.5 4271 10 3 7 0.3 4272 10 6 4 0.6 4273 10 7 3 0.7 4274 10 4 6 0.4 4275 10 5 5 0.5 4276 10 8 2 0.8 4277 10 5 5 0.5 4278 10 6 4 0.6 4279 10 7 3 0.7 4280 10 5 5 0.5 4281 10 7 3 0.7 4282 10 4 6 0.4 4283 10 8 2 0.8 4284 10 7 3 0.7 4285 10 6 4 0.6 4286 10 3 7 0.3 4287 10 7 3 0.7 4288 10 3 7 0.3 4289 10 8 2 0.8 4290 10 3 7 0.3 4291 10 4 6 0.4 4292 10 4 6 0.4 4293 10 5 5 0.5 4294 10 4 6 0.4 4295 10 6 4 0.6 4296 10 5 5 0.5 4297 10 7 3 0.7 4298 10 5 5 0.5 4299 10 6 4 0.6 4300 10 3 7 0.3 4301 10 6 4 0.6 4302 10 9 1 0.9 4303 10 6 4 0.6 4304 10 4 6 0.4 4305 10 5 5 0.5 4306 10 7 3 0.7 4307 10 4 6 0.4 4308 10 4 6 0.4 4309 10 5 5 0.5 4310 10 6 4 0.6 4311 10 5 5 0.5 4312 10 4 6 0.4 4313 10 6 4 0.6 4314 10 5 5 0.5 4315 10 3 7 0.3 4316 10 7 3 0.7 4317 10 4 6 0.4 4318 10 8 2 0.8 4319 10 2 8 0.2 4320 10 5 5 0.5 4321 10 3 7 0.3 4322 10 4 6 0.4 4323 10 4 6 0.4 4324 10 3 7 0.3 4325 10 2 8 0.2 4326 10 1 9 0.1 4327 10 5 5 0.5 4328 10 5 5 0.5 4329 10 2 8 0.2 4330 10 5 5 0.5 4331 10 6 4 0.6 4332 10 5 5 0.5 4333 10 7 3 0.7 4334 10 3 7 0.3 4335 10 3 7 0.3 4336 10 6 4 0.6 4337 10 7 3 0.7 4338 10 6 4 0.6 4339 10 5 5 0.5 4340 10 3 7 0.3 4341 10 7 3 0.7 4342 10 4 6 0.4 4343 10 5 5 0.5 4344 10 7 3 0.7 4345 10 3 7 0.3 4346 10 7 3 0.7 4347 10 2 8 0.2 4348 10 7 3 0.7 4349 10 4 6 0.4 4350 10 8 2 0.8 4351 10 7 3 0.7 4352 10 6 4 0.6 4353 10 6 4 0.6 4354 10 6 4 0.6 4355 10 5 5 0.5 4356 10 1 9 0.1 4357 10 5 5 0.5 4358 10 4 6 0.4 4359 10 5 5 0.5 4360 10 8 2 0.8 4361 10 4 6 0.4 4362 10 2 8 0.2 4363 10 3 7 0.3 4364 10 6 4 0.6 4365 10 5 5 0.5 4366 10 5 5 0.5 4367 10 6 4 0.6 4368 10 6 4 0.6 4369 10 5 5 0.5 4370 10 4 6 0.4 4371 10 8 2 0.8 4372 10 5 5 0.5 4373 10 5 5 0.5 4374 10 7 3 0.7 4375 10 4 6 0.4 4376 10 5 5 0.5 4377 10 5 5 0.5 4378 10 5 5 0.5 4379 10 3 7 0.3 4380 10 3 7 0.3 4381 10 5 5 0.5 4382 10 2 8 0.2 4383 10 7 3 0.7 4384 10 9 1 0.9 4385 10 2 8 0.2 4386 10 5 5 0.5 4387 10 4 6 0.4 4388 10 7 3 0.7 4389 10 3 7 0.3 4390 10 7 3 0.7 4391 10 5 5 0.5 4392 10 9 1 0.9 4393 10 5 5 0.5 4394 10 4 6 0.4 4395 10 6 4 0.6 4396 10 6 4 0.6 4397 10 4 6 0.4 4398 10 4 6 0.4 4399 10 6 4 0.6 4400 10 4 6 0.4 4401 10 6 4 0.6 4402 10 4 6 0.4 4403 10 4 6 0.4 4404 10 5 5 0.5 4405 10 6 4 0.6 4406 10 5 5 0.5 4407 10 6 4 0.6 4408 10 5 5 0.5 4409 10 6 4 0.6 4410 10 5 5 0.5 4411 10 3 7 0.3 4412 10 6 4 0.6 4413 10 5 5 0.5 4414 10 7 3 0.7 4415 10 3 7 0.3 4416 10 6 4 0.6 4417 10 6 4 0.6 4418 10 5 5 0.5 4419 10 3 7 0.3 4420 10 8 2 0.8 4421 10 6 4 0.6 4422 10 5 5 0.5 4423 10 3 7 0.3 4424 10 2 8 0.2 4425 10 8 2 0.8 4426 10 4 6 0.4 4427 10 5 5 0.5 4428 10 7 3 0.7 4429 10 6 4 0.6 4430 10 3 7 0.3 4431 10 4 6 0.4 4432 10 6 4 0.6 4433 10 5 5 0.5 4434 10 6 4 0.6 4435 10 5 5 0.5 4436 10 6 4 0.6 4437 10 5 5 0.5 4438 10 6 4 0.6 4439 10 6 4 0.6 4440 10 6 4 0.6 4441 10 6 4 0.6 4442 10 1 9 0.1 4443 10 3 7 0.3 4444 10 9 1 0.9 4445 10 6 4 0.6 4446 10 5 5 0.5 4447 10 6 4 0.6 4448 10 6 4 0.6 4449 10 6 4 0.6 4450 10 4 6 0.4 4451 10 4 6 0.4 4452 10 9 1 0.9 4453 10 6 4 0.6 4454 10 5 5 0.5 4455 10 6 4 0.6 4456 10 7 3 0.7 4457 10 5 5 0.5 4458 10 5 5 0.5 4459 10 5 5 0.5 4460 10 7 3 0.7 4461 10 4 6 0.4 4462 10 6 4 0.6 4463 10 4 6 0.4 4464 10 5 5 0.5 4465 10 6 4 0.6 4466 10 7 3 0.7 4467 10 4 6 0.4 4468 10 1 9 0.1 4469 10 4 6 0.4 4470 10 6 4 0.6 4471 10 6 4 0.6 4472 10 8 2 0.8 4473 10 5 5 0.5 4474 10 6 4 0.6 4475 10 4 6 0.4 4476 10 6 4 0.6 4477 10 6 4 0.6 4478 10 3 7 0.3 4479 10 6 4 0.6 4480 10 3 7 0.3 4481 10 3 7 0.3 4482 10 7 3 0.7 4483 10 7 3 0.7 4484 10 4 6 0.4 4485 10 7 3 0.7 4486 10 6 4 0.6 4487 10 6 4 0.6 4488 10 2 8 0.2 4489 10 5 5 0.5 4490 10 5 5 0.5 4491 10 8 2 0.8 4492 10 7 3 0.7 4493 10 7 3 0.7 4494 10 4 6 0.4 4495 10 6 4 0.6 4496 10 3 7 0.3 4497 10 8 2 0.8 4498 10 8 2 0.8 4499 10 6 4 0.6 4500 10 4 6 0.4 4501 10 4 6 0.4 4502 10 8 2 0.8 4503 10 4 6 0.4 4504 10 7 3 0.7 4505 10 6 4 0.6 4506 10 6 4 0.6 4507 10 7 3 0.7 4508 10 4 6 0.4 4509 10 4 6 0.4 4510 10 5 5 0.5 4511 10 5 5 0.5 4512 10 3 7 0.3 4513 10 6 4 0.6 4514 10 3 7 0.3 4515 10 7 3 0.7 4516 10 5 5 0.5 4517 10 6 4 0.6 4518 10 6 4 0.6 4519 10 7 3 0.7 4520 10 3 7 0.3 4521 10 3 7 0.3 4522 10 6 4 0.6 4523 10 6 4 0.6 4524 10 3 7 0.3 4525 10 6 4 0.6 4526 10 5 5 0.5 4527 10 6 4 0.6 4528 10 3 7 0.3 4529 10 4 6 0.4 4530 10 8 2 0.8 4531 10 8 2 0.8 4532 10 7 3 0.7 4533 10 7 3 0.7 4534 10 5 5 0.5 4535 10 4 6 0.4 4536 10 4 6 0.4 4537 10 7 3 0.7 4538 10 5 5 0.5 4539 10 5 5 0.5 4540 10 6 4 0.6 4541 10 4 6 0.4 4542 10 6 4 0.6 4543 10 6 4 0.6 4544 10 6 4 0.6 4545 10 7 3 0.7 4546 10 5 5 0.5 4547 10 6 4 0.6 4548 10 4 6 0.4 4549 10 5 5 0.5 4550 10 6 4 0.6 4551 10 5 5 0.5 4552 10 7 3 0.7 4553 10 6 4 0.6 4554 10 6 4 0.6 4555 10 7 3 0.7 4556 10 4 6 0.4 4557 10 6 4 0.6 4558 10 4 6 0.4 4559 10 6 4 0.6 4560 10 9 1 0.9 4561 10 6 4 0.6 4562 10 6 4 0.6 4563 10 2 8 0.2 4564 10 5 5 0.5 4565 10 4 6 0.4 4566 10 6 4 0.6 4567 10 4 6 0.4 4568 10 3 7 0.3 4569 10 8 2 0.8 4570 10 6 4 0.6 4571 10 4 6 0.4 4572 10 4 6 0.4 4573 10 6 4 0.6 4574 10 2 8 0.2 4575 10 4 6 0.4 4576 10 2 8 0.2 4577 10 7 3 0.7 4578 10 3 7 0.3 4579 10 7 3 0.7 4580 10 5 5 0.5 4581 10 2 8 0.2 4582 10 4 6 0.4 4583 10 3 7 0.3 4584 10 6 4 0.6 4585 10 7 3 0.7 4586 10 6 4 0.6 4587 10 8 2 0.8 4588 10 5 5 0.5 4589 10 5 5 0.5 4590 10 5 5 0.5 4591 10 6 4 0.6 4592 10 3 7 0.3 4593 10 6 4 0.6 4594 10 5 5 0.5 4595 10 6 4 0.6 4596 10 6 4 0.6 4597 10 4 6 0.4 4598 10 4 6 0.4 4599 10 7 3 0.7 4600 10 7 3 0.7 4601 10 8 2 0.8 4602 10 7 3 0.7 4603 10 6 4 0.6 4604 10 7 3 0.7 4605 10 7 3 0.7 4606 10 4 6 0.4 4607 10 7 3 0.7 4608 10 6 4 0.6 4609 10 6 4 0.6 4610 10 5 5 0.5 4611 10 5 5 0.5 4612 10 5 5 0.5 4613 10 7 3 0.7 4614 10 5 5 0.5 4615 10 6 4 0.6 4616 10 6 4 0.6 4617 10 5 5 0.5 4618 10 6 4 0.6 4619 10 6 4 0.6 4620 10 7 3 0.7 4621 10 5 5 0.5 4622 10 5 5 0.5 4623 10 2 8 0.2 4624 10 5 5 0.5 4625 10 7 3 0.7 4626 10 7 3 0.7 4627 10 5 5 0.5 4628 10 5 5 0.5 4629 10 5 5 0.5 4630 10 6 4 0.6 4631 10 5 5 0.5 4632 10 5 5 0.5 4633 10 5 5 0.5 4634 10 8 2 0.8 4635 10 6 4 0.6 4636 10 6 4 0.6 4637 10 6 4 0.6 4638 10 6 4 0.6 4639 10 7 3 0.7 4640 10 3 7 0.3 4641 10 5 5 0.5 4642 10 5 5 0.5 4643 10 5 5 0.5 4644 10 4 6 0.4 4645 10 4 6 0.4 4646 10 8 2 0.8 4647 10 5 5 0.5 4648 10 7 3 0.7 4649 10 7 3 0.7 4650 10 5 5 0.5 4651 10 6 4 0.6 4652 10 6 4 0.6 4653 10 5 5 0.5 4654 10 6 4 0.6 4655 10 4 6 0.4 4656 10 7 3 0.7 4657 10 6 4 0.6 4658 10 5 5 0.5 4659 10 7 3 0.7 4660 10 7 3 0.7 4661 10 1 9 0.1 4662 10 6 4 0.6 4663 10 6 4 0.6 4664 10 6 4 0.6 4665 10 5 5 0.5 4666 10 4 6 0.4 4667 10 7 3 0.7 4668 10 7 3 0.7 4669 10 6 4 0.6 4670 10 7 3 0.7 4671 10 5 5 0.5 4672 10 7 3 0.7 4673 10 5 5 0.5 4674 10 4 6 0.4 4675 10 3 7 0.3 4676 10 4 6 0.4 4677 10 5 5 0.5 4678 10 4 6 0.4 4679 10 6 4 0.6 4680 10 4 6 0.4 4681 10 6 4 0.6 4682 10 6 4 0.6 4683 10 6 4 0.6 4684 10 6 4 0.6 4685 10 5 5 0.5 4686 10 7 3 0.7 4687 10 4 6 0.4 4688 10 5 5 0.5 4689 10 6 4 0.6 4690 10 6 4 0.6 4691 10 4 6 0.4 4692 10 7 3 0.7 4693 10 2 8 0.2 4694 10 6 4 0.6 4695 10 2 8 0.2 4696 10 6 4 0.6 4697 10 3 7 0.3 4698 10 4 6 0.4 4699 10 6 4 0.6 4700 10 5 5 0.5 4701 10 6 4 0.6 4702 10 2 8 0.2 4703 10 6 4 0.6 4704 10 7 3 0.7 4705 10 5 5 0.5 4706 10 5 5 0.5 4707 10 6 4 0.6 4708 10 5 5 0.5 4709 10 7 3 0.7 4710 10 4 6 0.4 4711 10 4 6 0.4 4712 10 5 5 0.5 4713 10 5 5 0.5 4714 10 5 5 0.5 4715 10 4 6 0.4 4716 10 7 3 0.7 4717 10 2 8 0.2 4718 10 6 4 0.6 4719 10 5 5 0.5 4720 10 5 5 0.5 4721 10 6 4 0.6 4722 10 5 5 0.5 4723 10 7 3 0.7 4724 10 4 6 0.4 4725 10 8 2 0.8 4726 10 4 6 0.4 4727 10 4 6 0.4 4728 10 4 6 0.4 4729 10 5 5 0.5 4730 10 5 5 0.5 4731 10 6 4 0.6 4732 10 3 7 0.3 4733 10 5 5 0.5 4734 10 6 4 0.6 4735 10 3 7 0.3 4736 10 5 5 0.5 4737 10 4 6 0.4 4738 10 2 8 0.2 4739 10 4 6 0.4 4740 10 7 3 0.7 4741 10 7 3 0.7 4742 10 4 6 0.4 4743 10 4 6 0.4 4744 10 3 7 0.3 4745 10 9 1 0.9 4746 10 4 6 0.4 4747 10 4 6 0.4 4748 10 6 4 0.6 4749 10 6 4 0.6 4750 10 3 7 0.3 4751 10 6 4 0.6 4752 10 6 4 0.6 4753 10 2 8 0.2 4754 10 2 8 0.2 4755 10 5 5 0.5 4756 10 4 6 0.4 4757 10 7 3 0.7 4758 10 7 3 0.7 4759 10 4 6 0.4 4760 10 5 5 0.5 4761 10 4 6 0.4 4762 10 8 2 0.8 4763 10 4 6 0.4 4764 10 7 3 0.7 4765 10 4 6 0.4 4766 10 5 5 0.5 4767 10 6 4 0.6 4768 10 6 4 0.6 4769 10 4 6 0.4 4770 10 4 6 0.4 4771 10 2 8 0.2 4772 10 5 5 0.5 4773 10 5 5 0.5 4774 10 4 6 0.4 4775 10 5 5 0.5 4776 10 6 4 0.6 4777 10 4 6 0.4 4778 10 6 4 0.6 4779 10 5 5 0.5 4780 10 6 4 0.6 4781 10 1 9 0.1 4782 10 7 3 0.7 4783 10 6 4 0.6 4784 10 6 4 0.6 4785 10 5 5 0.5 4786 10 7 3 0.7 4787 10 4 6 0.4 4788 10 4 6 0.4 4789 10 4 6 0.4 4790 10 4 6 0.4 4791 10 5 5 0.5 4792 10 3 7 0.3 4793 10 5 5 0.5 4794 10 6 4 0.6 4795 10 6 4 0.6 4796 10 6 4 0.6 4797 10 6 4 0.6 4798 10 3 7 0.3 4799 10 8 2 0.8 4800 10 4 6 0.4 4801 10 4 6 0.4 4802 10 3 7 0.3 4803 10 4 6 0.4 4804 10 8 2 0.8 4805 10 6 4 0.6 4806 10 8 2 0.8 4807 10 1 9 0.1 4808 10 7 3 0.7 4809 10 6 4 0.6 4810 10 6 4 0.6 4811 10 5 5 0.5 4812 10 6 4 0.6 4813 10 5 5 0.5 4814 10 5 5 0.5 4815 10 7 3 0.7 4816 10 5 5 0.5 4817 10 6 4 0.6 4818 10 5 5 0.5 4819 10 4 6 0.4 4820 10 2 8 0.2 4821 10 4 6 0.4 4822 10 4 6 0.4 4823 10 5 5 0.5 4824 10 6 4 0.6 4825 10 3 7 0.3 4826 10 4 6 0.4 4827 10 3 7 0.3 4828 10 2 8 0.2 4829 10 7 3 0.7 4830 10 7 3 0.7 4831 10 7 3 0.7 4832 10 6 4 0.6 4833 10 2 8 0.2 4834 10 5 5 0.5 4835 10 5 5 0.5 4836 10 6 4 0.6 4837 10 3 7 0.3 4838 10 6 4 0.6 4839 10 4 6 0.4 4840 10 6 4 0.6 4841 10 4 6 0.4 4842 10 3 7 0.3 4843 10 5 5 0.5 4844 10 8 2 0.8 4845 10 6 4 0.6 4846 10 5 5 0.5 4847 10 5 5 0.5 4848 10 7 3 0.7 4849 10 1 9 0.1 4850 10 6 4 0.6 4851 10 4 6 0.4 4852 10 4 6 0.4 4853 10 6 4 0.6 4854 10 4 6 0.4 4855 10 4 6 0.4 4856 10 9 1 0.9 4857 10 5 5 0.5 4858 10 6 4 0.6 4859 10 3 7 0.3 4860 10 6 4 0.6 4861 10 6 4 0.6 4862 10 3 7 0.3 4863 10 5 5 0.5 4864 10 4 6 0.4 4865 10 4 6 0.4 4866 10 5 5 0.5 4867 10 5 5 0.5 4868 10 7 3 0.7 4869 10 5 5 0.5 4870 10 3 7 0.3 4871 10 4 6 0.4 4872 10 6 4 0.6 4873 10 7 3 0.7 4874 10 5 5 0.5 4875 10 4 6 0.4 4876 10 6 4 0.6 4877 10 5 5 0.5 4878 10 5 5 0.5 4879 10 3 7 0.3 4880 10 3 7 0.3 4881 10 4 6 0.4 4882 10 6 4 0.6 4883 10 7 3 0.7 4884 10 8 2 0.8 4885 10 3 7 0.3 4886 10 4 6 0.4 4887 10 4 6 0.4 4888 10 6 4 0.6 4889 10 6 4 0.6 4890 10 6 4 0.6 4891 10 4 6 0.4 4892 10 5 5 0.5 4893 10 6 4 0.6 4894 10 4 6 0.4 4895 10 4 6 0.4 4896 10 4 6 0.4 4897 10 6 4 0.6 4898 10 9 1 0.9 4899 10 5 5 0.5 4900 10 5 5 0.5 4901 10 4 6 0.4 4902 10 3 7 0.3 4903 10 2 8 0.2 4904 10 3 7 0.3 4905 10 5 5 0.5 4906 10 6 4 0.6 4907 10 7 3 0.7 4908 10 5 5 0.5 4909 10 4 6 0.4 4910 10 5 5 0.5 4911 10 5 5 0.5 4912 10 8 2 0.8 4913 10 4 6 0.4 4914 10 3 7 0.3 4915 10 5 5 0.5 4916 10 5 5 0.5 4917 10 9 1 0.9 4918 10 8 2 0.8 4919 10 7 3 0.7 4920 10 7 3 0.7 4921 10 5 5 0.5 4922 10 4 6 0.4 4923 10 5 5 0.5 4924 10 4 6 0.4 4925 10 8 2 0.8 4926 10 6 4 0.6 4927 10 3 7 0.3 4928 10 5 5 0.5 4929 10 3 7 0.3 4930 10 5 5 0.5 4931 10 4 6 0.4 4932 10 8 2 0.8 4933 10 5 5 0.5 4934 10 4 6 0.4 4935 10 4 6 0.4 4936 10 5 5 0.5 4937 10 2 8 0.2 4938 10 6 4 0.6 4939 10 8 2 0.8 4940 10 5 5 0.5 4941 10 5 5 0.5 4942 10 2 8 0.2 4943 10 6 4 0.6 4944 10 8 2 0.8 4945 10 5 5 0.5 4946 10 6 4 0.6 4947 10 3 7 0.3 4948 10 5 5 0.5 4949 10 1 9 0.1 4950 10 7 3 0.7 4951 10 4 6 0.4 4952 10 7 3 0.7 4953 10 6 4 0.6 4954 10 6 4 0.6 4955 10 5 5 0.5 4956 10 4 6 0.4 4957 10 4 6 0.4 4958 10 6 4 0.6 4959 10 6 4 0.6 4960 10 4 6 0.4 4961 10 7 3 0.7 4962 10 4 6 0.4 4963 10 2 8 0.2 4964 10 8 2 0.8 4965 10 7 3 0.7 4966 10 6 4 0.6 4967 10 6 4 0.6 4968 10 4 6 0.4 4969 10 7 3 0.7 4970 10 4 6 0.4 4971 10 5 5 0.5 4972 10 4 6 0.4 4973 10 7 3 0.7 4974 10 6 4 0.6 4975 10 6 4 0.6 4976 10 6 4 0.6 4977 10 3 7 0.3 4978 10 6 4 0.6 4979 10 8 2 0.8 4980 10 2 8 0.2 4981 10 6 4 0.6 4982 10 4 6 0.4 4983 10 3 7 0.3 4984 10 4 6 0.4 4985 10 7 3 0.7 4986 10 5 5 0.5 4987 10 5 5 0.5 4988 10 4 6 0.4 4989 10 5 5 0.5 4990 10 6 4 0.6 4991 10 4 6 0.4 4992 10 2 8 0.2 4993 10 5 5 0.5 4994 10 4 6 0.4 4995 10 4 6 0.4 4996 10 7 3 0.7 4997 10 6 4 0.6 4998 10 5 5 0.5 4999 10 3 7 0.3 5000 10 4 6 0.4 ``` --- ## R Simulations Example ```r # Gah! There are too many rows! simulations <- do(5000) * rflip(10) ``` ```r # We could also View() it View(simulations) ``` --- ## R Simulations Example ```r # Plot our resulting proportions ggplot(data = simulations, aes(x = prop)) + geom_histogram(binwidth = 0.1, color = "white") ``` 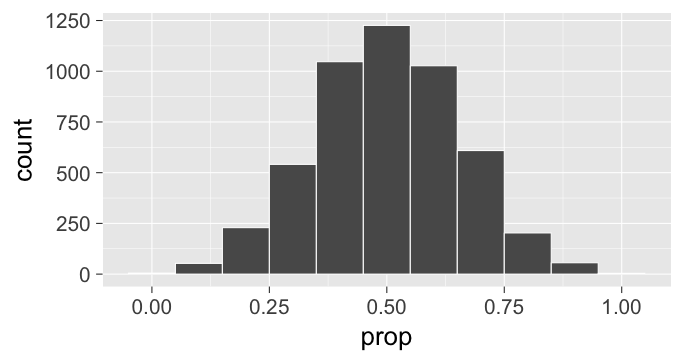<!-- --> --- ## R Simulations Example ```r # Plot our resulting proportions ggplot(data = simulations, mapping = aes(x = factor(prop))) + geom_bar() ``` 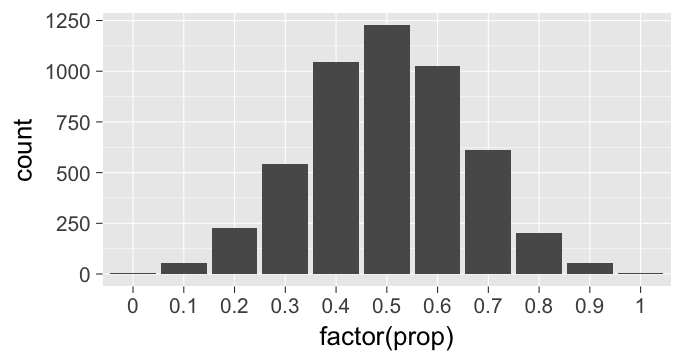<!-- --> --- ## Shuffling AKA Permuting Shuffling AKA re-ordering AKA **permuting** are all synonyms. I'm going to use all three terms interchangeably. Type the following code into your R script: ```r library(mosaic) # Define a vector fruit fruit <- c("apple", "orange", "mango") # Do this multiple times: shuffle(fruit) ``` --- ## Shuffling AKA Permuting This works with the `do()` operator... ```r do(5) * shuffle(fruit) ``` ... as well as within a `mutate()` ```r example_data <- data_frame( name = c("Ilana", "Abbi", "Hannibal"), fruit = c("apple", "orange", "mango") ) # Run this multiple times: example_data %>% mutate(fruit = shuffle(fruit)) ``` --- ## Key Distinction ### A *huge* distinction in types of sampling: 1. Sampling **with** replacement 1. Sampling **without** replacement --- ## Resampling ### At its most basic, `resample()` resamples the input vector **with** replacement. Run this in the console multiple times from your R script: ```r resample(fruit) ``` * You can get the same fruit all three times i.e. sampling with replacement * `resample()` has default settings that we can set to fit our needs; it is a swiss army knife. * Let's unpack the defaults: --- ## Resampling ```r resample(x = fruit, size = length(x), replace = TRUE, prob = rep(1 / length(x), times = length(x)) ) ``` * <small>`x`</small> is the input. In this case <small>`fruit`</small>. * <small>`size`</small>: size of output vector. By default the same size as <small>`x`</small>. * <small>`replace`</small>: Sample **with** or **without** replacement. By default with replacement. * <small>`prob`</small>: Probability of sampling each input value. By default, equal probability * Run the following. What does it do? ```r rep(1/length(fruit), times = length(fruit)) ``` --- ## Scenario for Today * Say you are a statistician and you meet someone called the "Lady Tasting Tea." * She claims she can tell by tasting whether the tea or the milk was added first to a cup. -- * You want to test whether + She can actually tell which came first **OR** + She's lying and is only guessing at random -- * Say you have just enough tea/milk to pour into 10 cups. --- ## Lady Tasting Tea * Lady Tasting Tea claims she can tell if tea or milk was poured first into a cup * You run an experiment with 10 cups if she can tell or if she is bullshitting * Let's _assume a hypothetical world where she is guessing at random_. --- ## Lady Tasting Tea ### If guessing at random, here are the *hypothetical* outcomes: 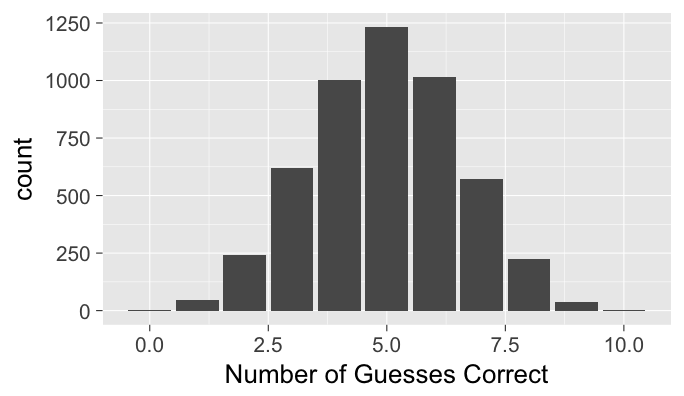<!-- --> --- ## Lady Tasting Tea She got 9/10 right! ```r ggplot(simulation, aes(x = heads)) + geom_bar() + labs(x = "Number of Guesses Correct") + geom_vline(xintercept = 9, color = "red") ``` <!-- --> --- ## Lady Tasting Tea She got 9/10 right! ```r ggplot(simulation, aes(x = heads)) + geom_bar() + labs(x = "Number of Guesses Correct") + geom_vline(xintercept = 9, color = "red") + scale_x_continuous(breaks = 0:10) ``` 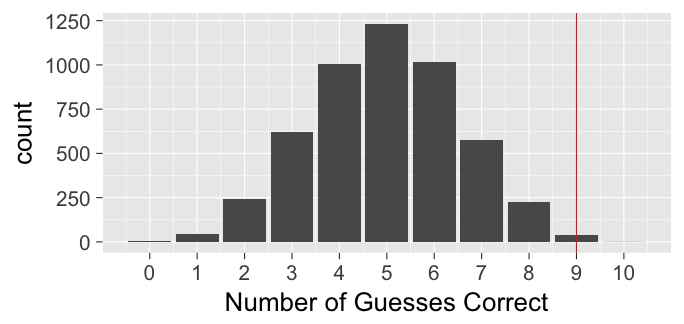<!-- --> --- ## Lady Tasting Tea * In our hypothetical world of guessing at random, 9/10 OR MORE occured 39 times out of 5000. i.e. <br> 0.78% of the time. * Can she tell, or is she bullshitting? --- ## Problem Set 10 - Due by end of class today - Turn in individually via pencil-and-paper ### [Learning Checks](https://ismayc.github.io/moderndiver-book/6-sim.html) - LC6.1 - LC6.3 - LC6.9 - LC6.11 - LC6.13 - LC6.15 --- layout: true .footer[[Week 10 - Tuesday](https://ismayc.github.io/soc301_s2017/2017/04/04/week-10---tuesday/)] --- name: week10t class: center, middle # Week 10 - Tuesday # April 4, 2017 --- # Warmup - Similar to Old Exam Question What `dplyr` and `ggplot2` code is needed to produce the following plot using the `gapminder` data set? (You can assume `library()` has already been run on all needed packages.) *Hint*: You should use the `%in%` operator. 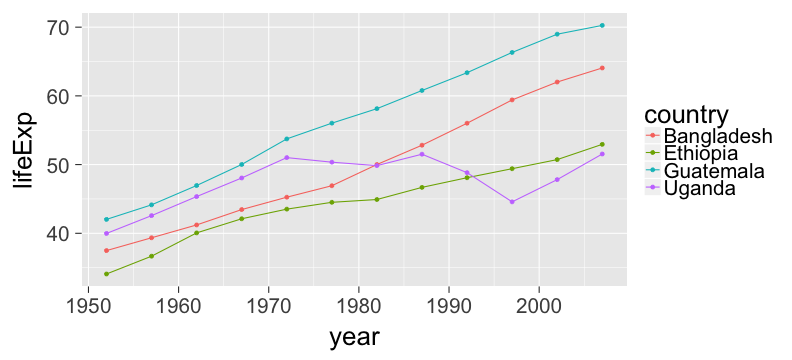<!-- --> What stands out to you from a social perspective as you observe this graph? --- ## Goals for the course going forward - Focus on understanding how data visualization and data wrangling play a role in inference - Discuss FiveThirtyEight articles from a social perspective while also relating back to statistical content - Work on summarizing important content and giving you a better sense of what to expect - What I need from you - More questions! I can't know what you don't understand unless you ask. - Focus on testing for your own understanding and speaking up when something needs more explanation --- ## Today: Switching Gears * We've covered **Tidy**, **Visualize**, and **Transform** * Start with **Model** * The focus throughout is on **Communicate**. <img src="figure/pipeline.png" alt="Drawing" style="width: 700px;" border="1"/> --- ## Key Definitions ### Let's ask our favorite search engine: * Define inference * Define **statistical** inference --- # Sampling ## Match the term to its correct definition - Carefully read over the [worksheet](sampling.pdf) individually - Complete the [worksheet](sampling.pdf) individually - Then pair up with two or three new people from class that you haven't worked with before to go over your answers --- ## The Paradigm for the Rest of the Class <img src="figure/target-population.jpg" alt="Drawing" style="width: 700px;" border="1"/> --- - <small><small>Identify the **population** of interest and the **population parameter**</small></small> - <small><small>Identify the **sample** used and the **statistic**</small></small> - <small><small>Comment on the **generalizability** of the results of the sample to the population.</small></small> *** - <small><small>You want to understand the income of Pacific University graduates in the last 10 years. You get the records of 10 randomly chosen Pacific graduates. You take the median of their answers.</small></small> - <small><small>Imagine it's 1993 i.e. almost all households have landlines. You want to know the average number of people in each household in Forest Grove. You randomly pick out 500 numbers from the phone book & conduct a phone survey.</small></small> - <small><small>You want to know the prevalence (i.e. proportion) of illegal downloading of TV shows among Pacific University students. You get the emails of 100 randomly chosen Pacific University students and ask them "How many times did you download a pirated TV show last week?"</small></small> --- ## Visualizing Sampling ### OkCupid Self-reported Heights from [ModernDive](https://ismayc.github.io/moderndiver-book/6-sim.html#visualizing-sampling) - [Shiny app to better understand the role of sampling](https://ismay.shinyapps.io/okcupidheights/) --- ## Recall * **Random** sampling: where we select the sample from the population in an unbiased fashion * Bias: A sample is biased if some observations have a higher **probability** of being included in the sample. > * Keywords: **random** and **probability** -- * In short: Probability is the study of randomness. * Its roots lie in one [historical constant](https://www.google.com/search?q=probability&newwindow=1&source=lnms&tbm=isch&sa=X&ved=0ahUKEwjnvJG7_oTQAhVIQiYKHSQ7CnoQ_AUICSgC&biw=2559&bih=1316&dpr=2#newwindow=1&tbm=isch&q=history+of+gambling). --- ## Two Approaches to Probability There are two approaches to studying probability: Mathematically | Simulations :-------------------------:|:-------------------------: <img src="figure/pdf.png" alt="Drawing" style="width: 300px;"/> | <img src="figure/coin.jpg" alt="Drawing" style="width: 300px;"/> > * **Note**: The "random simulation" in question is not limited to coin flips. --- ## Two Approaches to Probability - The mathematical approach to studying probability requires more mathematical background: set theory, discrete math, calculus, and later on measure theory. -- - The simulation approach does not. -- - Instead we require a computer's **random number generator** to generate simulations. Why? --- ## Simulations via Computer Doing this repeatedly by hand is tiring: <img src="figure/coin.jpg" alt="Drawing" style="width: 100px;"/><img src="figure/coin.jpg" alt="Drawing" style="width: 100px;"/><img src="figure/coin.jpg" alt="Drawing" style="width: 100px;"/><img src="figure/coin.jpg" alt="Drawing" style="width: 100px;"/><img src="figure/coin.jpg" alt="Drawing" style="width: 100px;"/><img src="figure/coin.jpg" alt="Drawing" style="width: 100px;"/><img src="figure/coin.jpg" alt="Drawing" style="width: 100px;"/><img src="figure/coin.jpg" alt="Drawing" style="width: 100px;"/><img src="figure/coin.jpg" alt="Drawing" style="width: 100px;"/><img src="figure/coin.jpg" alt="Drawing" style="width: 100px;"/><img src="figure/coin.jpg" alt="Drawing" style="width: 100px;"/><img src="figure/coin.jpg" alt="Drawing" style="width: 100px;"/><img src="figure/coin.jpg" alt="Drawing" style="width: 100px;"/><img src="figure/coin.jpg" alt="Drawing" style="width: 100px;"/><img src="figure/coin.jpg" alt="Drawing" style="width: 100px;"/><img src="figure/coin.jpg" alt="Drawing" style="width: 100px;"/><img src="figure/coin.jpg" alt="Drawing" style="width: 100px;"/><img src="figure/coin.jpg" alt="Drawing" style="width: 100px;"/><img src="figure/coin.jpg" alt="Drawing" style="width: 100px;"/><img src="figure/coin.jpg" alt="Drawing" style="width: 100px;"/><img src="figure/coin.jpg" alt="Drawing" style="width: 100px;"/><img src="figure/coin.jpg" alt="Drawing" style="width: 100px;"/><img src="figure/coin.jpg" alt="Drawing" style="width: 100px;"/><img src="figure/coin.jpg" alt="Drawing" style="width: 100px;"/><img src="figure/coin.jpg" alt="Drawing" style="width: 100px;"/><img src="figure/coin.jpg" alt="Drawing" style="width: 100px;"/><img src="figure/coin.jpg" alt="Drawing" style="width: 100px;"/><img src="figure/coin.jpg" alt="Drawing" style="width: 100px;"/><img src="figure/coin.jpg" alt="Drawing" style="width: 100px;"/><img src="figure/coin.jpg" alt="Drawing" style="width: 100px;"/><img src="figure/coin.jpg" alt="Drawing" style="width: 100px;"/><img src="figure/coin.jpg" alt="Drawing" style="width: 100px;"/><img src="figure/coin.jpg" alt="Drawing" style="width: 100px;"/><img src="figure/coin.jpg" alt="Drawing" style="width: 100px;"/><img src="figure/coin.jpg" alt="Drawing" style="width: 100px;"/><img src="figure/coin.jpg" alt="Drawing" style="width: 100px;"/><img src="figure/coin.jpg" alt="Drawing" style="width: 100px;"/><img src="figure/coin.jpg" alt="Drawing" style="width: 100px;"/><img src="figure/coin.jpg" alt="Drawing" style="width: 100px;"/><img src="figure/coin.jpg" alt="Drawing" style="width: 100px;"/><img src="figure/coin.jpg" alt="Drawing" style="width: 100px;"/><img src="figure/coin.jpg" alt="Drawing" style="width: 100px;"/> --- # [Problem Set 9 Assignment for Thursday](http://ismayc.github.io/soc301_s2017/problem-sets/index.html#ps9) - Due at the end of the day (11:59 PM on Thursday) - You will be peer reviewing a Group Project assigned to you - You'll also be including (Helpful for Midterm Exam) - an updated plot using `ggplot2` - a `dplyr` wrangling task to summarize the `fivethirtyeight` data frame used in the vignette - This will give you practive with `dplyr`, `ggplot2`, and `rmarkdown` while (hopefully) ensuring that you can perform the tasks asked of you in the group project individually. --- layout: true .footer[[Week 08 - Tuesday](https://ismayc.github.io/soc301_s2017/2017/03/21/week-08---tuesday/)] --- name: week08t class: center, middle # Week 08 - Tuesday # March 21, 2017 --- class: center, middle # Very impressed with Group Project drafts! <img src="https://media.giphy.com/media/sjDV6YTbw8tig/giphy.gif" width="80%" /> --- # Jazzing up your Group Project plots - Make sure to add labels to your axes and a title to your plot via `labs(x = , y = , title = )`. - Remember to use the `+` to add labs/themes to your plot just like you do with `geom_`etries --- # Jazzing up your Group Project plots - Think about using `theme_fivethirtyeight()` as I did in the `example_vignette.Rmd`. - Note your need to install the `ggthemes` package and load it via `library(ggthemes)` in the top R Markdown chunk. - Another option for cool themes of your plots is `theme_ipsum()` in the [`hrbrthemes` package](https://github.com/hrbrmstr/hrbrthemes) --- # Warmup -- 1. How can I better address this feedback? - "more of a social science aspect to the data we are using in our work." 2. "I was under the impression we were going to analyze data more than we have been." - What does 'analyzing data' mean to you? 3. Why do I ask DataCamp questions over content we haven't explicitly covered? --- # Comment ### "I wish there was a way for us to go over material with you if we had to miss class one day." -- - I'm happy to chat with you (even be it over video chat). All it takes is you reaching out to me. - I'd encourage you to look over the slides first to better understand what you've missed and to do the reading assignment in the textbook before reaching out to me. FoR Simulations Example, `geom_col` was discussed when we talked about [barplots](#geomcol). --- # Work day! - Look over my comments on your Group Project - Press the spellcheck button in R Markdown! <img src="figure/spellcheck.png" width="435" style="display: block; margin: auto;" /> - Do [Problem Set 8](https://goo.gl/forms/tCBFF7cuMbYgPIyb2) - Finish [dplyr Review](https://campus.datacamp.com/courses/effective-data-storytelling-using-the-tidyverse/dplyr-review-8) (Due today at 11:59 PM) - Read [Chapter 6 of ModernDive](https://ismayc.github.io/moderndiver-book/6-sim.html) ## ENJOY YOUR SPRING BREAK! --- layout: true .footer[[Week 07 - Tuesday](https://ismayc.github.io/soc301_s2017/2017/03/14/week-07---tuesday/)] --- name: week07t class: center, middle # Week 07 - Tuesday # March 14, 2017 --- # Warmup ### Write the code to produce the following table using ALL 5 of the 5MV functions in the `dplyr` package on the `gapminder` data frame in the `gapminder` R package. - Your code will be simplest if you use `|` in your `filter`. - Notice how the data is sorted. <br> <table> <thead> <tr> <th style="text-align:left;"> continent </th> <th style="text-align:left;"> country </th> <th style="text-align:right;"> mean_gdp_all_years </th> <th style="text-align:right;"> mean_minus_one </th> </tr> </thead> <tbody> <tr> <td style="text-align:left;"> Africa </td> <td style="text-align:left;"> South Africa </td> <td style="text-align:right;"> 7247.431 </td> <td style="text-align:right;"> 7246.431 </td> </tr> <tr> <td style="text-align:left;"> Oceania </td> <td style="text-align:left;"> New Zealand </td> <td style="text-align:right;"> 17262.623 </td> <td style="text-align:right;"> 17261.623 </td> </tr> <tr> <td style="text-align:left;"> Europe </td> <td style="text-align:left;"> Belgium </td> <td style="text-align:right;"> 19900.758 </td> <td style="text-align:right;"> 19899.758 </td> </tr> <tr> <td style="text-align:left;"> Oceania </td> <td style="text-align:left;"> Australia </td> <td style="text-align:right;"> 19980.596 </td> <td style="text-align:right;"> 19979.596 </td> </tr> </tbody> </table> --- class: center, middle # Today is a work day on your Group Projects --- layout: true .footer[[Week 06 - Thursday](https://ismayc.github.io/soc301_s2017/2017/03/09/week-07---thursday/)] --- name: week06th class: center, middle # Week 06 - Thursday # March 9, 2017 --- # Warmup ### Write the code to produce the following table using functions in the `dplyr` package on the `gapminder` data frame in the `gapminder` R package ``` Source: local data frame [4 x 3] Groups: continent [?] continent country mean_gdp_all_years <fctr> <fctr> <dbl> 1 Americas Canada 22410.75 2 Americas United States 26261.15 3 Oceania Australia 19980.60 4 Oceania New Zealand 17262.62 ``` --- # Setting 1 to change in RStudio <img src="figure/setting1.png" width="65%" style="display: block; margin: auto;" /> --- # Setting 2 to change in RStudio <img src="figure/setting2.png" width="65%" style="display: block; margin: auto;" /> --- # Review of the 5MV so far - `filter()` - `summarize()` - `group_by()` --- # The 5MV - `filter()` - `summarize()` - `group_by()` -- - `mutate()` -- - `arrange()` --- # `mutate()` - Allows you to 1. <font color="blue">create a new variable with a specific value</font> OR 2. create a new variable based on other variables OR 3. change the contents of an existing variable -- ```r gap_plus <- gapminder %>% mutate(just_one = 1) head(gap_plus) ``` ``` # A tibble: 6 × 7 country continent year lifeExp pop gdpPercap just_one <fctr> <fctr> <int> <dbl> <int> <dbl> <dbl> 1 Afghanistan Asia 1952 28.801 8425333 779.4453 1 2 Afghanistan Asia 1957 30.332 9240934 820.8530 1 3 Afghanistan Asia 1962 31.997 10267083 853.1007 1 4 Afghanistan Asia 1967 34.020 11537966 836.1971 1 5 Afghanistan Asia 1972 36.088 13079460 739.9811 1 6 Afghanistan Asia 1977 38.438 14880372 786.1134 1 ``` --- # `mutate()` - Allows you to 1. create a new variable with a specific value OR 2. <font color="blue">create a new variable based on other variables</font> OR 3. change the contents of an existing variable -- ```r gap_w_gdp <- gapminder %>% mutate(gdp = pop * gdpPercap) head(gap_w_gdp) ``` ``` # A tibble: 6 × 7 country continent year lifeExp pop gdpPercap gdp <fctr> <fctr> <int> <dbl> <int> <dbl> <dbl> 1 Afghanistan Asia 1952 28.801 8425333 779.4453 6567086330 2 Afghanistan Asia 1957 30.332 9240934 820.8530 7585448670 3 Afghanistan Asia 1962 31.997 10267083 853.1007 8758855797 4 Afghanistan Asia 1967 34.020 11537966 836.1971 9648014150 5 Afghanistan Asia 1972 36.088 13079460 739.9811 9678553274 6 Afghanistan Asia 1977 38.438 14880372 786.1134 11697659231 ``` --- # `mutate()` - Allows you to 1. create a new variable with a specific value OR 2. create a new variable based on other variables OR 3. <font color="blue">change the contents of an existing variable</font> -- ```r gap_weird <- gapminder %>% mutate(pop = pop + 1000) head(gap_weird) ``` ``` # A tibble: 6 × 6 country continent year lifeExp pop gdpPercap <fctr> <fctr> <int> <dbl> <dbl> <dbl> 1 Afghanistan Asia 1952 28.801 8426333 779.4453 2 Afghanistan Asia 1957 30.332 9241934 820.8530 3 Afghanistan Asia 1962 31.997 10268083 853.1007 4 Afghanistan Asia 1967 34.020 11538966 836.1971 5 Afghanistan Asia 1972 36.088 13080460 739.9811 6 Afghanistan Asia 1977 38.438 14881372 786.1134 ``` --- # `arrange()` - Reorders the rows in a data frame based on the values of one or more variables -- ```r gapminder %>% arrange(year, country) ``` ``` # A tibble: 1,704 × 6 country continent year lifeExp pop gdpPercap <fctr> <fctr> <int> <dbl> <int> <dbl> 1 Afghanistan Asia 1952 28.801 8425333 779.4453 2 Albania Europe 1952 55.230 1282697 1601.0561 3 Algeria Africa 1952 43.077 9279525 2449.0082 4 Angola Africa 1952 30.015 4232095 3520.6103 5 Argentina Americas 1952 62.485 17876956 5911.3151 6 Australia Oceania 1952 69.120 8691212 10039.5956 7 Austria Europe 1952 66.800 6927772 6137.0765 8 Bahrain Asia 1952 50.939 120447 9867.0848 9 Bangladesh Asia 1952 37.484 46886859 684.2442 10 Belgium Europe 1952 68.000 8730405 8343.1051 # ... with 1,694 more rows ``` --- # `arrange()` - Can also put into descending order -- ```r gapminder %>% filter(year > 2000) %>% arrange(desc(lifeExp)) ``` -- ``` # A tibble: 284 × 6 country continent year lifeExp pop gdpPercap <fctr> <fctr> <int> <dbl> <int> <dbl> 1 Japan Asia 2007 82.603 127467972 31656.07 2 Hong Kong, China Asia 2007 82.208 6980412 39724.98 3 Japan Asia 2002 82.000 127065841 28604.59 4 Iceland Europe 2007 81.757 301931 36180.79 5 Switzerland Europe 2007 81.701 7554661 37506.42 6 Hong Kong, China Asia 2002 81.495 6762476 30209.02 7 Australia Oceania 2007 81.235 20434176 34435.37 8 Spain Europe 2007 80.941 40448191 28821.06 9 Sweden Europe 2007 80.884 9031088 33859.75 10 Israel Asia 2007 80.745 6426679 25523.28 # ... with 274 more rows ``` --- # Other verbs ## `rename()` - To change a variable's name in a data frame to a new name - New name comes before the `=`, old name comes after the `=` -- ```r gap2 <- gapminder gap2 <- gap2 %>% rename(life_exp = lifeExp, gdp_per_cap = gdpPercap) head(gap2, 3) ``` ``` # A tibble: 3 × 6 country continent year life_exp pop gdp_per_cap <fctr> <fctr> <int> <dbl> <int> <dbl> 1 Afghanistan Asia 1952 28.801 8425333 779.4453 2 Afghanistan Asia 1957 30.332 9240934 820.8530 3 Afghanistan Asia 1962 31.997 10267083 853.1007 ``` --- # More with `inner_join()` - Join `by` columns of different names ```r library(readr) library(dplyr) url <- "http://ismayc.github.io/soc301_s2017/countries.csv" countries <- read_csv(url) head(countries) ``` ``` # A tibble: 6 × 3 name region sub_region <chr> <chr> <chr> 1 Afghanistan Asia Southern Asia 2 Åland Islands Europe Northern Europe 3 Albania Europe Southern Europe 4 Algeria Africa Northern Africa 5 American Samoa Oceania Polynesia 6 Andorra Europe Southern Europe ``` --- # More with `inner_join()` ```r new_gap <- inner_join(x = gapminder, y = countries, by = c("country" = "name")) %>% arrange(year) %>% select(-pop, -gdpPercap) head(new_gap, 10) ``` ``` # A tibble: 10 × 6 country continent year lifeExp region sub_region <chr> <fctr> <int> <dbl> <chr> <chr> 1 Afghanistan Asia 1952 28.801 Asia Southern Asia 2 Albania Europe 1952 55.230 Europe Southern Europe 3 Algeria Africa 1952 43.077 Africa Northern Africa 4 Angola Africa 1952 30.015 Africa Middle Africa 5 Argentina Americas 1952 62.485 Americas South America 6 Australia Oceania 1952 69.120 Oceania Australia and New Zealand 7 Austria Europe 1952 66.800 Europe Western Europe 8 Bahrain Asia 1952 50.939 Asia Western Asia 9 Bangladesh Asia 1952 37.484 Asia Southern Asia 10 Belgium Europe 1952 68.000 Europe Western Europe ``` --- # Work time! *** ## Work on [PS6](../problem-sets/index.html#ps6) ## Ask questions about [DC8](https://campus.datacamp.com/courses/reporting-with-r-markdown) ## Work on [DC9](https://campus.datacamp.com/courses/effective-data-storytelling-using-the-tidyverse/filtering-grouping-summarizing) ## Work on Group Project --- layout: true .footer[[Week 06 - Tuesday](https://ismayc.github.io/soc301_s2017/2017/03/07/week-06---tuesday/)] --- name: week06t class: center, middle # Week 06 - Tuesday # March 7, 2017 *** ### Make sure to share your Group Project proposals with me --- # Follow-up on assignment for today ## Check that R and RStudio installed on your machine ## And all that needed packages are installed ```r needed_pkgs <- c("nycflights13", "tibble", "dplyr", "ggplot2", "knitr", "okcupiddata", "dygraphs", "rmarkdown", "mosaic", "ggplot2movies", "fivethirtyeight") new.pkgs <- needed_pkgs[!(needed_pkgs %in% installed.packages())] if(length(new.pkgs)) { install.packages(new.pkgs, repos = "http://cran.rstudio.com") } ``` --- # Group Options - If you want to work in a group of four, you can do so BUT you'll need to complete 2 unrelated vignettes. -- - If you are left in a group of two, I will work with you to set up an assignment that is fair. -- - Final decisions on group members MUST be made by the end of the day today. I won't accept any changes in group members after today. -- - If you have problems contacting your group members, please let me know on Slack as soon as possible. **Check** SLACK at least once per day! --- # Group Options - If you are already having trouble setting up times to meet to work on the Group Project, you are going to have an even harder time going forward. - Pick a standard time to meet that works for everyone. - I would count a missed group meeting as a failing grade for the project. -- - You will need to meet outside of class to complete this project. This could easily include having a Google Hangout video chat as needed. Further, if you set up tasks appropriately, you may not need to meet in person much. --- # Set-up Google Drive on your computer - Click on the link I sent you for your Group Project folder in your email or in the Slack message I sent to your group - https://www.google.com/drive/download/ -- - Open the Rproj file in the Google Drive folder on your computer -- - Make sure that `script.R` opens with RStudio -- - Try to Knit `group_project.Rmd` --- # Group Project Deadlines - Always check [the Group Project Outline](https://ismayc.github.io/soc301_s2017/group_project_outline/index.html) to make sure you are on schedule and more specific deadlines - March 14 (2:45 PM) - Group Project Draft Due - March 23 (10:00 AM) - Revised Group Project Due --- class: center, middle # Data ~~Manipulation~~ Wrangling --- ## The `gapminder` data frame in the `gapminder` package ```r library(gapminder) library(dplyr) glimpse(gapminder) ``` ``` Observations: 1,704 Variables: 6 $ country <fctr> Afghanistan, Afghanistan, Afghanistan, Afghanistan,... $ continent <fctr> Asia, Asia, Asia, Asia, Asia, Asia, Asia, Asia, Asi... $ year <int> 1952, 1957, 1962, 1967, 1972, 1977, 1982, 1987, 1992... $ lifeExp <dbl> 28.801, 30.332, 31.997, 34.020, 36.088, 38.438, 39.8... $ pop <int> 8425333, 9240934, 10267083, 11537966, 13079460, 1488... $ gdpPercap <dbl> 779.4453, 820.8530, 853.1007, 836.1971, 739.9811, 78... ``` --- ## Base R versus the `tidyverse` - Say we wanted mean life expectancy across all years for Asia -- ```r # Base R asia <- gapminder[gapminder$continent == "Asia", ] mean(asia$lifeExp) ``` ``` [1] 60.0649 ``` -- ```r library(dplyr) gapminder %>% filter(continent == "Asia") %>% summarize(mean_exp = mean(lifeExp)) ``` ``` # A tibble: 1 × 1 mean_exp <dbl> 1 60.0649 ``` --- ## The pipe `%>%` <img src="figure/pipe.png" width="245" />       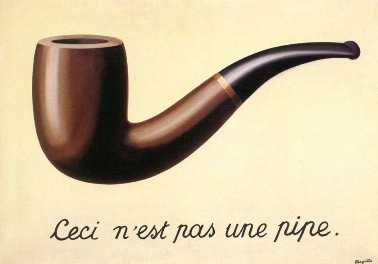<!-- --> -- - A way to chain together commands -- - It is *essentially* the `dplyr` equivalent to the <br> `+` in `ggplot2` --- # The 5NG of data viz -- ### `geom_point()`<br> `geom_line()` <br> `geom_histogram()`<br> `geom_boxplot()`<br> `geom_bar()` --- # The 5MV of data wrangling ### `filter()` <br> `summarize()` <br> `group_by()` <br> `mutate()` <br> `arrange()` --- # `filter()` - Allows you to select a subset of the rows of a data frame. - The arguments are the "filters" that you'd like to apply. -- ```r library(gapminder); library(dplyr) gap_2007 <- gapminder %>% filter(year == 2007) head(gap_2007) ``` ``` # A tibble: 6 × 6 country continent year lifeExp pop gdpPercap <fctr> <fctr> <int> <dbl> <int> <dbl> 1 Afghanistan Asia 2007 43.828 31889923 974.5803 2 Albania Europe 2007 76.423 3600523 5937.0295 3 Algeria Africa 2007 72.301 33333216 6223.3675 4 Angola Africa 2007 42.731 12420476 4797.2313 5 Argentina Americas 2007 75.320 40301927 12779.3796 6 Australia Oceania 2007 81.235 20434176 34435.3674 ``` --- # Logical operators - Use `==` to compare a variable to a value -- ```r library(gapminder); library(dplyr) gap_2007 <- gapminder %>% filter(year = 2007) ``` ``` Error: filter() takes unnamed arguments. Do you need `==`? ``` --- # Logical operators - Use `|` to check for any in multiple filters being true: -- ```r gapminder %>% filter(year == 2002 | continent == "Europe") ``` -- ``` # A tibble: 472 × 6 country continent year lifeExp pop gdpPercap <fctr> <fctr> <int> <dbl> <int> <dbl> 1 Afghanistan Asia 2002 42.129 25268405 726.7341 2 Albania Europe 1952 55.230 1282697 1601.0561 3 Albania Europe 1957 59.280 1476505 1942.2842 4 Albania Europe 1962 64.820 1728137 2312.8890 5 Albania Europe 1967 66.220 1984060 2760.1969 6 Albania Europe 1972 67.690 2263554 3313.4222 7 Albania Europe 1977 68.930 2509048 3533.0039 8 Albania Europe 1982 70.420 2780097 3630.8807 9 Albania Europe 1987 72.000 3075321 3738.9327 10 Albania Europe 1992 71.581 3326498 2497.4379 # ... with 462 more rows ``` --- # Logical operators - Use `&` or `,` to check for all of multiple filters being true: -- ```r gapminder %>% filter(year == 2002, continent == "Europe") ``` ``` # A tibble: 30 × 6 country continent year lifeExp pop gdpPercap <fctr> <fctr> <int> <dbl> <int> <dbl> 1 Albania Europe 2002 75.651 3508512 4604.212 2 Austria Europe 2002 78.980 8148312 32417.608 3 Belgium Europe 2002 78.320 10311970 30485.884 4 Bosnia and Herzegovina Europe 2002 74.090 4165416 6018.975 5 Bulgaria Europe 2002 72.140 7661799 7696.778 6 Croatia Europe 2002 74.876 4481020 11628.389 7 Czech Republic Europe 2002 75.510 10256295 17596.210 8 Denmark Europe 2002 77.180 5374693 32166.500 9 Finland Europe 2002 78.370 5193039 28204.591 10 France Europe 2002 79.590 59925035 28926.032 # ... with 20 more rows ``` --- # Logical operators - Use `%in%` to check for any being true <br> (shortcut to using `|` repeatedly) -- ```r gapminder %>% filter(country %in% c("Argentina", "Belgium", "Mexico"), year %in% c(1987, 1992)) ``` -- ``` # A tibble: 6 × 6 country continent year lifeExp pop gdpPercap <fctr> <fctr> <int> <dbl> <int> <dbl> 1 Argentina Americas 1987 70.774 31620918 9139.671 2 Argentina Americas 1992 71.868 33958947 9308.419 3 Belgium Europe 1987 75.350 9870200 22525.563 4 Belgium Europe 1992 76.460 10045622 25575.571 5 Mexico Americas 1987 69.498 80122492 8688.156 6 Mexico Americas 1992 71.455 88111030 9472.384 ``` --- # `summarize()` - Any numerical summary that you want to apply to a column of a data frame is specified within `summarize()`. ```r max_exp_1997 <- gapminder %>% filter(year == 1997) %>% summarize(max_exp = max(lifeExp)) max_exp_1997 ``` -- ``` # A tibble: 1 × 1 max_exp <dbl> 1 80.69 ``` --- ### Combining `summarize()` with `group_by()` - When you'd like to determine a numerical summary for all levels of a different categorical variable ```r max_exp_1997_by_cont <- gapminder %>% filter(year == 1997) %>% group_by(continent) %>% summarize(max_exp = max(lifeExp)) knitr::kable(max_exp_1997_by_cont, format = "html") ``` -- <table> <thead> <tr> <th style="text-align:left;"> continent </th> <th style="text-align:right;"> max_exp </th> </tr> </thead> <tbody> <tr> <td style="text-align:left;"> Africa </td> <td style="text-align:right;"> 74.772 </td> </tr> <tr> <td style="text-align:left;"> Americas </td> <td style="text-align:right;"> 78.610 </td> </tr> <tr> <td style="text-align:left;"> Asia </td> <td style="text-align:right;"> 80.690 </td> </tr> <tr> <td style="text-align:left;"> Europe </td> <td style="text-align:right;"> 79.390 </td> </tr> <tr> <td style="text-align:left;"> Oceania </td> <td style="text-align:right;"> 78.830 </td> </tr> </tbody> </table> --- class: center, middle # Finalize Group Project Proposal / Groups *** # Work on [PS6](../problem-sets/index.html#ps6) # Work on [DC8](https://campus.datacamp.com/courses/reporting-with-r-markdown) # Work on [DC9](https://campus.datacamp.com/courses/effective-data-storytelling-using-the-tidyverse/filtering-grouping-summarizing) --- layout: true .footer[[Week 05 - Thursday](https://ismayc.github.io/soc301_s2017/2017/03/02/week-05---thursday/)] --- name: week05th class: center, middle # Week 05 - Thursday # March 2, 2017 --- class: center, middle # The Process ## DataCamp *** -- ## RStudio -- ## `\(\rightarrow\)` R script + R Console -- ## `\(\rightarrow\)` R Markdown --- class: center, middle ## Analogy R | RStudio | DataCamp :-------------------------:|:-------------------------:|:-------------------------: <img src="figure/engine.jpg" alt="Drawing" style="width: 350px;"/> | <img src="figure/dashboard.jpg" alt="Drawing" style="width: 350px;"/> | <img src="figure/instructor.jpg" alt="Drawing" style="width: 350px;"/> --- class: center, middle # I'm ready to grant you your R driver's license -- ## But, you'll need to continue your tRaining to become an excellent driveR --- class: center, middle # R / RStudio / R Markdown DEMO --- # Diligently work on Group Project - Determine your group of three - Look over the `fivethirtyeight` data sets - [Reference manual](https://cran.r-project.org/web/packages/fivethirtyeight/fivethirtyeight.pdf) - [Listing of all data sets with links to 538 articles](https://cran.r-project.org/web/packages/fivethirtyeight/vignettes/fivethirtyeight.html) - Pick two that would be of interest to all group members - Review the [Group Project Outline](../group_project_outline/index.html) for further instructions --- class: center, middle # To Do for Next time ## Click the link <img src="https://image.freepik.com/free-icon/down-arrow-hand-drawn-sign_318-53286.jpg" width="50%" /> --- layout: true .footer[[Week 05 - Tuesday](https://ismayc.github.io/soc301_s2017/2017/02/28/week-05---tuesday/)] --- name: week05t class: center, middle # Week 05 - Tuesday # February 28, 2017 *** # Make sure to turn in PS4 --- # Warmup What code is needed to produce this plot using the `na.omit(flying)` data frame derived from the `fivethirtyeight` package? Make sure to load all needed libraries. <img src="slide_deck_files/figure-html/unnamed-chunk-96-1.png" style="display: block; margin: auto;" /> --- # `scale_fill_manual` - We will use `scale_`s sparingly but they can be nice for customizing your plots more - You'll probably want to use them on your Group Project -- - Let's review how it works on the `bechdel` plot on the **Showing Off** question you produced in your DataCamp homework. --- # Build the pieces ```r library(fivethirtyeight) library(ggplot2) ggplot(data = bechdel, mapping = aes(x = five_year)) + geom_bar(position = "fill") ``` 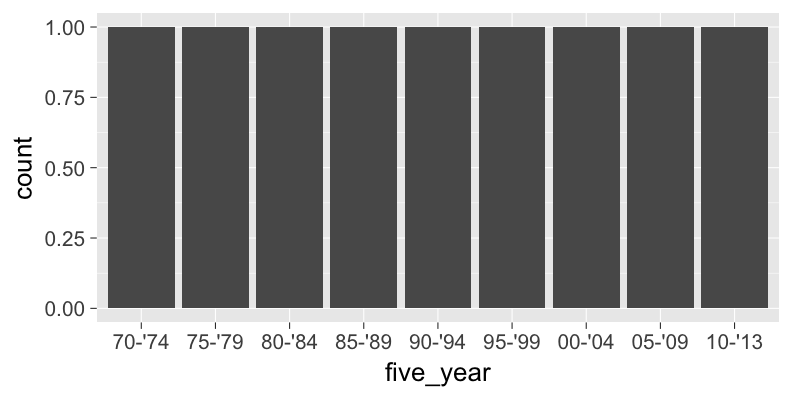<!-- --> --- # Build the pieces ```r ggplot(data = bechdel, mapping = aes(x = five_year, fill = clean_test)) + geom_bar(position = "fill") ``` 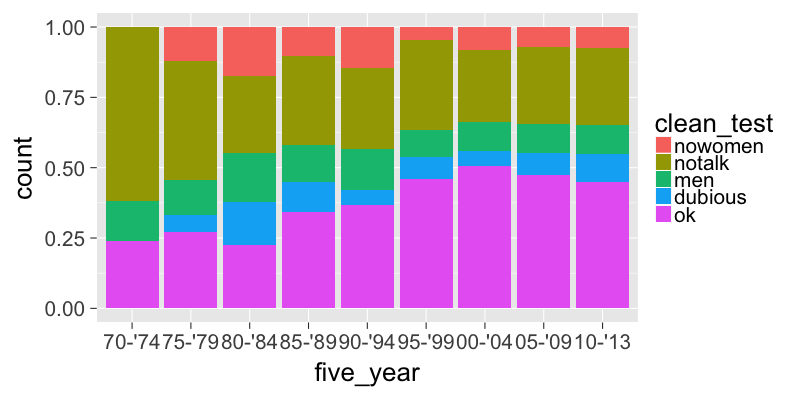<!-- --> --- # Build the pieces ```r ggplot(data = bechdel, mapping = aes(x = five_year, fill = clean_test)) + geom_bar(position = "fill", color = "white") ``` <!-- --> --- ```r ggplot(data = bechdel, mapping = aes(x = five_year, fill = clean_test)) + geom_bar(position = "fill", color = "white") + scale_fill_manual(values = c("#FF2603", "#FF937F", "#FEC9C3", "#6AB4DA", "#018ED5")) ``` 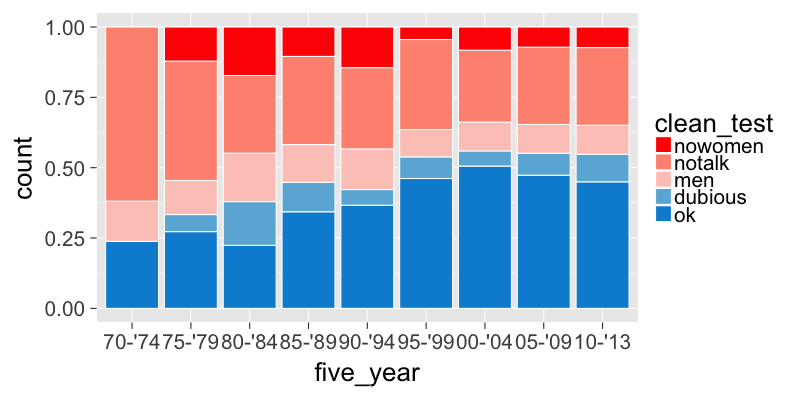<!-- --> Identified colors from the `bechdel` 538 [image](https://espnfivethirtyeight.files.wordpress.com/2014/04/hickey-bechdel-11.png?quality=90&strip=all&w=1150&ssl=1) at http://imagecolorpicker.com/ --- ### Could also... ```r ggplot(data = bechdel, mapping = aes(x = five_year, fill = clean_test)) + geom_bar(position = "fill", color = "black") + scale_fill_manual(values = c("navy", "blanchedalmond", "brown", "gray87", "lawngreen")) ``` 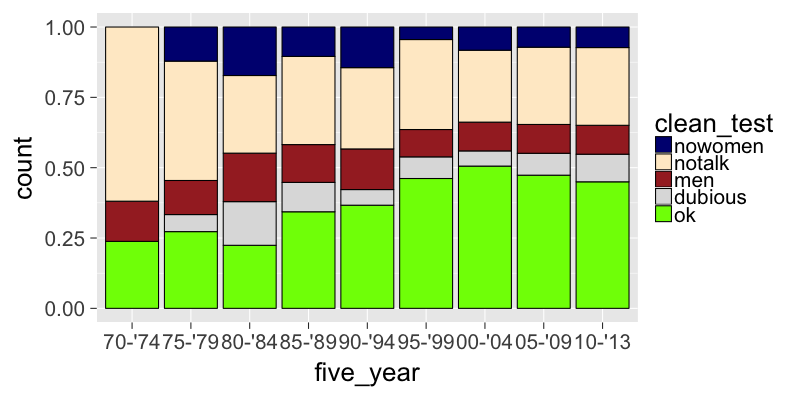<!-- --> --- class: center, middle # Review [PS4 Discussion](https://ismayc.github.io/soc301_s2017/problem-sets/ps-key/index.html#ps4-key) --- class: center, middle # Plicker time! ## Let's take [last semester's Quiz 2](https://ismayc.github.io/soc301_s2017/slides/Quiz2.pdf) --- class: center, middle # Discussion ## Why am I using DataCamp? -- ### How does this material apply to your everyday life? To your major? To the world as a whole? --- class: center, middle # Review Q & A - Get into groups and identify questions you have that you aren't sure about with the material from Chapters 3 and 4 - If you have no questions, take someone else's practice quiz --- class: center, middle # Check the post for the [day!](https://ismayc.github.io/soc301_s2017/2017/02/28/week-05---tuesday/) --- class: middle, center # Minute paper ### Write down as much information as you can about what you have learned about using `ggplot2` for graphics --- layout: true .footer[[Week 04 - Thursday](https://ismayc.github.io/soc301_s2017/2017/02/23/week-04---thursday/)] --- name: week04th class: center, middle # Week 04 - Thursday # February 23, 2017 --- # Warmup - Old Exam Question - Recall the `gap2007` data frame derived from the `gapminder` data frame in the `gapminder` package we worked with last week. (Assume that `gap2007` has been created and loaded for you.) - Write the code to produce an appropriate plot looking at the distribution of `gdpPercap` in `gap2007`. - Change the color of the inside of the plotted values and the border of the plotted values. --- class: center, middle # [DataCamp Demo](http://bit.ly/stats-sandbox) --- class: center, middle # Warmup in groups ### Discuss the difference between the `binwidth` and the `bins` arguments to `geom_histogram` --- class: split-50 ## The `library` function - Loads a package that contain functions and/or data ### At this point in the course you should only be adding these packages ### <u>For functions</u> `library(tibble)`<br> `library(ggplot2)`<br> `library(dplyr)` ### <u>For data</u> `library(nycflights13)`<br> `library(fivethirtyeight)`<br> `library(gapminder)` --- # Reviewing `ggplot2` syntax ```r library(________(1)_____________) ggplot(data = ______(2)___________, mapping = aes(x = ________(3)___________, y = ________(4)___________)) + geom_ _____(5)______() + facet_grid(facets = _______(6)___________) ``` -- ### Notes - Remember that faceting is setting up a formula so it needs a `~`. You'll get an error without it. - Note that the `+` must appear at the end of the line of code NOT at the beginning. --- ## Time slept vs day of the week for ONE professor over 100 weeks <img src="figure/box.jpg" width="900" height="350" alt="Sleep Boxplot"> - What does this plot tell you/what story does it tell? --- class: center, middle # [Reviewing Problem Set 3 Discussion](https://ismayc.github.io/soc301_s2017/problem-sets/ps-key/index.html#ps3-key) --- ## Types of variables & appropriate plots (so far) - One numerical variable -- - Histogram: `geom_histogram()` -- - Categorical explanatory & numerical response variable -- - Boxplot: `geom_boxplot()` - Faceted histogram: <br>`geom_histogram() + facet_grid( ~ _______)` -- - Numerical explanatory & response variables -- - Scatter-plot: `geom_point()` - Line-graph: `geom_line()` --- ## Recall creating a histogram from a frequency table Bin | Count --------|------- (9-15] | 4 (15-21] | 2 ```r library(ggplot2) ggplot(data = hist_example, mapping = aes(x = numeric_var)) + geom_histogram(binwidth = 6, color = "black", fill = "white") ``` 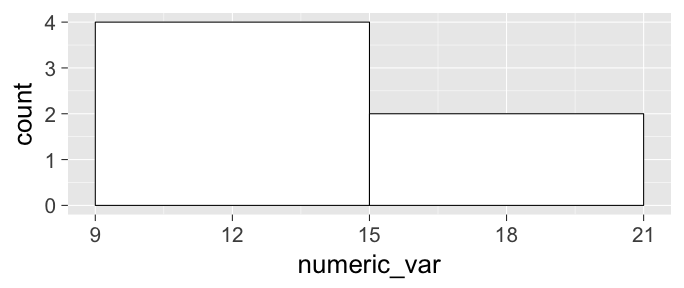<!-- --> --- - We are interested in the distribution of SOC 301 by grade. ```r class <- c(rep("Sophomore", times = 6), rep("Junior", times = 13), rep("Senior", times = 8)) class <- factor(class, levels = c("Sophomore", "Junior", "Senior")) class_df <- tibble::data_frame(class) class_df ``` ``` # A tibble: 27 × 1 class <fctr> 1 Sophomore 2 Sophomore 3 Sophomore 4 Sophomore 5 Sophomore 6 Sophomore 7 Junior 8 Junior 9 Junior 10 Junior # ... with 17 more rows ``` - Create a frequency table for this data on paper --- - Sketch a plot based on this frequency table class | Count --------|------- Sophomore | 6 Junior | 13 Senior | 8 -- ```r library(ggplot2) ggplot(data = class_df, mapping = aes(x = class)) + geom_bar(fill = "lightgreen", color = "black") ``` 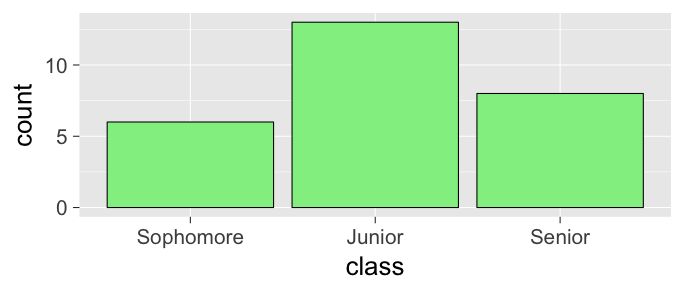<!-- --> --- # For aggregated data, use `geom_col` ```r class <- factor(c("Sophomore", "Junior", "Senior"), levels = c("Sophomore", "Junior", "Senior")) count <- c(6, 13, 8) class_freq <- tibble::data_frame(class, count) class_freq ``` ``` # A tibble: 3 × 2 class count <fctr> <dbl> 1 Sophomore 6 2 Junior 13 3 Senior 8 ``` --- name: geomcol # For aggregated data, use `geom_col` ```r ggplot(data = class_freq, mapping = aes(x = class, y = count)) + geom_col(fill = "lightgreen", color = "black") ``` 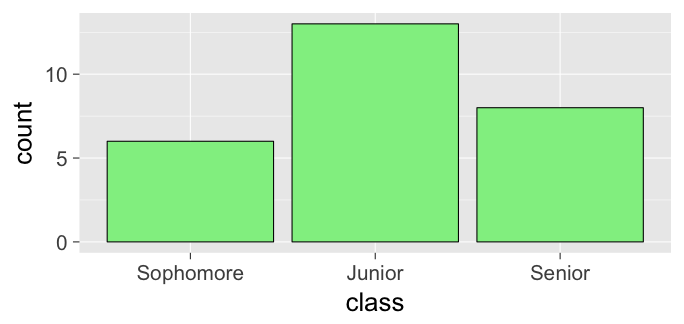<!-- --> --- # What about for two categorical variables? 1. Stacked barplot -- 2. Side-by-side barplot -- 3. Faceted barplot -- 4. Stacked proportional plot - Preferred if <br> number of groups in the second variable `\(\le 3\)` --- ## What code is needed to produce this plot? - This is using the `profiles` data frame in the `okcupiddata` package. 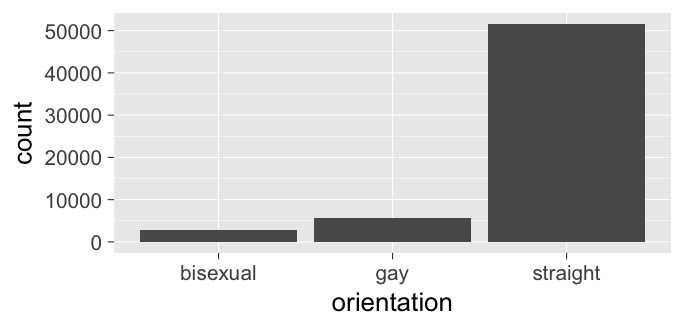<!-- --> --- # Stacked barplot - Uses `fill` to change the colors of the bars of the `x` variable based on the values of another variable -- - What code is needed to produce this plot? 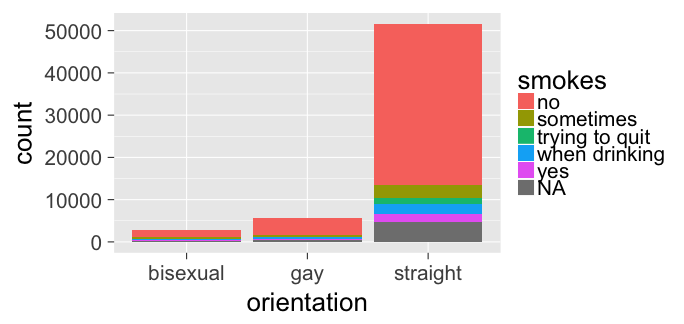<!-- --> --- # Side-by-side barplot - Uses `position = "dodge"` to place the bars next to each other for each group of the `x` variable -- - What code is needed to produce this plot? 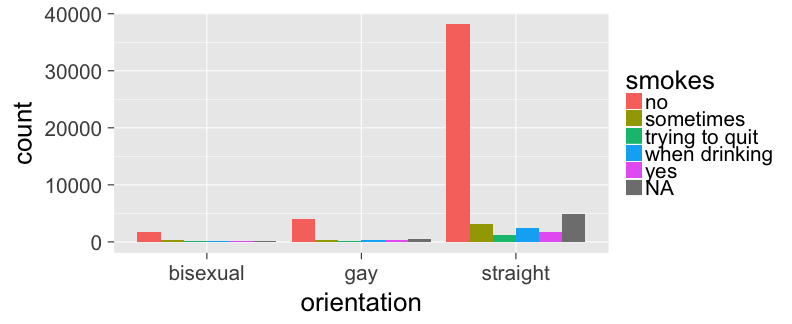<!-- --> --- # Faceted barplot - Uses `facet_wrap()` or `facet_grid()` to make small multiples of the plot -- - What code is needed to produce this plot? 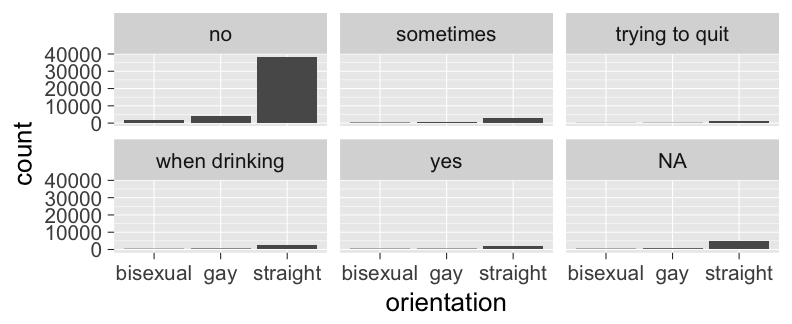<!-- --> --- # Stacked proportional barplot - Uses `position = "fill"` to show the percentage of the `fill` variable for each group of `x` -- - What code is needed to produce this plot? 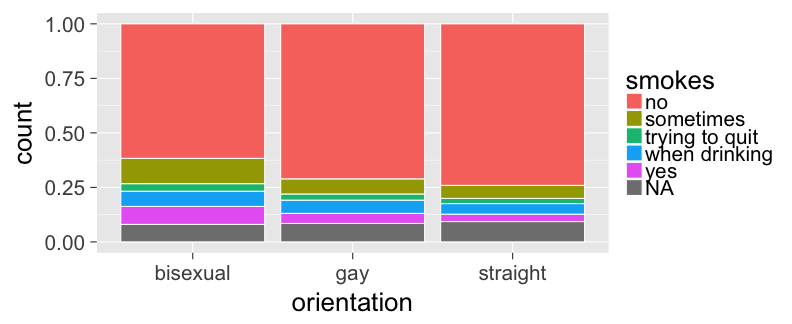<!-- --> --- class: center, middle # [Basic Data Visualization mindmap](https://coggle.it/diagram/V_G2gzukTDoQ-aZt-) --- class: center, middle # The [`ggplot2` Cheatsheet](https://www.rstudio.com/wp-content/uploads/2016/11/ggplot2-cheatsheet-2.1.pdf) --- ## Start looking over [`fivethirtyeight` data sets](https://cran.r-project.org/web/packages/fivethirtyeight/vignettes/fivethirtyeight.html) to find an interesting one for your Group Project - All the data sets are available in the [sandbox](http://bit.ly/stats-sandbox) for you to explore - I recommend you scan the table for topics you may be interested in - Then scan the 538 articles and narrow it down to 2-4 that interest you and your group-mates (groups of 3) --- class: center, middle # Finish [Problem Set 4](https://ismayc.github.io/soc301_s2017/problem-sets/#ps4) in groups ## Due Tuesday at the beginning of class *** ## [DC5: Barplots](https://campus.datacamp.com/courses/effective-data-storytelling-using-the-tidyverse/barplots?ex=1) due on Tuesday at 1 PM ## [PS5](https://ismayc.github.io/soc301_s2017/problem-sets/index.html#ps5) due on Tuesday at 1 PM *** # Quiz 2 over Chapters 3 and 4 of [ModernDive](http://moderndive.com) is on Thursday, March 2! --- layout: true .footer[[Week 04 - Tuesday](https://ismayc.github.io/soc301_s2017/2017/02/21/week-04---tuesday/)] --- name: week04t class: center, middle # Week 04 - Tuesday # February 21, 2017 --- class: center, middle # Turn in Problem Set 3 *** ### Also note that a short link for the course page is <br> http://bit.ly/soc-301 ### My office is now Marsh 213 ### Useful tip: Take notes on where R functions are introduced in the book and in DataCamp --- # Warmup Write the code necessary to produce the last plot using the `US_births_2014` data frame from the [Scatter-plots and Line-graphs](https://campus.datacamp.com/courses/effective-data-storytelling-using-the-tidyverse/scatter-plots-line-graphs?ex=4) chapter of DataCamp: 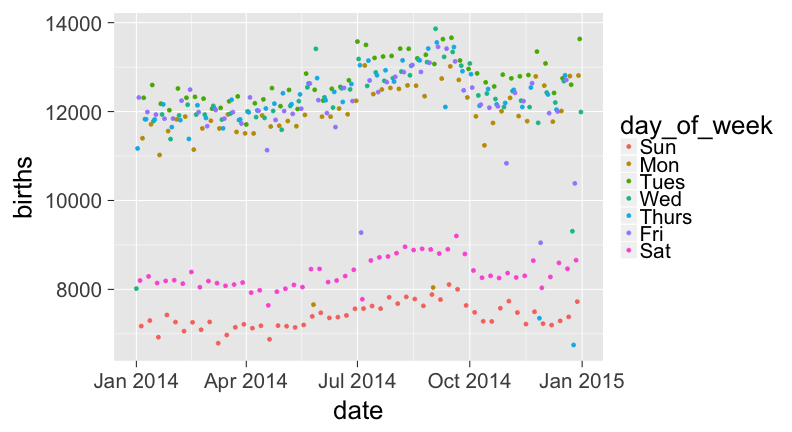<!-- --> --- # Discuss in groups of three - What stands out to you when you look at this plot? In other words, what stories could be told by observing this data? 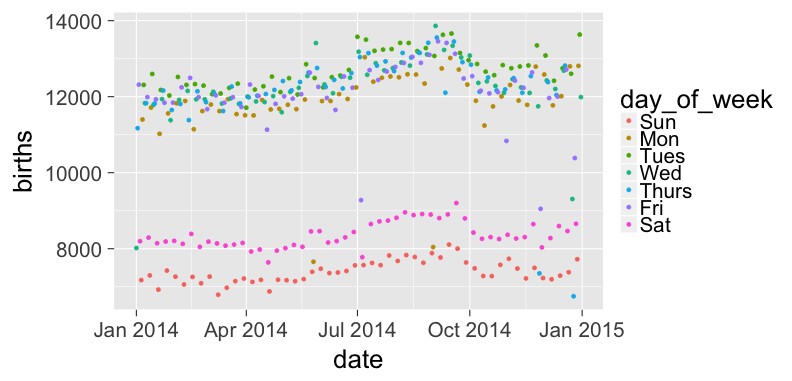<!-- --> --- class: center, middle # Histogram Basics --- ### Consider the following numerical data frame ```r library(dplyr) numeric_var <- c(10, 12, 14, 15, 18, 21) hist_example <- data_frame(numeric_var) hist_example ``` -- ``` # A tibble: 6 × 1 numeric_var <dbl> 1 10 2 12 3 14 4 15 5 18 6 21 ``` - Place the data into two bins of size 6 - Then produce a table showing how many data points fall in each bin --- ### Sketch a plot of this frequency table Bin | Count --------|------- (9-15] | 4 (15-21] | 2 -- ```r library(ggplot2) ggplot(data = hist_example, mapping = aes(x = numeric_var)) + geom_histogram(binwidth = 6, color = "black", fill = "white") ``` 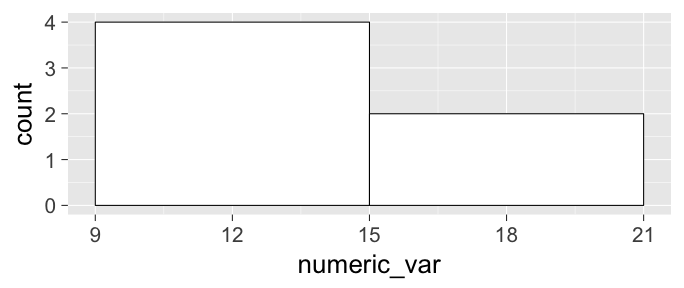<!-- --> --- class: center, middle # Recall that a statistical graphic is ## A <small>`mapping`</small> of <br> <small>`data`</small> variables ## to <br> <small>`aes()`</small>thetic attributes ## of <br> <small>`geom_`</small>etric objects. --- ## Practice in pairs - Write the code to produce a histogram of the humidity recordings in the [<small>`weather`</small> data frame](https://ismayc.github.io/Effective-Data-Storytelling-using-the-tidyverse/moderndive.html#weather) from the <small>`nycflights13`</small> package - with a bin width of 15, - white border color, and - fill with the [color of your choosing](http://www.stat.columbia.edu/~tzheng/files/Rcolor.pdf). - Practice in the DataCamp sandbox at <br> http://bit.ly/stats-sandbox -- - THINK ABOUT IT: What does this plot tell us? --- # Faceting - Oftentimes, we'd like to produce a small multiple of the same plot across the levels of a different variable. Suppose we'd like to look at the plot created on the last slide *across* the different <small>`origin`</small> airports. -- - Use the code given in Section 4.6 of [ModernDive](https://ismayc.github.io/moderndiver-book/4-viz.html#facets) and the plot you created on the last slide. - Write down your code on paper and test in the [sandbox](http://bit.ly/stats-sandbox) -- - THINK ABOUT IT: What does this faceted plot tell us? --- # Faceting - Write the code to produce the plot below 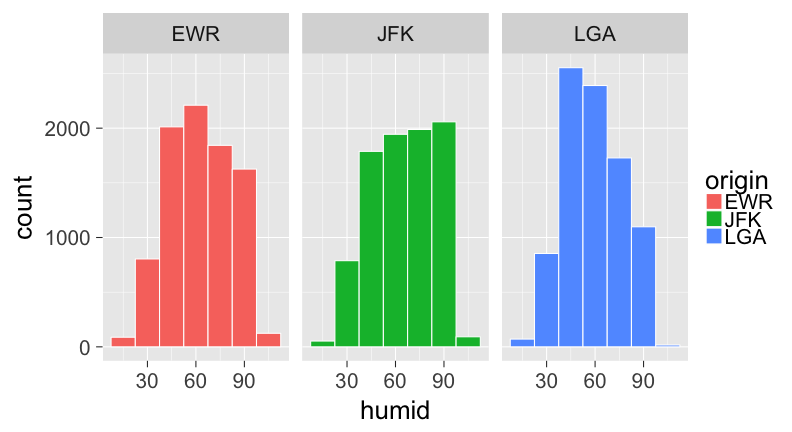<!-- --> --- # Another way to <small>`facet`</small> ```r library(nycflights13); library(ggplot2) ggplot(data = weather, mapping = aes(x = humid, fill = origin)) + geom_histogram(bins = 5, color = "white") + # NOTE bins here facet_grid(facets = origin ~ .) ``` 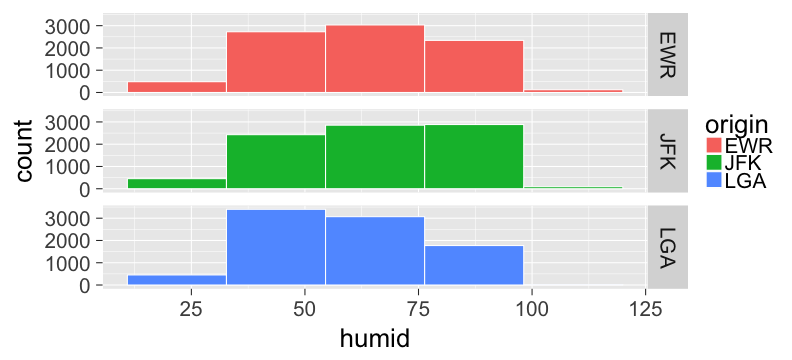<!-- --> --- # Explanatory and Response Variables ### Suppose we were interested in investigating the humidity throughout 2013 across the airports in NYC. - What would be the explanatory and response variables? - Note there is only one correct answer here. --- # Boxplots - Another way to view the relationship between a categorical explanatory variable and a numerical response variable is via a boxplot. Think about how we might write the code to produce the boxplot for humidity across airports. 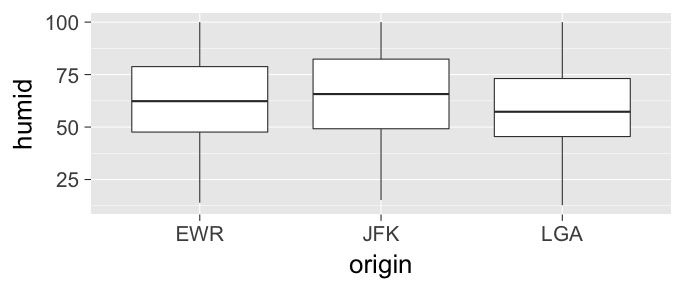<!-- --> --- class: center, middle # Recall that a statistical graphic is ## A <small>`mapping`</small> of <br> <small>`data`</small> variables ## to <br> <small>`aes()`</small>thetic attributes ## of <br> <small>`geom_`</small>etric objects. --- name: box ## Another boxplot example ### Time slept versus day of the week for a college professor <img src="figure/box.jpg" width="900" height="300" alt="Sleep Boxplot"> - What does this plot tell you/what story does it tell? --- class: center, middle # Work on [Problem Set 4](https://ismayc.github.io/soc301_s2017/problem-sets/#ps4) in groups ## Due on Thursday at the end of class *** ## [DC4: Histograms & Boxplots chapter](https://campus.datacamp.com/courses/effective-data-storytelling-using-the-tidyverse/histograms-boxplots?ex=1) due on Thursday at 1 PM *** # Quiz 2 over Chapters 3 and 4 of [ModernDive](http://moderndive.com) is on Thursday, March 2! --- # Minute paper ### Write the code necessary to produce this plot from earlier in class. - <small>Make sure to load all needed packages!</small> 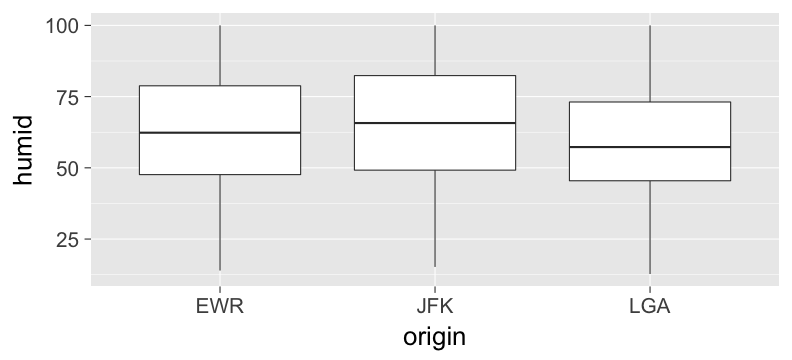<!-- --> --- layout: true .footer[[Week 03 - Thursday](https://ismayc.github.io/soc301_s2017/2017/02/16/week-03---thursday/)] --- name: week03th class: center, middle # Week 03 - Thursday # February 16, 2017 --- name: warmup # Warmup ### Is this data frame showing [average total fertility by country by year (since 1945)](http://ismayc.github.io/Effective-Data-Storytelling-using-the-tidyverse/total_fertility.html) a tidy data frame? <table> <thead> <tr> <th style="text-align:left;"> country </th> <th style="text-align:right;"> 1945 </th> <th style="text-align:right;"> 1946 </th> <th style="text-align:right;"> 1947 </th> <th style="text-align:right;"> 1948 </th> <th style="text-align:right;"> 1949 </th> <th style="text-align:right;"> 1950 </th> <th style="text-align:right;"> 1951 </th> <th style="text-align:right;"> 1952 </th> <th style="text-align:right;"> 1953 </th> <th style="text-align:right;"> 1954 </th> <th style="text-align:right;"> 1955 </th> <th style="text-align:right;"> 1956 </th> </tr> </thead> <tbody> <tr> <td style="text-align:left;"> Afghanistan </td> <td style="text-align:right;"> 7.54 </td> <td style="text-align:right;"> 7.56 </td> <td style="text-align:right;"> 7.59 </td> <td style="text-align:right;"> 7.62 </td> <td style="text-align:right;"> 7.64 </td> <td style="text-align:right;"> 7.67 </td> <td style="text-align:right;"> 7.67 </td> <td style="text-align:right;"> 7.67 </td> <td style="text-align:right;"> 7.67 </td> <td style="text-align:right;"> 7.67 </td> <td style="text-align:right;"> 7.67 </td> <td style="text-align:right;"> 7.67 </td> </tr> <tr> <td style="text-align:left;"> Albania </td> <td style="text-align:right;"> 4.93 </td> <td style="text-align:right;"> 5.10 </td> <td style="text-align:right;"> 5.28 </td> <td style="text-align:right;"> 5.45 </td> <td style="text-align:right;"> 5.63 </td> <td style="text-align:right;"> 5.80 </td> <td style="text-align:right;"> 5.90 </td> <td style="text-align:right;"> 6.07 </td> <td style="text-align:right;"> 6.21 </td> <td style="text-align:right;"> 6.31 </td> <td style="text-align:right;"> 6.37 </td> <td style="text-align:right;"> 6.40 </td> </tr> <tr> <td style="text-align:left;"> Algeria </td> <td style="text-align:right;"> 7.52 </td> <td style="text-align:right;"> 7.54 </td> <td style="text-align:right;"> 7.57 </td> <td style="text-align:right;"> 7.59 </td> <td style="text-align:right;"> 7.62 </td> <td style="text-align:right;"> 7.65 </td> <td style="text-align:right;"> 7.65 </td> <td style="text-align:right;"> 7.65 </td> <td style="text-align:right;"> 7.65 </td> <td style="text-align:right;"> 7.65 </td> <td style="text-align:right;"> 7.65 </td> <td style="text-align:right;"> 7.65 </td> </tr> </tbody> </table> - <small>If so, what is the observational unit and what are the variables?</small> - <small>If not, what would three rows of a tidy data set look like with variable names at the top?</small> Source: Original data from [Gapminder](https://www.gapminder.org/data/), [Google Sheet with explanations](https://docs.google.com/spreadsheets/d/1oq3r8W7ajenKFgoAYoOf2MXeTWWNPpudR-Fo5m2-o30/pub?gid=0) <small>[Why is tidy data important?](#whytidy) slide<small> --- class: middle, center ## Reviewing <small>`inner_join`</small> ### [DataCamp problem](https://campus.datacamp.com/courses/effective-data-storytelling-using-the-tidyverse/tidy-data?ex=4) --- class: center, middle # What is a statistical graphic? --- # Grammar of Graphics ### Wilkinson (2005) laid out the proposed "Grammar of Graphics" <img src="figure/graphics.jpg" style="display: block; margin: auto;" /> --- # Grammar of Graphics in R ### Wickham implemented the grammar in R in the `ggplot2` package <img src="figure/ggplot2.jpg" style="display: block; margin: auto;" /> --- class: center, middle # But...what is a statistical graphic? -- ## A <small>`mapping`</small> of <br> <small>`data`</small> variables -- ## to <br> <small>`aes()`</small>thetic attributes -- ## of <br> <small>`geom_`</small>etric objects. --- class: center, middle # Back to basics --- ### Consider the following data in tidy format: <table> <thead> <tr> <th style="text-align:right;"> A </th> <th style="text-align:right;"> B </th> <th style="text-align:right;"> C </th> <th style="text-align:left;"> D </th> </tr> </thead> <tbody> <tr> <td style="text-align:right;"> 1 </td> <td style="text-align:right;"> 1 </td> <td style="text-align:right;"> 3 </td> <td style="text-align:left;"> a </td> </tr> <tr> <td style="text-align:right;"> 2 </td> <td style="text-align:right;"> 2 </td> <td style="text-align:right;"> 2 </td> <td style="text-align:left;"> a </td> </tr> <tr> <td style="text-align:right;"> 3 </td> <td style="text-align:right;"> 3 </td> <td style="text-align:right;"> 1 </td> <td style="text-align:left;"> b </td> </tr> <tr> <td style="text-align:right;"> 4 </td> <td style="text-align:right;"> 4 </td> <td style="text-align:right;"> 2 </td> <td style="text-align:left;"> b </td> </tr> </tbody> </table> - Sketch the graphics below on paper, where the `x`-axis is variable `A` and the `y`-axis is variable `B` 1. <small>A scatter plot</small> 1. <small>A scatter plot where the `color` of the points corresponds to `D`</small> 1. <small>A scatter plot where the `size` of the points corresponds to `C`</small> 1. <small>A line graph</small> 1. <small>A line graph where the `color` of the line corresponds to `D`</small> --- ## Reproducing the plots in <small>`ggplot2`</small> ### 1. A scatterplot ```r library(ggplot2) ggplot(data = simple_ex, mapping = aes(x = A, y = B)) + geom_point() ``` -- 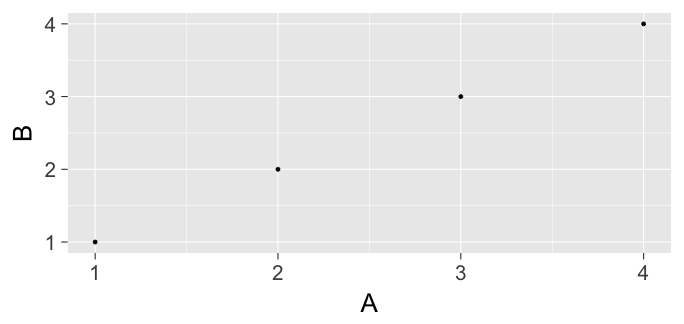<!-- --> --- ## Reproducing the plots in <small>`ggplot2`</small> ### 2. A scatter plot where the `color` of the points corresponds to `D` ```r library(ggplot2) ggplot(data = simple_ex, mapping = aes(x = A, y = B)) + geom_point(aes(color = D)) ``` -- 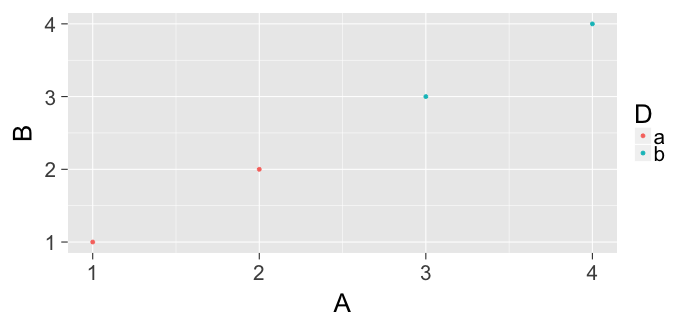<!-- --> --- ## Reproducing the plots in <small>`ggplot2`</small> ### 3. A scatter plot where the `size` of the points corresponds to `C` ```r library(ggplot2) ggplot(data = simple_ex, mapping = aes(x = A, y = B, size = C)) + geom_point() ``` -- 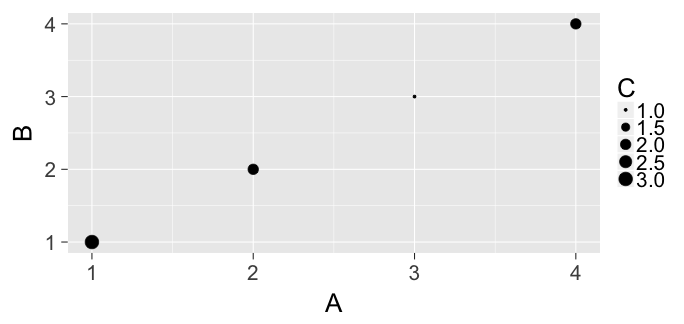<!-- --> --- ## Reproducing the plots in <small>`ggplot2`</small> ### 4. A line graph ```r library(ggplot2) ggplot(data = simple_ex, mapping = aes(x = A, y = B)) + geom_line() ``` -- <!-- --> --- ## Reproducing the plots in <small>`ggplot2`</small> ### 5. A line graph where the `color` of the line corresponds to `D` ```r library(ggplot2) ggplot(data = simple_ex, mapping = aes(x = A, y = B, color = D)) + geom_line() ``` -- 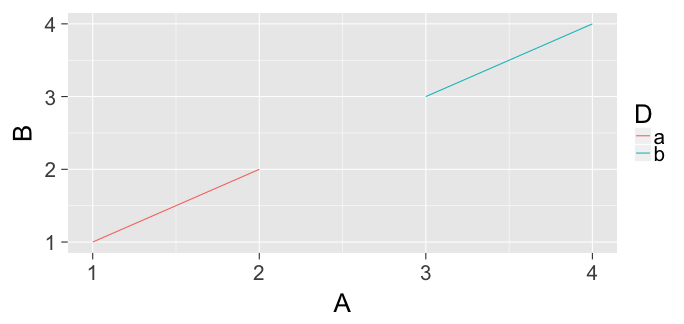<!-- --> --- name: whytidy ## Why is tidy data important? - Think about trying to plot total fertility across years in the simplest way possible with the data on [Warmup slide](#warmup). -- - It would be much easier if the data looked like what follows instead so we could put <small>`year`</small> on the <small>`x`</small>-axis and <small>`fert`</small> on the <small>`y`</small>-axis. <table> <thead> <tr> <th style="text-align:left;"> country </th> <th style="text-align:right;"> year </th> <th style="text-align:right;"> fert </th> </tr> </thead> <tbody> <tr> <td style="text-align:left;"> Chad </td> <td style="text-align:right;"> 1981 </td> <td style="text-align:right;"> 6.99 </td> </tr> <tr> <td style="text-align:left;"> Haiti </td> <td style="text-align:right;"> 1989 </td> <td style="text-align:right;"> 5.55 </td> </tr> <tr> <td style="text-align:left;"> Haiti </td> <td style="text-align:right;"> 1980 </td> <td style="text-align:right;"> 6.06 </td> </tr> <tr> <td style="text-align:left;"> Mexico </td> <td style="text-align:right;"> 1985 </td> <td style="text-align:right;"> 3.90 </td> </tr> <tr> <td style="text-align:left;"> Vietnam </td> <td style="text-align:right;"> 1953 </td> <td style="text-align:right;"> 5.52 </td> </tr> <tr> <td style="text-align:left;"> Vietnam </td> <td style="text-align:right;"> 1965 </td> <td style="text-align:right;"> 6.48 </td> </tr> </tbody> </table> --- ## Making a scatterplot ```r library(ggplot2) ggplot(data = total_fert_tidy, mapping = aes(x = year, y = fert)) + geom_point() ``` 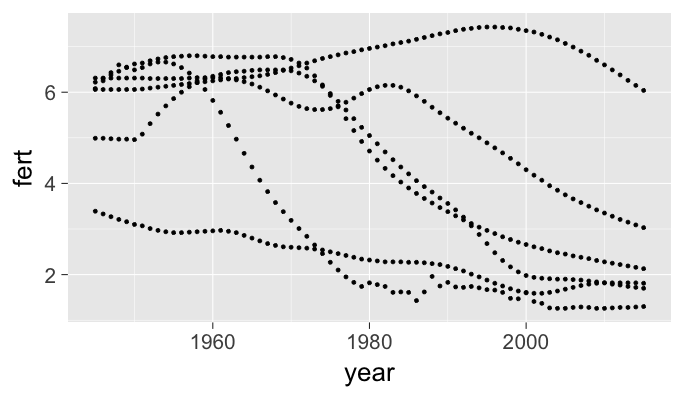<!-- --> --- ## Discussing the scatterplot 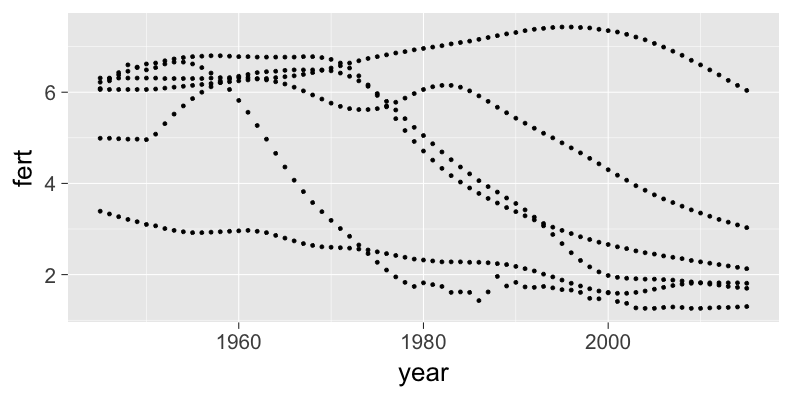<!-- --> - What stands out to you as you look at this plot? - What other information would be useful here? --- ## Identifying the countries <table> <thead> <tr> <th style="text-align:left;"> country </th> </tr> </thead> <tbody> <tr> <td style="text-align:left;"> Chad </td> </tr> <tr> <td style="text-align:left;"> Georgia </td> </tr> <tr> <td style="text-align:left;"> Haiti </td> </tr> <tr> <td style="text-align:left;"> Mexico </td> </tr> <tr> <td style="text-align:left;"> Singapore </td> </tr> <tr> <td style="text-align:left;"> Vietnam </td> </tr> </tbody> </table> -- - How could we identify the countries on the plot? -- - This is another reason why *tidy data* is so valuable. <br> Check this out! --- ## Color by country ```r library(ggplot2) ggplot(data = total_fert_tidy, mapping = aes(x = year, y = fert, color = country)) + geom_point() ``` 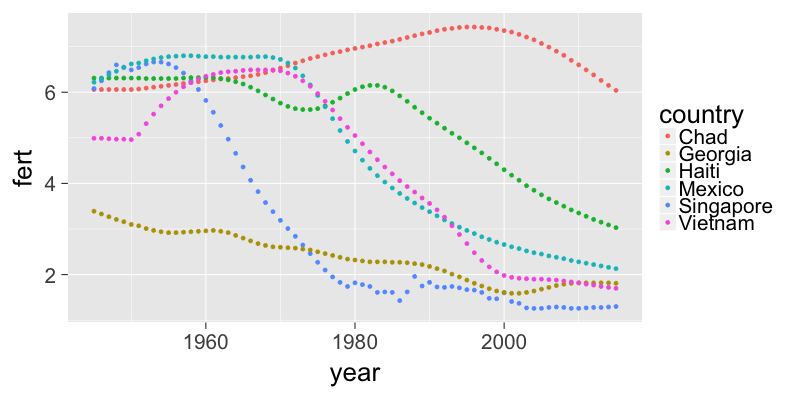<!-- --> --- ## Shape by country ```r library(ggplot2) ggplot(data = total_fert_tidy, mapping = aes(x = year, y = fert, shape = country)) + geom_point() ``` 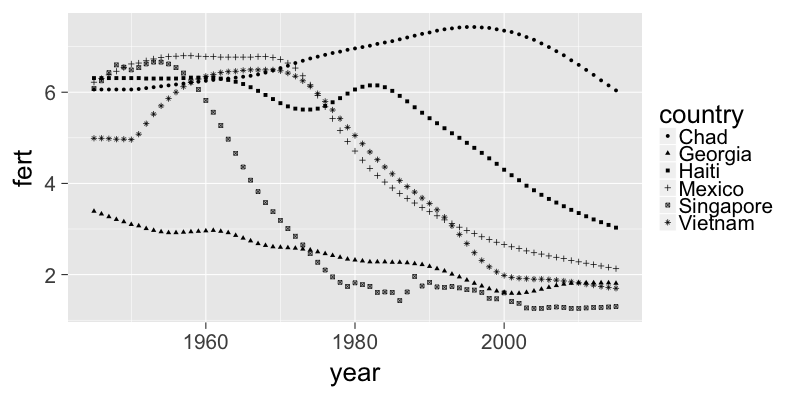<!-- --> --- ## Color and shape by country ```r library(ggplot2) ggplot(data = total_fert_tidy, mapping = aes(x = year, y = fert, color = country, shape = country)) + geom_point() ``` 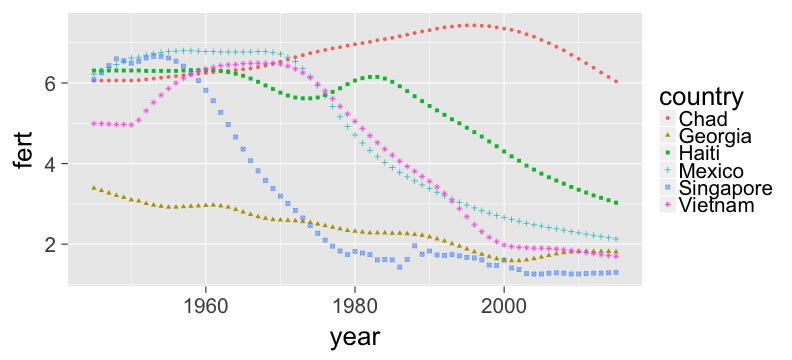<!-- --> --- class: center, middle # Shifting back to the data from [Hans Rosling's video](https://www.youtube.com/watch?v=jbkSRLYSojo) we watched yesterday --- ## The <small>`gapminder`</small> package and data frame ```r library(gapminder) data(gapminder) glimpse(gapminder) ``` ``` Observations: 1,704 Variables: 6 $ country <fctr> Afghanistan, Afghanistan, Afghanistan, Afghanistan,... $ continent <fctr> Asia, Asia, Asia, Asia, Asia, Asia, Asia, Asia, Asi... $ year <int> 1952, 1957, 1962, 1967, 1972, 1977, 1982, 1987, 1992... $ lifeExp <dbl> 28.801, 30.332, 31.997, 34.020, 36.088, 38.438, 39.8... $ pop <int> 8425333, 9240934, 10267083, 11537966, 13079460, 1488... $ gdpPercap <dbl> 779.4453, 820.8530, 853.1007, 836.1971, 739.9811, 78... ``` *** <table> <thead> <tr> <th style="text-align:left;"> country </th> <th style="text-align:left;"> continent </th> <th style="text-align:right;"> year </th> <th style="text-align:right;"> lifeExp </th> <th style="text-align:right;"> pop </th> <th style="text-align:right;"> gdpPercap </th> </tr> </thead> <tbody> <tr> <td style="text-align:left;"> Afghanistan </td> <td style="text-align:left;"> Asia </td> <td style="text-align:right;"> 1952 </td> <td style="text-align:right;"> 28.801 </td> <td style="text-align:right;"> 8425333 </td> <td style="text-align:right;"> 779.4453 </td> </tr> <tr> <td style="text-align:left;"> Afghanistan </td> <td style="text-align:left;"> Asia </td> <td style="text-align:right;"> 1957 </td> <td style="text-align:right;"> 30.332 </td> <td style="text-align:right;"> 9240934 </td> <td style="text-align:right;"> 820.8530 </td> </tr> <tr> <td style="text-align:left;"> Afghanistan </td> <td style="text-align:left;"> Asia </td> <td style="text-align:right;"> 1962 </td> <td style="text-align:right;"> 31.997 </td> <td style="text-align:right;"> 10267083 </td> <td style="text-align:right;"> 853.1007 </td> </tr> <tr> <td style="text-align:left;"> Afghanistan </td> <td style="text-align:left;"> Asia </td> <td style="text-align:right;"> 1967 </td> <td style="text-align:right;"> 34.020 </td> <td style="text-align:right;"> 11537966 </td> <td style="text-align:right;"> 836.1971 </td> </tr> </tbody> </table> --- ## Looking only at 2007 - This code will be covered more in [Chapter 5 of ModernDive](https://ismayc.github.io/moderndiver-book/5-manip.html) ```r library(dplyr) gap2007 <- gapminder %>% filter(year == 2007) head(gap2007, n = 10) ``` ``` # A tibble: 10 × 6 country continent year lifeExp pop gdpPercap <fctr> <fctr> <int> <dbl> <int> <dbl> 1 Afghanistan Asia 2007 43.828 31889923 974.5803 2 Albania Europe 2007 76.423 3600523 5937.0295 3 Algeria Africa 2007 72.301 33333216 6223.3675 4 Angola Africa 2007 42.731 12420476 4797.2313 5 Argentina Americas 2007 75.320 40301927 12779.3796 6 Australia Oceania 2007 81.235 20434176 34435.3674 7 Austria Europe 2007 79.829 8199783 36126.4927 8 Bahrain Asia 2007 75.635 708573 29796.0483 9 Bangladesh Asia 2007 64.062 150448339 1391.2538 10 Belgium Europe 2007 79.441 10392226 33692.6051 ``` --- ## What code is needed to produce this plot? - Write the code on paper and then discuss in groups of 3. 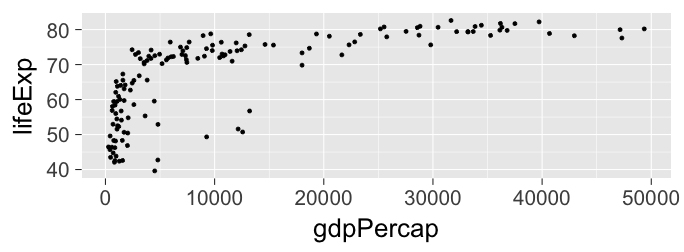<!-- --> -- - There are 199 countries included here. What would be a different/better way we could highlight points than by country that would be of interest here? --- ## Coloring by `continent` - Write the code on paper and then discuss in groups of 3. 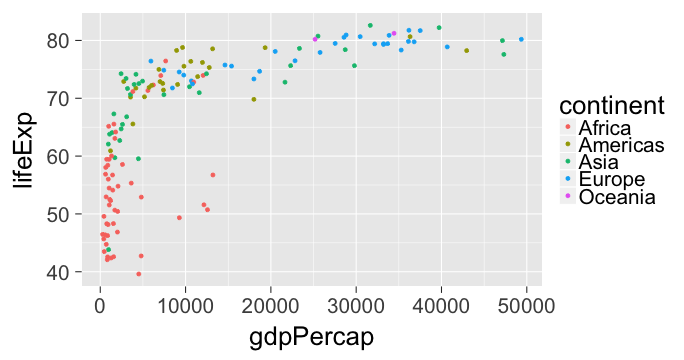<!-- --> -- - What information is added by the coloring here? --- class: center, middle # Work on [Problem Set 3](https://ismayc.github.io/soc301_s2017/problem-sets/#ps3) in groups ## Due Tuesday at the beginning of class *** ## [DC3: Scatter-plots & Line-graphs chapter](https://campus.datacamp.com/courses/effective-data-storytelling-using-the-tidyverse/scatter-plots-line-graphs?ex=1) due on Tuesday at 1 PM --- ## DataCamp notes - Leaderboard not available currently - Complete your DataCamp profiles! - Click on your user ID in the top right of the screen when you first open DataCamp. - Click on My Profile. - Select Edit Account Settings. - Input at the very least your first and last name. More helpful if you include a picture. --- ## Minute Paper - Put your name on it! What `ggplot2` code is needed to produce this plot? The `simple_ex` data frame is printed for support. <table> <thead> <tr> <th style="text-align:right;"> A </th> <th style="text-align:right;"> B </th> <th style="text-align:right;"> C </th> <th style="text-align:left;"> D </th> </tr> </thead> <tbody> <tr> <td style="text-align:right;"> 1 </td> <td style="text-align:right;"> 1 </td> <td style="text-align:right;"> 3 </td> <td style="text-align:left;"> a </td> </tr> <tr> <td style="text-align:right;"> 2 </td> <td style="text-align:right;"> 2 </td> <td style="text-align:right;"> 2 </td> <td style="text-align:left;"> a </td> </tr> <tr> <td style="text-align:right;"> 3 </td> <td style="text-align:right;"> 3 </td> <td style="text-align:right;"> 1 </td> <td style="text-align:left;"> b </td> </tr> <tr> <td style="text-align:right;"> 4 </td> <td style="text-align:right;"> 4 </td> <td style="text-align:right;"> 2 </td> <td style="text-align:left;"> b </td> </tr> </tbody> </table> <img src="slide_deck_files/figure-html/unnamed-chunk-152-1.png" style="display: block; margin: auto;" /> --- layout: true .footer[[Week 03 - Tuesday](https://ismayc.github.io/soc301_s2017/2017/02/14/week-03---tuesday/)] --- name: week03t class: center, middle # Week 03 - Tuesday # February 14, 2017 --- # Warmup ### Take out a (half) sheet of paper - What are the three properties of a tidy data set? --- class:center, middle # Happy Valentine's Day! [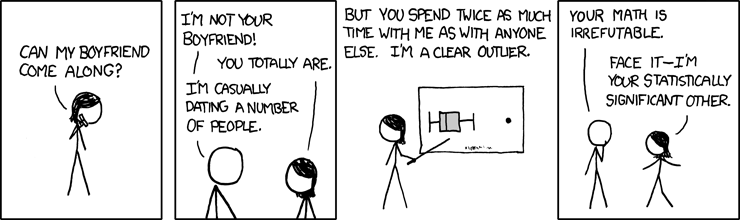](https://xkcd.com/539/) --- # Questions/concerns about class - What is the longest assignment we will be given? -- - Eventually are we going to write our own code to solve problems? -- - What are good ways to practice programming without DataCamp? -- - How do you format your exams? --- # Questions/concerns about class - If I get questions wrong many times before I get it right on DataCamp, will this hurt my grade? -- - I worry I don't actually know what I'm doing. -- - More details about the project. -- - Does `?`/`help()` only work with explaining functions? --- ## Studying well and efficiently - Image from [_Teach Students How to Learn_](https://www.amazon.com/Teach-Students-How-Learn-Metacognition/dp/162036316X) ### <http://ismayc.github.io/soc301_s2017/img/studentlearn.jpg> --- # Some clarifications - Telling me early that you will be gone on a day in the future is NOT an excused absence - You can miss class three times for whatever you wish - You need institutional excuse to miss more than three -- - Just doing the homework is not enough to build sufficient understanding - You need to go back over DataCamp, the Learning Checks, and the book on your own to check for understanding --- # Some clarifications - I'm not trying to scare you, but quizzes and exams will be HARD and you will struggle if you don't follow what I am telling you. - If you follow the effective learning strategies I have shown you and devote yourself to practice, you'll do great AND you'll retain the material. - You'll find that you'll actually be studying LESS, since you won't have to re-learn all the material and cram for exams/quizzes. --- class: center, middle # Review [Problem Set 2 Discussion](https://ismayc.github.io/soc301_s2017/problem-sets/ps-key/index.html#ps2-key) --- class: center, middle # Plickers for Review --- # Important R functions/operators from [Chapter 3](https://ismayc.github.io/moderndiver-book/3-tidy.html) - `library()` (VERY IMPORTANT) -- - `<-` -- - `glimpse()` -- - `?` -- - `inner_join()` --- # What is meant by "joining data frames" and why is it useful? -- <img src="https://ismayc.github.io/moderndiver-book/images/join-inner.png" style="display: block; margin: auto;" /> --- # Do joins change the observational unit? -- ### View [`police_locals`](https://ismayc.github.io/Effective-Data-Storytelling-using-the-tidyverse/police_locals.html) ### View [`ideology`](https://ismayc.github.io/Effective-Data-Storytelling-using-the-tidyverse/ideology.html) - Join based on `"city"` --- # Do joins change the observational unit? ```r library(fivethirtyeight); library(dplyr); library(readr) data(police_locals) ideology <- read_csv("http://bit.ly/ideology-csv") police_join <- inner_join(x = police_locals, y = ideology, by = "city") ``` ### View [`police_join`](https://ismayc.github.io/Effective-Data-Storytelling-using-the-tidyverse/police_join.html) --- class: center, middle ## THINK ABOUT IT ### How does linking these two data sets together help in analyzing the relationship between `state_ideology` and percentage of police officers residing in the cities they serve by race? --- # Shifting to [Data Viz](https://ismayc.github.io/moderndiver-book/4-viz.html) <div class="videoWrapper">
</div> --- ## Grade Viewer is up ### <http://ismay.shinyapps.io/soc301_grades> - Also available on the [course page](http://ismayc.github.io/soc301_s2017) under Resources --- class: center, middle # Work on [Problem Set 3](https://ismayc.github.io/soc301_s2017/problem-sets/#ps3) in groups ## Due on Thursday at the end of class *** ## [DC2: Tidy Data chapter](https://campus.datacamp.com/courses/effective-data-storytelling-using-the-tidyverse/tidy-data?ex=1) due on Thursday at 1 PM --- # Minute Paper ### (Half sheet of paper is fine. Share with your neighbor. Make sure to put your name on it!) - What are the three characteristics of tidy data? - How did Hans Rosling use data visualization to help tell the story he wanted to tell in the video we watched? --- layout: true .footer[[Week 02 - Thursday](https://ismayc.github.io/soc301_s2017/2017/02/09/week-02---thursday/)] --- name: week02th class: center, middle # Week 02 - Thursday # February 9, 2017 --- class: center, middle ## Learning Quote of the Day <img src="figure/hadley.png" width="1571" /> --- class: center, middle # Take Notes! --- ## Tidy Data <img src="figure/tidy-1.png" alt="Drawing" style="width: 750px;"/> --- ## Is this tidy? ``` # A tibble: 96 × 13 country `1952` `1957` `1962` `1967` `1972` `1977` `1982` `1987` <chr> <int> <int> <int> <int> <int> <int> <int> <int> 1 Albania -9 -9 -9 -9 -9 -9 -9 -9 2 Argentina -9 -1 -1 -9 -9 -9 -8 8 3 Armenia -9 -7 -7 -7 -7 -7 -7 -7 4 Australia 10 10 10 10 10 10 10 10 5 Austria 10 10 10 10 10 10 10 10 6 Azerbaijan -9 -7 -7 -7 -7 -7 -7 -7 7 Belarus -9 -7 -7 -7 -7 -7 -7 -7 8 Belgium 10 10 10 10 10 10 10 10 9 Bhutan -10 -10 -10 -10 -10 -10 -10 -10 10 Bolivia -4 -3 -3 -4 -7 -7 8 9 # ... with 86 more rows, and 4 more variables: `1992` <int>, `1997` <int>, # `2002` <int>, `2007` <int> ``` --- ## Beginning steps ### Frequently the first thing you should do when given a dataset is to - check that the data is *tidy*, - identify the observational unit, - specify the variables, and - give the types of variables you are presented with. ### This will help you with - choosing the appropriate plot, - summarizing the data, and - understanding which inferences can be applied. --- ## What is Tidy Data? <img src="http://garrettgman.github.io/images/tidy-1.png" alt="Drawing" style="width: 600px;"/> 1. Each variable forms a column. 2. Each observation forms a row. 3. Each type of observational unit forms a table. ### The third point means we don't mix apples and oranges, keep apples together with apples. --- ## What is Tidy Data? 1. Each observation forms a row. In other words, each row corresponds to a single *observational unit* 1. Each variable forms a column: + Some of the variables may be used to identify the *observational units*. For organizational purposes, it's generally better to put these in the left-hand columns + Some of the variables may be *observed values* associated with each observational unit 1. Each type of observational unit forms a table. --- ## What is Tidy Data? ### This is just a spreadsheet! ### But we are very strict about the previous three rules. --- ## [nycflights13 Package](http://github.com/hadley/nycflights13) - Loaded via `library(nycflights13)` - Contains information about all flights that departed from NYC in 2013: 336,776 flights in total. --- ## [nycflights13 Package](http://github.com/hadley/nycflights13) - To help understand what causes delays, it also includes a number of other useful datasets: <small> * `weather`: hourly meterological data for each airport * `planes`: construction information about each plane * `airports`: airport names and locations * `airlines`: translation between two letter carrier codes and names ### All of these data frames are available for viewing [here](https://ismayc.github.io/Effective-Data-Storytelling-using-the-tidyverse/moderndive.html). --- class: center, middle # Work on [Problem Set 2](https://ismayc.github.io/soc301_s2017/problem-sets/) --- ## Minute Paper ### (Half sheet of paper is fine. Share with your neighbor.) - What are the three characteristics of tidy data? - Draw the DATA diagram that was presented on the board in all previous classes. --- name: week02t layout: true .footer[[Week 02 - Tuesday](https://ismayc.github.io/soc301_s2017/2017/02/07/week-02---tuesday/)] --- class: center, middle # Week 02 - Tuesday # February 7, 2017 --- ## Sample quiz question <center> <img src="figure/jeopardy.png" width="810" /> </center> <center> <img src="figure/jq.png" width="821" /> </center> --- ## Asking for help - Recommended: Post your problem and drag a screenshot image of what you have tried to the #general channel on Slack. - If you know the answer to a question there, please attempt to answer it. It will help you learn! - Send me a private message on Slack (@chester) - Discuss your problems with the tutors in person - Last resort, send the tutors a private message on Slack --- ## You Should Learn to Program <div class="videoWrapper">
</div> --- ## Easy classes are worthless classes - You won't retain any of the material covered -- - You or someone else pays good money for you to get a good education here -- - I want to do everything in my power to help you learn this material as I see it as THE MOST IMPORTANT class you can take as an undergraduate. -- - You have many misconceptions about how you learn best. That's not your fault, but habits must be broken. -- - Can we build muscles sitting on the couch? --- ## Why are you learning R? - ~~Because I enjoy partaking in [Schadenfreude](https://en.wikipedia.org/wiki/Schadenfreude)~~ <center> <img src="figure/google_scholar.png" width="567" /> </center> --- ## Why are you learning R? - R is free, R improves, R has existed for 40+ years - You can show what you have learned to a potential employer easily - I want to prepare you for the real world as best as I can and make you more employable than your competitors --- ## Why are you learning R? - R will save you TONS of time in the long run. Long term thinking is key. - You can easily tweak your code if you need to do another analysis - Remembering what drop-down menu some thing is in two years from now in a different program will be hard --- class: center, middle # Reviewing R basics <br> (on chalkboard) --- ## Key R functions/operators going forward ### Operators - `<-` - `[]` - `[ , ]` - `$` (VERY IMPORTANT) - `:` - `<`, `>`, `<=`, `>=` - `==`, `!=` - `?` --- ## Key R functions/operators going forward ### Functions - `names()` - `c()` - `sum()` - `mean()` - `factor()` - `factor( , order = TRUE, levels = c( , , )`) - ~~`str()`~~ `tibble::glimpse()` --- ## How should you prepare for the quiz? <div class="videoWrapper">
</div> --- ## How should you prepare for the quiz? - [Advice from former students](http://ismayc.github.io/soc301_s2017/advice_from_former/index.html) - Practice! --- ## Get into groups of 3 or 4 - Pass your multiple choice questions to someone else in your group - Take the "quiz" with no notes - Discuss your answers with your group - Take another "quiz" - Rinse and repeat --- class: middle, center # Check the [webpage](https://ismayc.github.io/soc301_s2017/)! --- class: middle, center # I want your feedback! --- ## Minute Paper ### (Half sheet of paper is fine. Share with your neighbor.) - What are the big take-aways for you from class today? - What questions do you have about the course? About the material you've learned so far? --- layout: true .footer[[Week 01 - Thursday](https://ismayc.github.io/soc301_s2017/2017/02/02/week-01---thursday/)] --- name: week01th class: center, middle # Week 01 - Thursday # February 2, 2017 --- ## DataCamp Assignments - <https://www.datacamp.com/groups/soc-301-spring-2017/assignments> --- ## Videos on Resources page - <https://ismayc.github.io/soc301_s2017/resources/> --- ## Studying well and efficiently - Image from [_Teach Students How to Learn_](https://www.amazon.com/Teach-Students-How-Learn-Metacognition/dp/162036316X) ### <http://ismayc.github.io/soc301_s2017/img/studentlearn.jpg> --- ## Tuesday plans - Review the content in the Introduction to R DataCamp course - Finish writing multiple choice questions - Study for Quiz 1 over Introduction to R DataCamp course on Thursday, February 9 in class - Bring your laptops on Tuesday, and every class period! - This is the time to make a good impression and start off strong! --- ## Minute papers - Place your name on a sheet of paper. Answer the following: 1. When you think of statistics what do you think of? 1. What statistical concepts would you like to learn about? 2. What concerns you most about this course? 3. Do you believe you'll be able to devote the time needed to succeed in this course? Explain. - A four credit-hour course requires between 9 and 12 hours of time outside of it each week. --- layout: true .footer[[Week 01 - Tuesday](https://ismayc.github.io/soc301_s2017/2017/01/31/week-01---tuesday/)] --- name: week01t class: center, middle # Week 01 - Tuesday # January 31, 2017 --- ## For interviews in groups of three 1. Name 3. How do you think this course applies to your major? 5. What are your goals for this course? 3. What is your class standing (Sophomore, Junior, etc.)? 3. What is your major? 4. What is your hometown? 6. What is the farthest from Pacific you were over break (in miles)? 7. What is one interesting fact about you? 8. How old is Chester (in years)? --- class: center, middle <img src="figure/notebook.jpg" alt="notebook" style="width:450px;"> --- ## Slides link ### <http://ismayc.github.io/soc301_s2017/slides/slide_deck.html> - Easier to get from <http://ismayc.github.io/soc301_s2017/> --- ## Course Textbook - <http://moderndive.com> --- ## DataCamp Assignments - <https://www.datacamp.com/groups/soc-301-spring-2017/assignments> --- ## Special accommodations ### Please come talk to me after class if you require special accommodations. --- ## To do for next time - Carefully read over the syllabus in entirety - Make note of important dates on the schedule - Read Chapters 1 and 2 of [ModernDive](http://moderndive.com) - Begin working on Introduction to R DataCamp course - Needs to be completed by 1 PM Pacific on Tuesday, February 7 - If you have spent more than 30 minutes on a problem, move on to another one OR reach out for help. --- ## Asking for help - Recommended: Post your problem and drag a screenshot image of what you have tried to the #general channel on Slack. - If you know the answer to a question there, please attempt to answer it. It will help you learn! - Send me a private message on Slack (@chester) - Discuss your problems with the tutors in person - Last resort, send the tutors a private message on Slack --- ## Thursday plans - Bring your laptops on Thursday, and every class period! - Work on and finish Introduction to R DataCamp course - Make sure to have started and be at the point where you have specific questions Dinisa and I can address - This is the time to make a good impression and start off strong!